Predictive Maintenance in Manufacturing: A Complete Guide
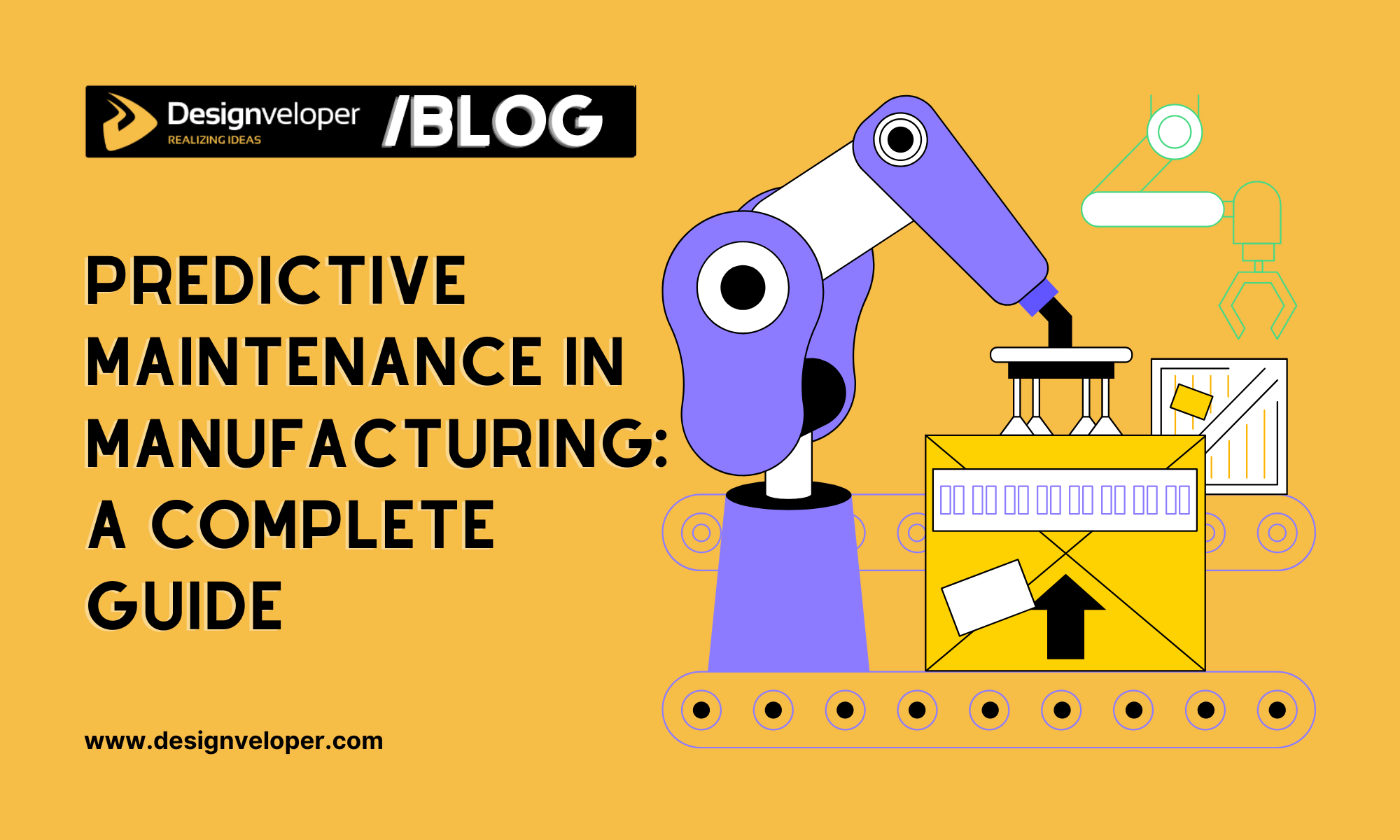
Maintaining machinery is crucial for any manufacturing plant if they don’t want delays in production and unhappy customers. Various maintenance practices were introduced, each with pros and cons. Among them, predictive maintenance in manufacturing proves most effective and changes the way companies monitor their equipment. So what is it, and how does it work, exactly? Let’s learn about this interesting topic from A to Z.
Understanding Predictive Maintenance (PdM) in Manufacturing
In the first section, we’ll dive into the basics of predictive maintenance in manufacturing, from its definition to its common types and technology trends.
What is Predictive Maintenance in Manufacturing?
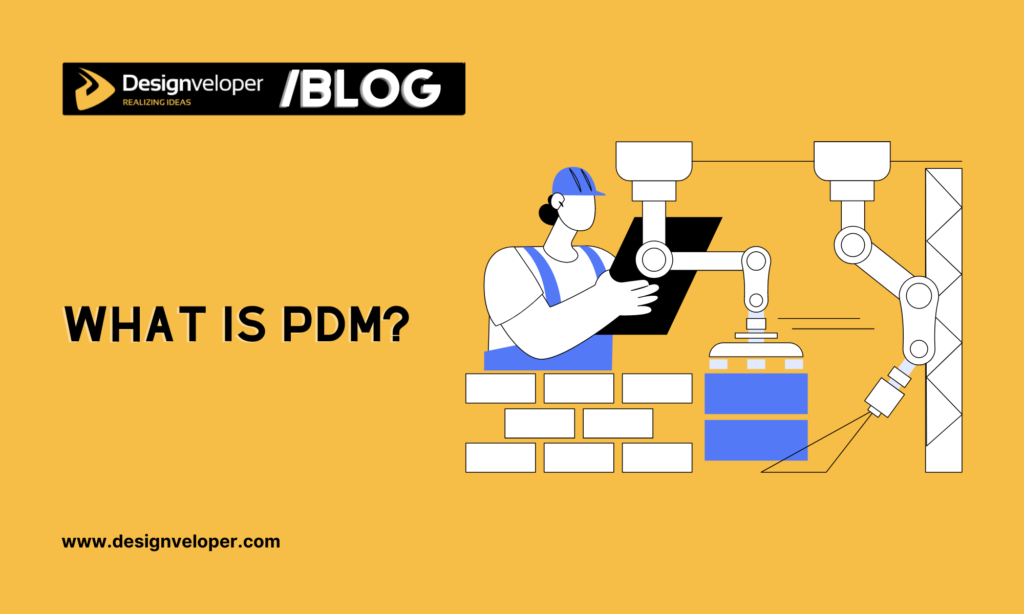
Predictive maintenance, or PdM, is a proactive method that helps manufacturers predict when a machine will fail so that you can repair it before it breaks down. The approach collects real-time data from sensor devices and monitoring systems for analytics to better understand the equipment’s performance and overall health. This also allows manufacturers to identify early signs of problems like equipment failure or malfunction.
The concept of PdM dates back to the 1940s when C.H. Waddington developed a scheduled maintenance program. This program used rule-based manual data collection and vibration analysis to periodically check fighter planes.
Despite its success, predictive maintenance wasn’t quite popular and only confined to the heavy machinery industry until the 1990s. Especially, the coming of computers, sensors, and the Internet of Things (IoT) had advanced PdM, hence making it a common approach in many industries, including manufacturing. This has increased the global value of predictive maintenance by 35.1% annually from 2024 to 2029.
How Does PdM Work?
So, how can this maintenance practice predict the potential issues of your machinery? Before initiating a predictive maintenance program, you need to set conditional baselines. They function as control values used to compare with the operating conditions of an asset or equipment. In other words, they help your manufacturing plant identify whether the machine is working properly or behaving within normal conditions.
Then, sensors are installed to collect real-time data about the equipment’s performance. This data might involve vibration, temperature, pressure, oil amounts, power consumption, noise levels, etc. By comparing the collected data with conditional baselines, PdM can easily spot any deviations that indicate any potential issues.
For instance, if a motor vibrates more than normal, the PdM system can catch this abnormal pattern through the data transmitted from sensors. It then automatically alerts your team to inspect and fix the issue before the motor breaks down.
Types of PdM: Rule-Based vs. Machine Learning-Based
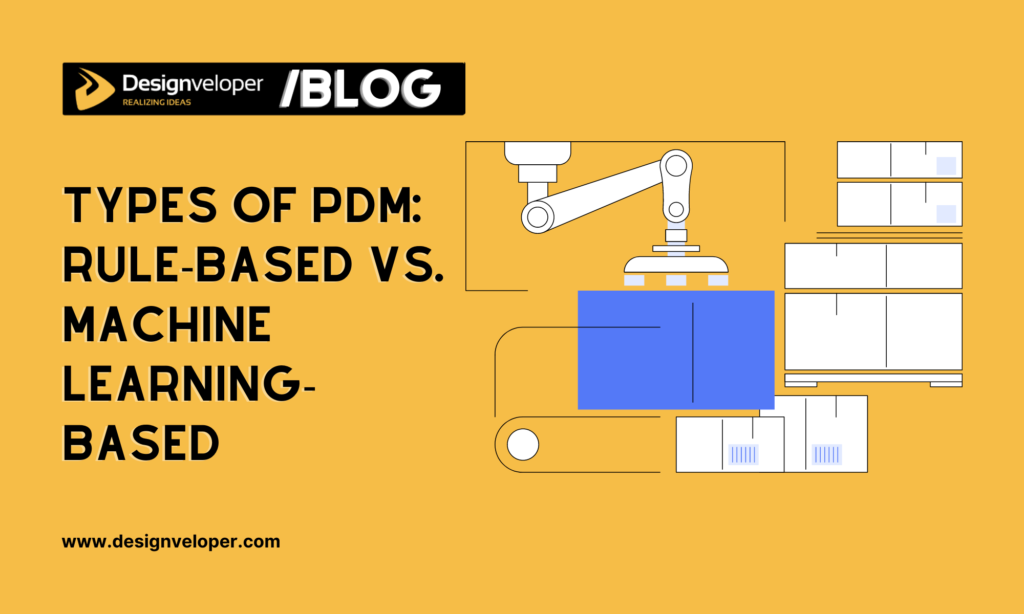
Predictive maintenance in manufacturing comes into categories based on different factors. Here, we’ll classify PdM approaches according to their technologies:
Rule-Based
This practice depends on condition monitoring sensors and specific rules established in advance. These sensors constantly collect data about machinery and trigger alerts based on these predefined conditions. For instance, if a machine’s temperature goes beyond a particular limit, the system will send a warning to the maintenance team.
These conditions are often based on historical data and experiences. However, this might make the system miss some issues as it only reacts to pre-programmed rules.
Therefore, the rule-based approach requires close collaboration between departments involved in manufacturing – like engineering, production, and even customer support – to pool their expertise for the diverse causes of equipment breakdown. Then, you can develop a virtual model that visualizes how these causes interact through different IoT devices.
For example, a temperature sensor spots overheating problems, and the model knows that a high temperature can also impact the hydraulic pressure. It then checks the pressure sensor data as well. If both the temperature and pressure readings are abnormal, the model will enable the system to automatically alert corresponding departments. This allows you to proactively manage your whole system instead of just responding to separate problems.
Machine Learning-Based
This approach is more advanced. One 2024 research indicated that machine learning is primarily used for fault diagnostics and anomaly detection. It utilizes algorithms to discover patterns by gathering real-time data from the Industrial Internet of Things (IIoT) devices. As such, this method analyzes vast datasets to make decisions about equipment conditions and quickly spot even minuscule signs of problems.
Machine learning also has the ability to constantly learn from past and real-time data to enhance its predictions. This allows ML algorithms to predict more accurately when a machine is likely to break. However, the efficiency of machine learning depends much on ML techniques and PdM data used. The research stated that, for example, ML algorithms like Extreme gradient boosting (XGBoost) and RF outperform Logistic regression (LR) in forecasting the maintenance of printing machines.
PdM Technology Trends to Consider in 2024
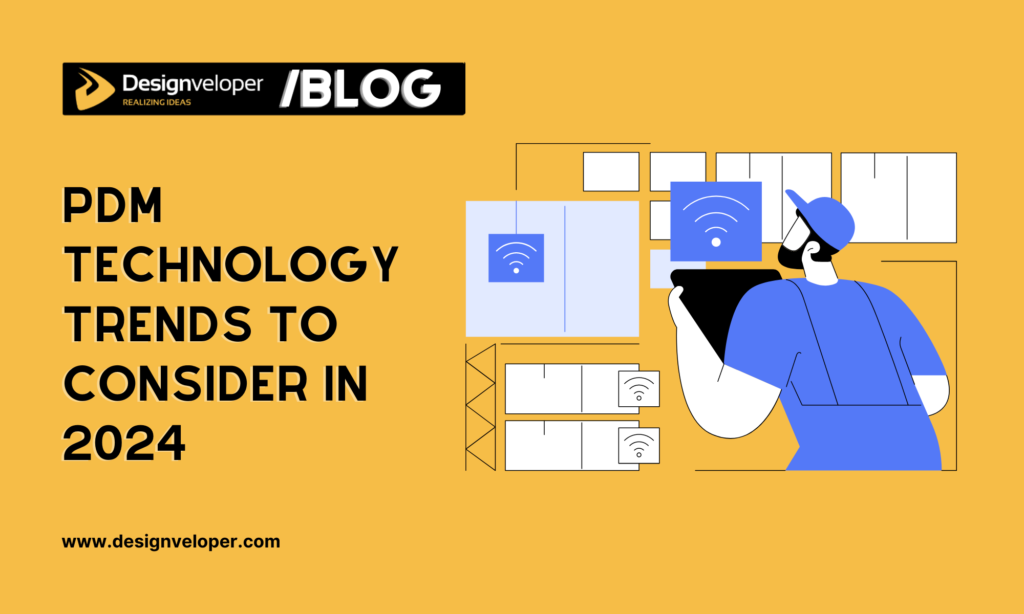
Machine learning, IoT, and Big Data are common technologies behind the success and growth of predictive maintenance in manufacturing. In addition to them, we also witness a rise in other cutting-edge technologies that contribute to shaping the future of PdM:
Digital Twin
A Digital Twin is a virtual representation of a physical machine that reflects how the machine behaves in real-time through data. How? Sensors attached to physical equipment gather and send real-time data to its digital twin. This allows you to simulate, manage, and analyze the performance of the physical machinery in a digital environment. For this reason, you can boost predictive accuracy, reduce downtime & maintenance costs, and enhance decision-making.
Inspection Technology
This refers to all the tools, systems, and techniques to monitor and evaluate the overall health of machinery. They include visual inspection tools (e.g., drones or infrared scanners), non-destructive testing (e.g., ultrasound or X-ray scanning), vibration analysis, thermal imaging, etc. These techs help manage equipment remotely and spot issues early without disrupting operations or posing risks to plant personnel.
VR/AR
Immersive techs like Virtual Reality (VR) and Augmented Reality (AR) boost visualization and real-time monitoring of equipment. VR can create the machinery’s detailed 3D model that you can interact with to visualize its specific components. Meanwhile, AR allows you to track performance data and automatically scan components in real-time through smart devices (e.g., glasses).
Benefits & Challenges of PdM in Manufacturing
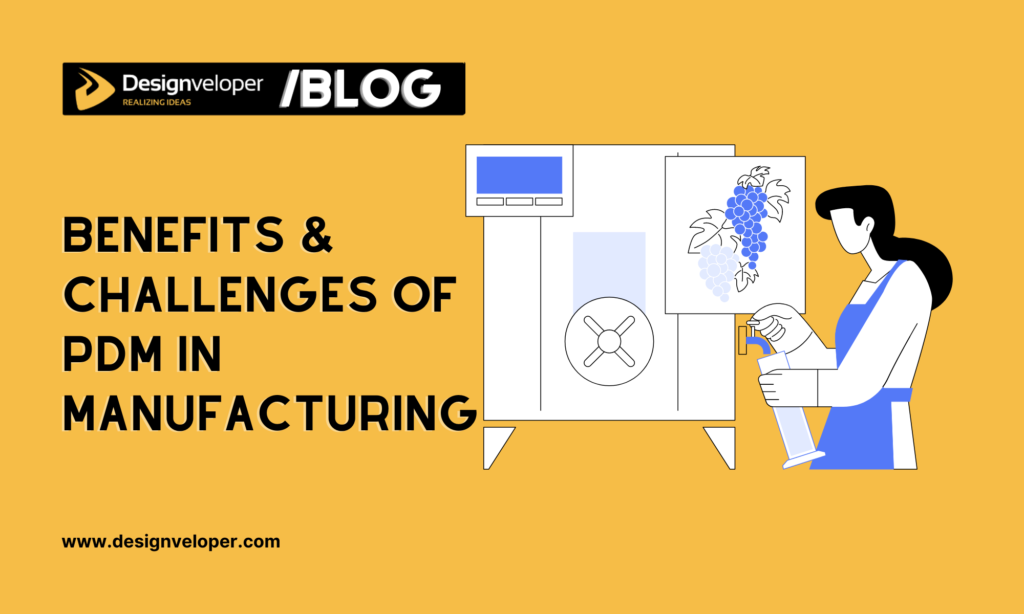
You’ve understood what PdM in manufacturing is and how it works. Now, we’ll delve into the possible advantages and disadvantages you should consider before adopting this maintenance practice in your manufacturing system.
Pros of PdM in Manufacturing
Predictive maintenance benefits the manufacturing industry in many ways. Let’s take a look:
Reduced Downtime & Extended Equipment Lifespan
As already mentioned, PdM uses sensors, data analytics, and other cutting-edge technologies to identify early signs of wear or failure before they turn into big problems. This enables you to schedule maintenance promptly, thereby reducing downtime and expanding the lifespan of machines. As such, less downtime means fewer interruptions. This keeps production running smoothly and avoids expensive breakdowns.
Optimized Resource Allocation & Lower Maintenance Costs
PdM allows maintenance to be conducted only when needed. This removes the need to follow rigid maintenance schedules, hence saving more resources (e.g., labor, time, and costs) than other practices like preventive maintenance. In other words, your maintenance team can focus their effort on machinery that actually needs attention. This helps optimize resource allocation, which may reduce maintenance costs in the long run.
Enhanced Safety & Quality Control
We can’t deny that PdM helps create a safer working environment. By anticipating breakdowns in advance, it can stop dangerous situations that arise from sudden machinery failures. As such, there are fewer risks to plant personnel, such as workers, if machines perform reliably and stably. Moreover, predictive maintenance ensures that machines can produce high-quality and consistent products. If any signs of defects appear, your maintenance team can interfere promptly to prevent their impacts on the output’s quality. This helps enhance quality control.
Improved Operational Efficiency
Predictive maintenance keeps equipment working under optimal conditions for longer periods. This helps minimize unexpected interruptions in the manufacturing process. As a result, you can meet production targets more easily, enhance team productivity, and increase operational efficiency. This, in the long run, will improve profitability and overall business success.
Cons & Solutions for PdM in Manufacturing
Despite its immense benefits, predictive maintenance also comes with possible challenges you need to consider. With the right solutions, you can overcome these hurdles and make PdM adoption easier.
High Upfront Costs of Implementation
Many manufacturers might be hesitant to use PdM because of its high initial investment, let alone further costs related to upgrading or integrating PdM into your existing infrastructure. Here’s why: installing a PdM system involves data storage, sensors, software, and other physical devices if necessary. This can be a large financial burden for smaller manufacturers.
Solution: Using cloud computing can lower setup costs. You can store and analyze data on the cloud instead of installing expensive hardware and on-site infrastructure. This makes PdM more affordable, especially for SMBs.
Data Issues (Volume, Analytics & Security)
We all know that PdM depends greatly on data for predictions. However, monitoring, storing, and analyzing massive amounts of data is never an easy task. Moreover, data security is always the biggest concern of management as any breaches can disrupt operations.
Solution: AI-powered advanced analytics can help process vast, complex databases more effectively. Don’t ignore regular security risk assessments to detect and minimize possible vulnerabilities. Additionally, leverage robust security measures like access control or zero-trust protocols to further protect your data.
Technical Expertise
Another challenge is the shortage of technical expertise. Predictive maintenance systems require employees who are skilled in AI/ML, data analytics, and practical experience with manufacturing equipment. But not all manufacturers can meet this demand. This can slow down the effective implementation and use of PdM.
Solution: Employee training is crucial to address this issue. Upskilling your current workforce or partnering with external specialists will help your company make full use of PdM.
5 Examples of PdM in Manufacturing
How do companies successfully implement predictive maintenance in their manufacturing processes? Let’s look at five typical examples to better understand this:
1. BASF
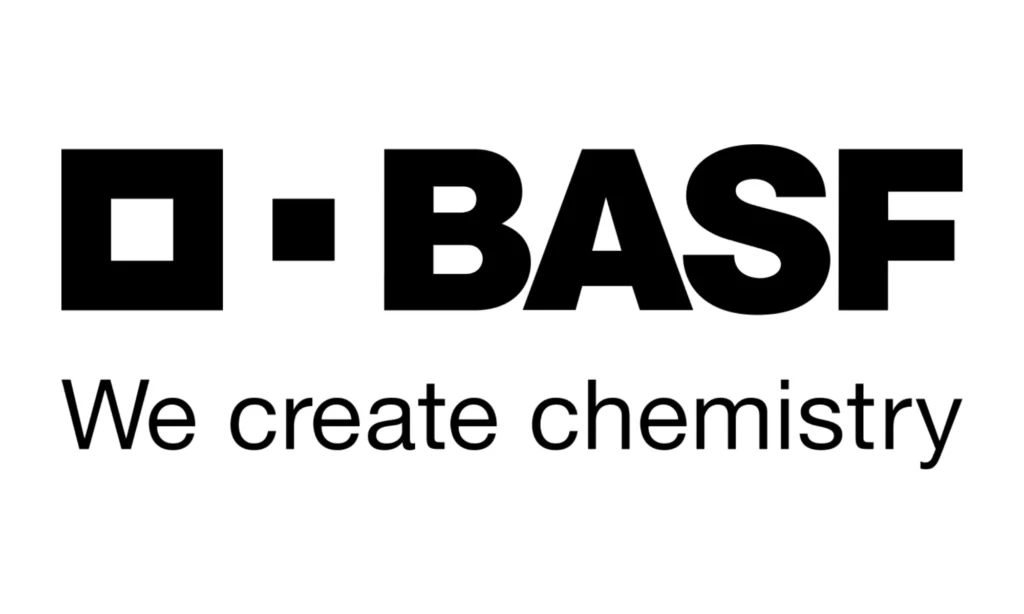
BASF is the world’s largest chemical company. They specialize in a diverse range of products like construction materials, wearable devices, and mass transportation. When BASF built a new electrical substation at its Beaumont, Texas plant, the company leveraged EcoStructure Asset Advisor to monitor its operations remotely for maximum plant uptime and productivity.
This IoT-enabled solution is a condition-based monitoring and predictive analysis system. It’s connected with devices like TeSys motor control systems, UPS units, or variable frequency drives to constantly collect and analyze data on the health of 63 prime assets through BASF’s dashboard. Lee Perry, an Electrical Design Engineer at BASF, said that the use of predictive maintenance helps predict asset performance more accurately. Further, continuous monitoring and prompt anomaly identification prevent catastrophic failures, increase plant safety, and improve operational efficiency.
2. Toyota
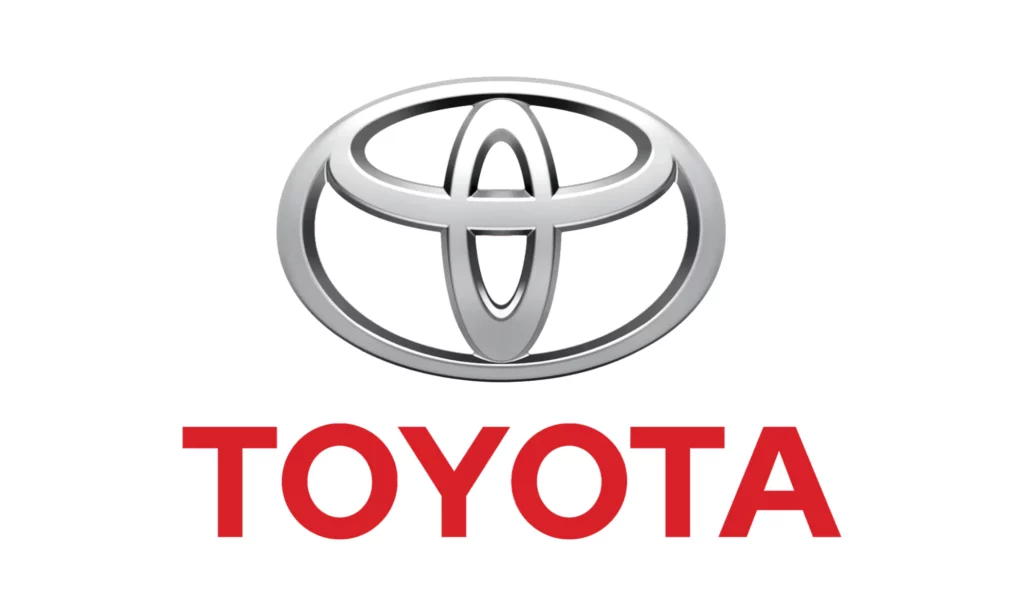
Toyota, in partnership with IBM, has created a smarter, more digital factory for its Indiana branch (North America). The company confronted a big challenge: as a car rolls off the assembly line every minute, each process in a vehicle’s assembly needs to be flawless to deliver a high-quality product while maintaining production pace. This required them to minimize downtime and have zero defects during manufacturing.
Trever White, a Group Manager & Divisional Information Officer at Toyota North America Indiana, shared that the company’s goal is to consolidate a common maintenance platform that supports better customer experience and faster predictive decision-making. He believed that factory floor equipment, combined with AI and IIoT, can gain critical insights into reducing downtime and defects.
That’s why Toyota leveraged Maximo as their enterprise asset management system. This cloud-based platform will contextualize and integrate PLC (Programmable Logic Controller), sensors, and existing manufacturing data like work orders. It then uses AI to generate better insights into the health of equipment and its components, monitor any anomalies, and make maintenance work more proactive. The platform also allows Toyota to measure the efficiency of its maintenance operations.
As a result, Toyota can increase uptime by 10-20%, reduce operational costs by 15-50%, and mitigate risks, especially compliance, by 5-10%.
3. Alcoa
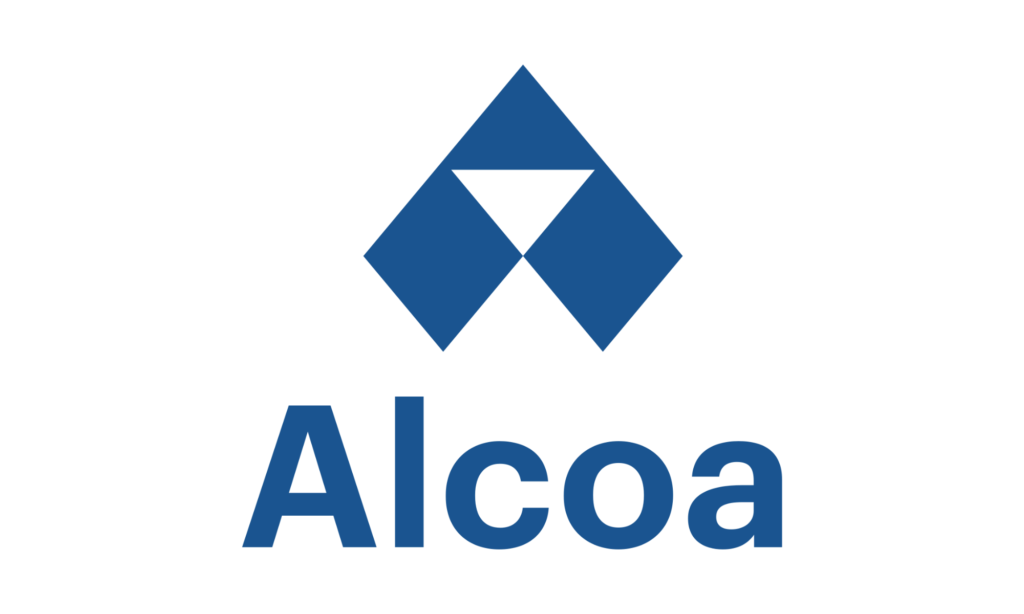
Alcoa is one of the world’s biggest producers of aluminum, alumina, and bauxite. The company believed that predictive maintenance is a strategic factor that helps its production be more stable and profitable. So, they leveraged the AVEVA PI System to gather real-time sensor data while storing maintenance data in Oracle eAM. However, Alcoa struggled to combine these two pieces of information to monitor equipment health and predict its failures effectively.
Alcoa used Senseye – a predictive maintenance tool – to address this issue. This system isn’t developed to store data or connect to sensors. So, to use the data already collected by Alcoa’s systems, Senseye depends on the Asset Framework of the AVEVA PI Server. The Asset Framework creates a digital copy of Alcoa’s physical equipment and uses Event Frames to catch abnormal events from the sensors. Senseye then uses these digital copies and event frames, coupled with the PI Web API, to analyze historical and real-time data. This allows Alcoa to predict when a machine is likely to break down.
As a result, Alcoa can decrease unplanned downtime by 20%, reduce maintenance work hours by 10%, and lower maintenance costs.
4. Mondi Gronau
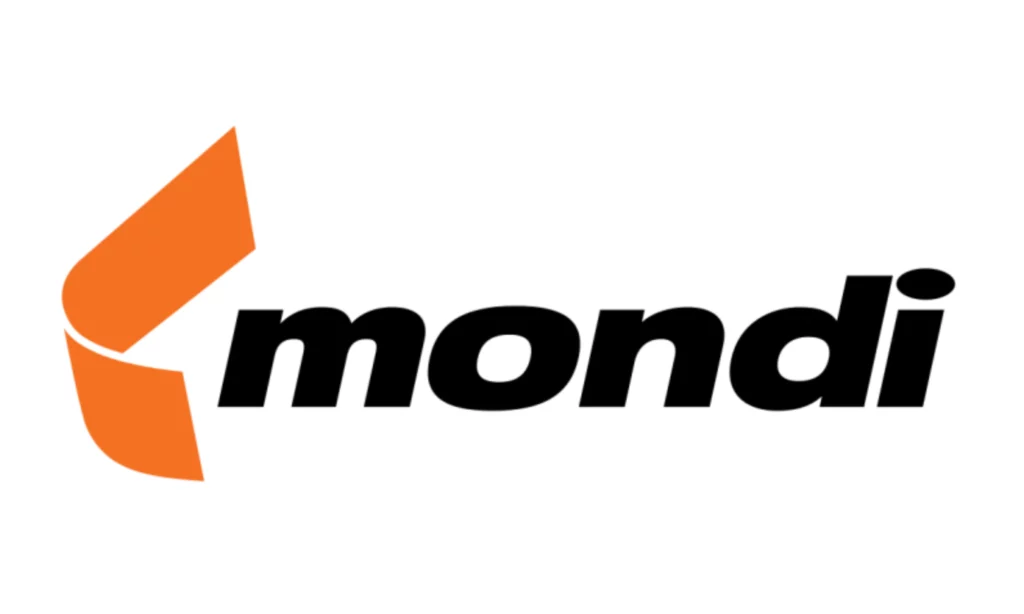
Mondi Gronau is the world’s international leader in the production of packaging and paper products. Its plant has roughly 60 large, complex machines that are controlled by five PLCs and may generate 7 gigabytes of data per day. However, Mondi met difficulty with using this data for predictive maintenance as its plant personnel lacked technical expertise and experience in machine learning and data analytics.
To avoid unexpected costs caused by machinery failures and maximize plant efficiency, Mondi outsourced a PdM app development using MATLAB. This system uses ML algorithms to predict machinery failures and keep production running reliably 24/7. Dr. Michael Kohlert, a Head of IT and Automation at Mondi, said that they saved more than 50,000 euros annually after the launch of the software.
5. Nestlé
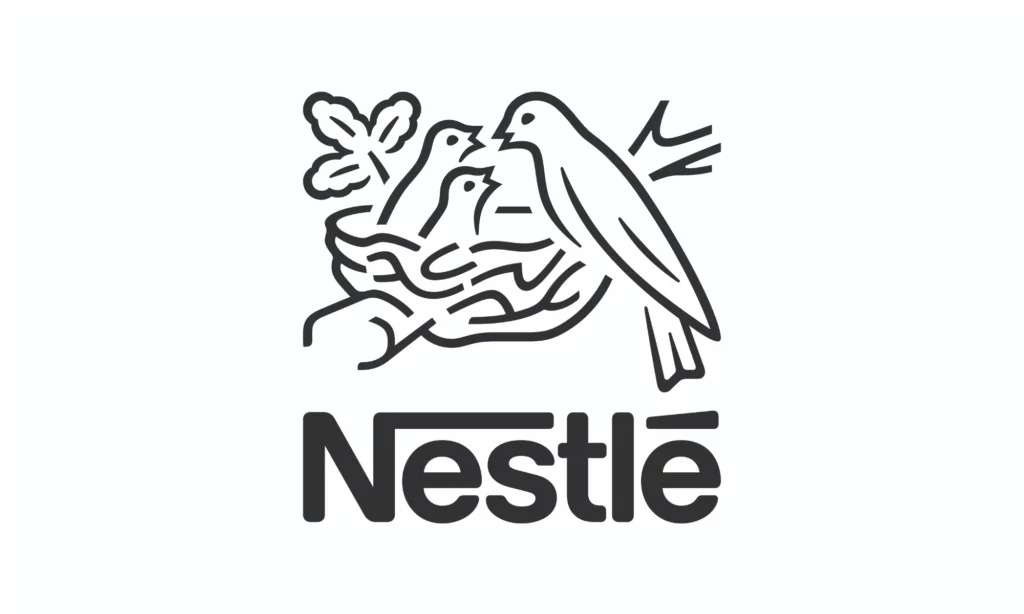
Nestle is one of the largest food and beverage companies in the world. Striving to become a “cognitive business” with machine learning tools, Nestle has adopted AI, predictive maintenance, and robotics in different aspects of its business, from manufacturing to management and front-line customer support.
One biggest success of Nestle lies in its Dubai-based AI Maha factory. Opened in 2017, this facility focuses on NESCAFÉ and MAGGI products. To improve asset performance, avoid unplanned downtime, and optimize electricity loads, Nestle used EcoStruxure technology to assess real-time data and conduct cloud-based predictive analytics to promptly detect possible defects. Further, the technology can suggest predictive maintenance schedules, which allow Nestle’s teams to monitor assets more proactively.
5 Steps to Conduct Predictive Maintenance Effectively in Manufacturing
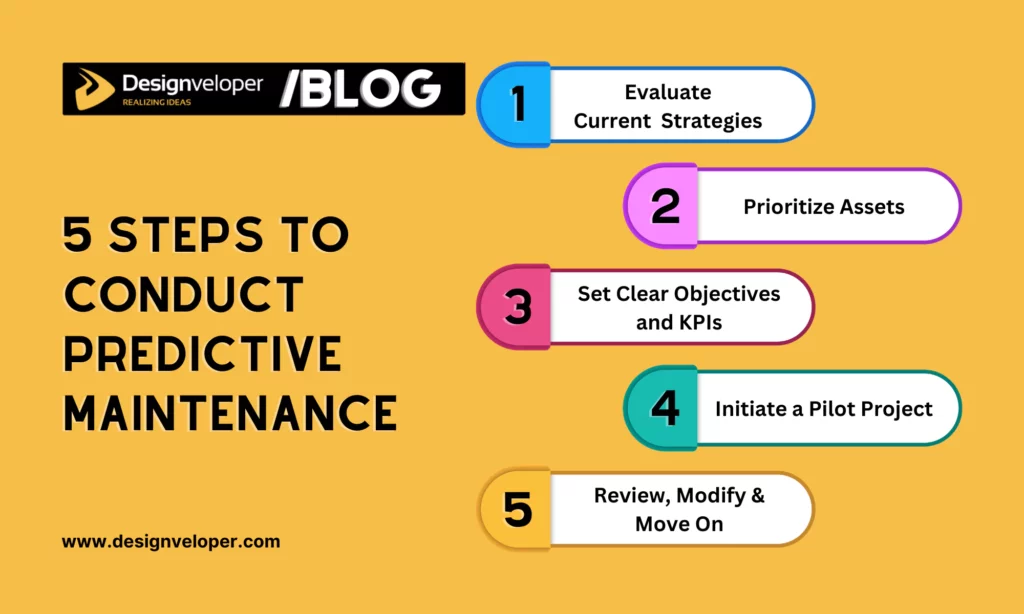
You’ve seen how global companies have successfully applied predictive maintenance in manufacturing. Now, it’s your turn to consider this approach in your plant. Below is a step-by-step guide to help you devise an effective PdM strategy before you dive into this realm:
Step 1: Evaluate Current Maintenance Strategies
Start by assessing your existing maintenance practice. Check whether they work effectively for your maintenance purpose and identify any gaps where your current plan may be falling short. In case you don’t have a formal maintenance strategy, look into your historical data to find patterns related to machinery failures and downtime. This evaluation can help you understand where PdM offers your plant the most benefit.
Step 2: Prioritize Assets
Not all assets need predictive maintenance immediately. To focus your efforts and ensure cost-effectiveness, you should identify which machine has a significant impact on manufacturing or where failure may lead to large costs.
Step 3: Set Clear Objectives and KPIs
Next, establish the specific goals you want to achieve with PdM, be it reduced downtime, enhanced safety, or extended equipment lifespan. Having clear objectives with measurable KPIs helps you track maintenance plans easily.
Step 4: Initiate a Pilot Project
Once you’ve developed a comprehensive PdM strategy or application, how can you know it works well for your system? Test it with a pilot project beforehand. Like in the case study of Alcoa we mentioned above, the company started its new PdM strategy at the Fjardaal aluminum smelter and observed its efficiency before rolling it to other assets. If a strategy proves ineffective in predictive maintenance, you may fine-tune it more easily without disrupting a whole operation.
Step 5: Review, Modify & Move On
When the pilot project is underway, frequently review the performance of your PdM strategy. Then, track your KPIs and compare the results with the initial goals. Refine the strategy based on real-world data and challenges. Once your PdM strategy works effectively after improvements, expand it to more machines.
Final Words
Now, you’re here! After discovering predictive maintenance in manufacturing, we expect you to grasp how it works, what technology trends it comes with, and which benefits or challenges it has. Understanding PdM without the right strategy can prevent you from making full use of this maintenance practice. Therefore, follow our structured guide to devise the appropriate framework and strategies for your PdM adoption. For more interesting news about this topic, subscribe to our blog!
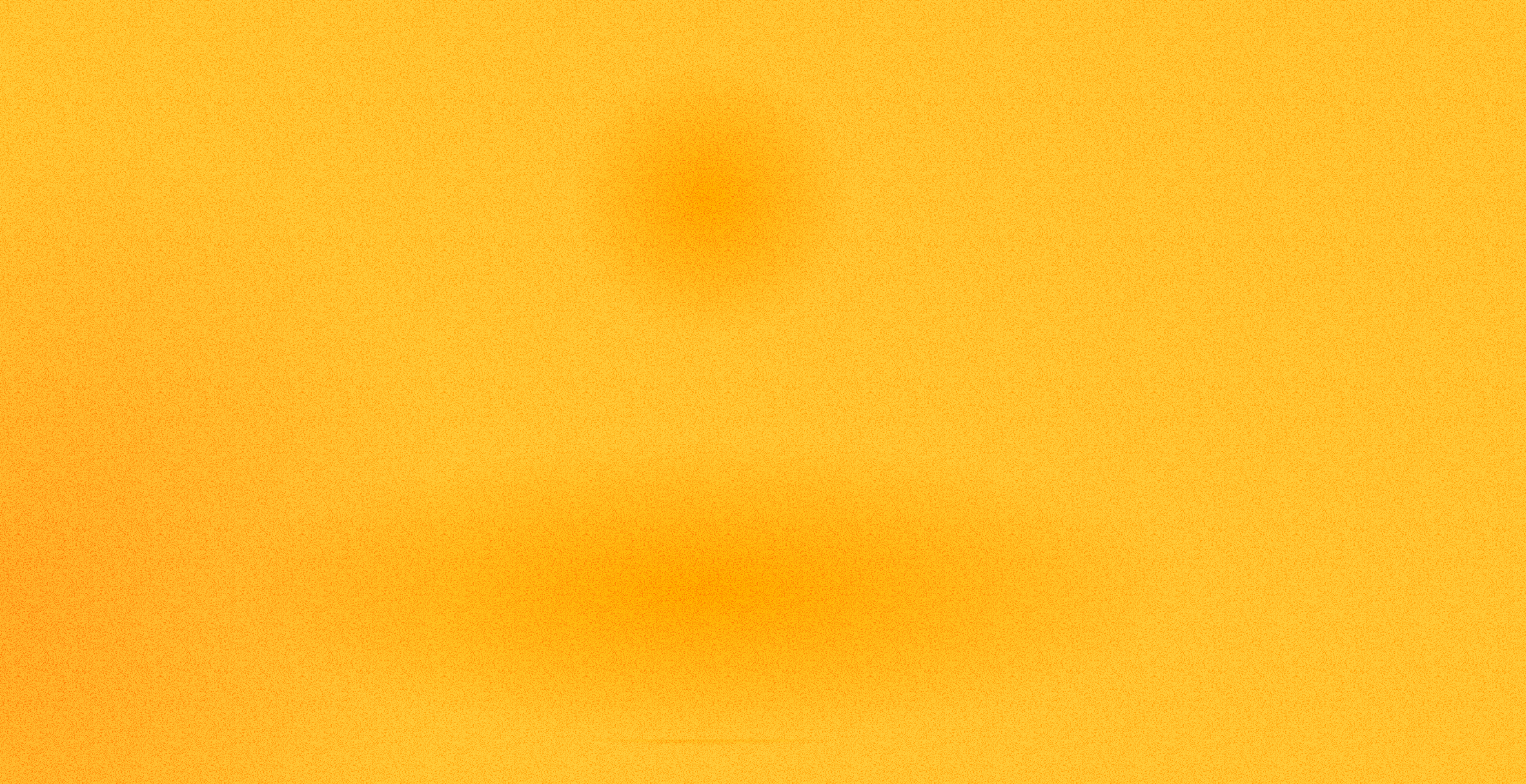
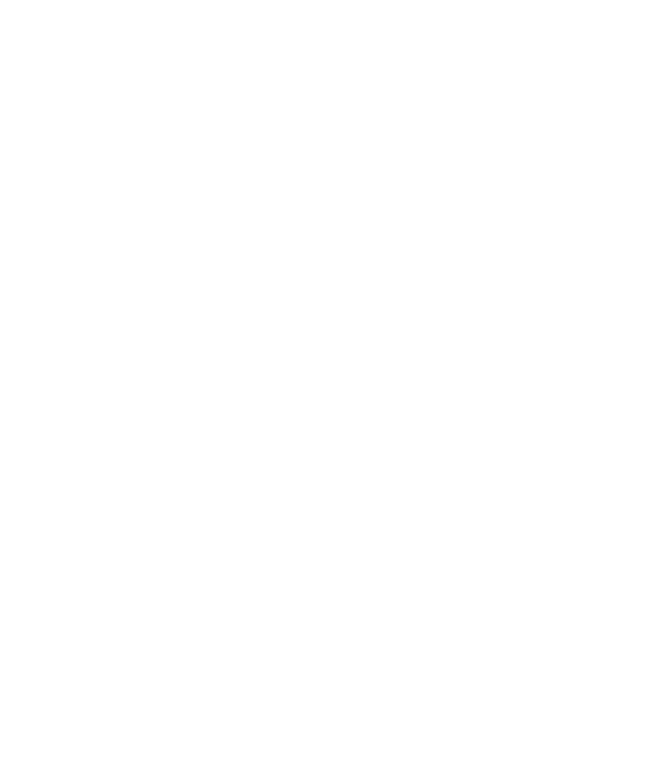
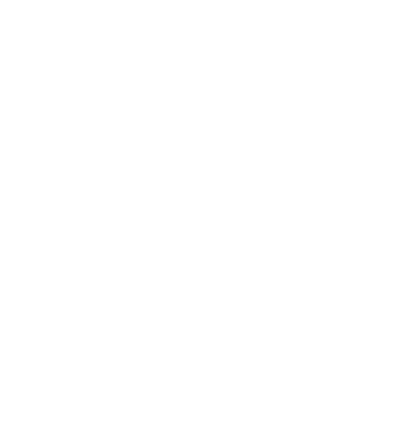
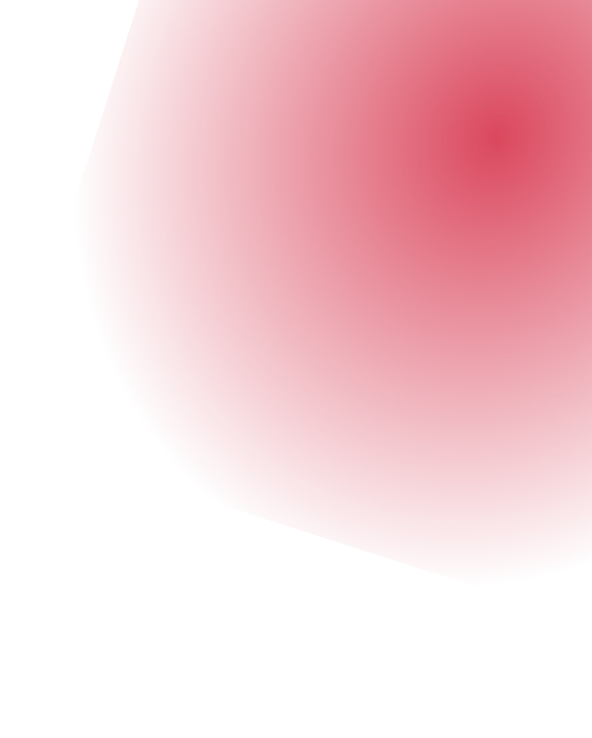
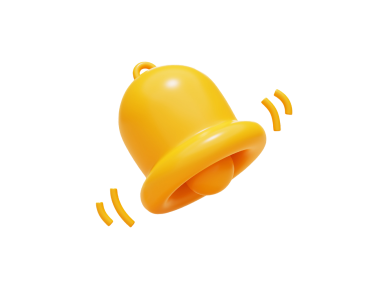
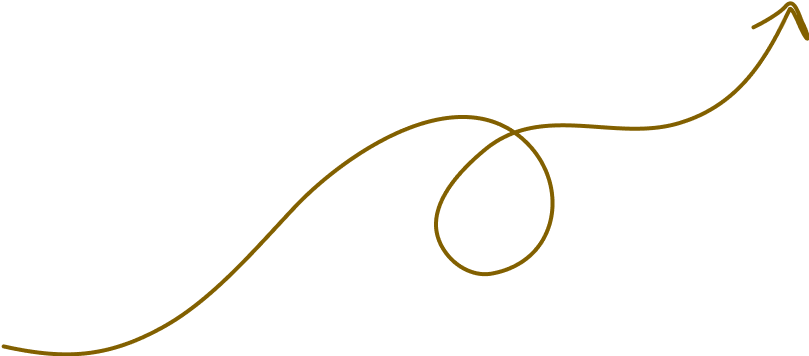