How to Derive Insights From Data with 5 Key Steps
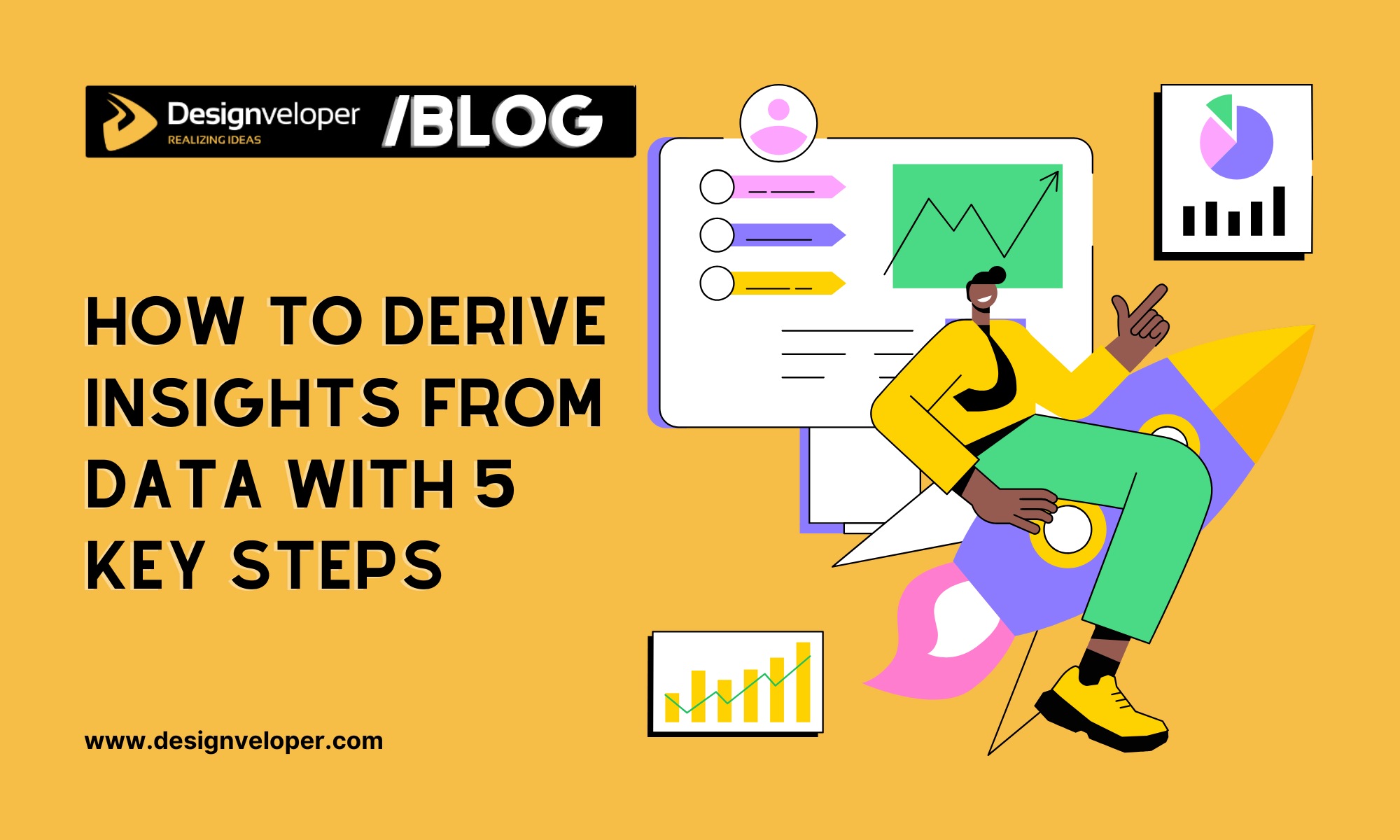
Thanks to tech advancements and the growing internet connection, we can now approach a huge volume of data. This data helps us understand our target customers and streamline operations, be it sales figures, customer feedback, or market research.
However, this raises a big challenge among many companies: converting raw data into actionable insights. In other words, how can they use this information to develop a marketing campaign or make operational decisions that boost sales and productivity? The answer lies in the master of deriving meaningful insights from data – a crucial skill that turns your challenges into opportunities.
If you want to know how to do this effectively, keep reading! This article is a golden mine for discovering everything about data-driven insights from A to Z.
What are Insights from Data?
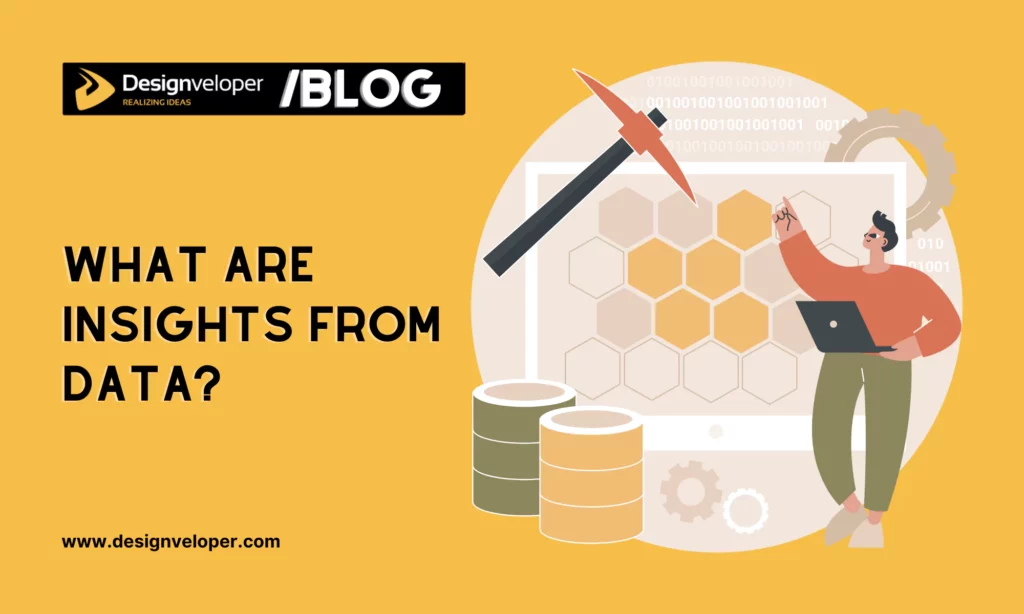
Insights from data mean actionable knowledge you obtain from analyzing raw data. These insights are not confined to only numbers or observations. Instead, they provide a detailed view of patterns or trends (e.g., customer behaviors or market changes) that support informed decision-making.
When working on data analytics, you might hear of these terminologies: “raw data,” “information,” and “insights.” While raw data is the foundation, information will structure it and actionable insights will transform it into a valuable tool to make the right decisions. Here’s how:
- Raw data is unorganized and unprocessed. It consists of numbers, text, and observations you gather from different sources. For instance, raw data can be the monthly sales figures of your crossbody bag across store branches.
- Information is extracted after raw data is processed and displayed in an understandable way. It helps you answer common questions like “How much?” For example, information can be a report showing a 15% decrease in the bag’s sales last month.
- Insights explain why something happened or what to do next. In the example above, you can obtain insights into why sales declined (i.e. because the bag’s design lacked appeal to a certain demographic). Several analytics tools are even equipped with AI capabilities to give suitable recommendations (i.e., redesign the bag and market it differently).
Why Should You Derive Insights from Data?
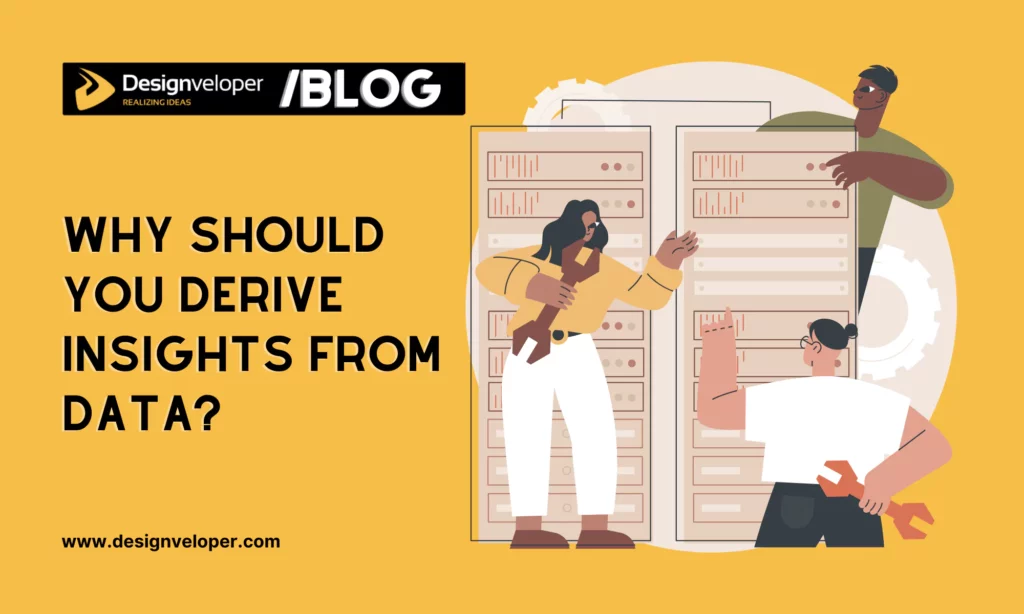
Most companies (72%) are implementing data management strategies to gain insights through analytics and reporting. So why is this so essential? The answer lies in the immense power of meaningful insights. Let’s learn how these insights can open new avenues and drive success for your company:
Inform Decision-Making
Actionable insights play a key role in driving better decisions.
Instead of depending on guesswork, you can conduct data analytics to identify what works well and what needs improvement. Insights derived from this analytics also help answer which factors contribute to that success or failure.
Suppose you newly launched an outerwear collection. By analyzing customer behavior towards this collection, you might realize why a certain group of customers prefer specific colors or materials. You also discover how endorsements from social media influencers drove sales last month. These insights give you an evidence-based guide to future product development and marketing strategies.
Mitigate Risks
Insights from data help you reduce unexpected risks.
For example, during the manufacturing process, cameras or sensors are often embedded in machinery to track its performance continuously. Analyzing data sent from these tools will give a detailed insight into whether the equipment is working well or likely to break down.
Further, insights help spot suspicious spending patterns that point out fraud. This allows for proactive action before these problems escalate.
Stay Competitive
Our world is flooded with data, especially mobile data traffic estimated to reach over 300 exabytes monthly in 2029. Those who can derive insights efficiently can stay ahead of customer expectations and market trends. This gives them a competitive advantage over rivals.
For instance, advanced data analytics reveals a growing interest in products made from sustainable or recycled materials. This offers you a new idea of developing a sustainable product line to meet this emerging demand and boost customer satisfaction.
Various companies like Amazon or Netflix even use AI predictive analytics to get insights into customer preferences. This allows them to provide a personalized experience to customers by suggesting tailored products.
How Companies Use Data-Driven Insights in Reality
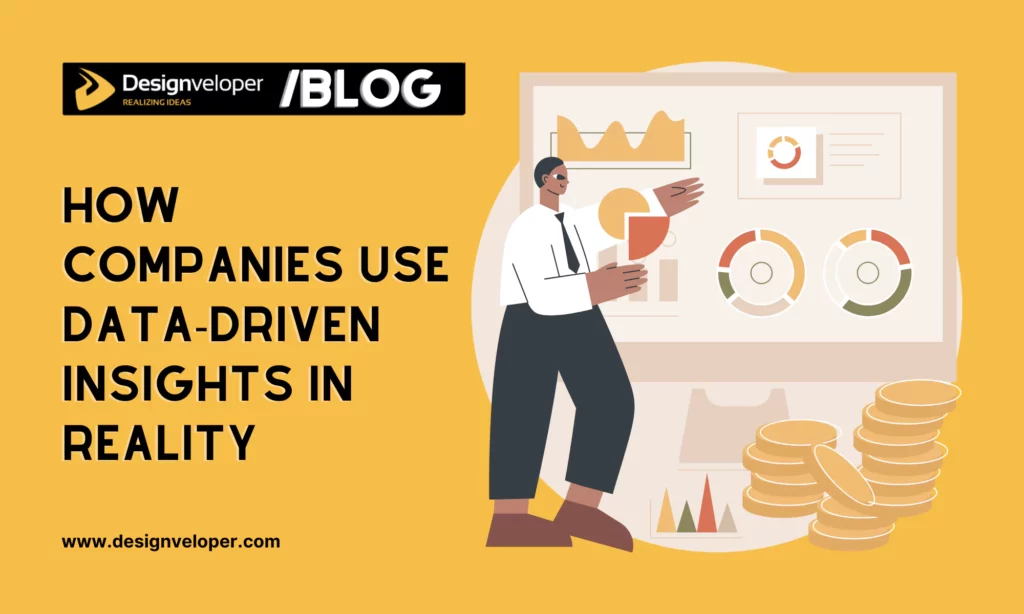
To better understand how insights derived from data benefit business operations, let’s learn how companies worldwide use these insights in reality:
1. Netflix
Netflix collects data on user behavior (for example, viewing history, ratings, watching duration, or the time of the day users enjoy their favorite shows). By analyzing this data, Netflix’s AI algorithms can forecast accurately which series or shows viewers are likely to prefer and offer them personalized recommendations. More than 80% of the content people watch on Netflix, accordingly is attributed to this recommendation system.
2. Walmart
By analyzing large volumes of sales and inventory data, Walmart extracts meaningful insights for supply chain optimization.
For instance, in 2004, the retailer leveraged predictive technology to harness trillions of sales data bytes from recent hurricanes. This data helped them discover an unexpected trend: customers tended to stock up on beer and toaster pastries ahead of a hurricane.
With this insight, Walmart proactively filled these items to stores on the path of Hurricane Frances to meet the growing demand. This gave Walmart a competitive edge and better serve its customers during harsh times.
3. Designveloper
As one of the leading software developers in Vietnam, Designveloper has helped numerous clients achieve their business goals with data-driven insights. In one retail project, the client came to us for an eCommerce website. However, they struggled with identifying the website’s key features that align with their target users.
To resolve this challenge, our data analytics team analyzed customer behavior and relevant data. This data consisted of page views, time on page, click-through rates, shopping cart conversion rates, and more. We also conducted descriptive analysis to detect different patterns in customer behavior, such as age, user flow drop-offs, or feature usage frequency.
Through data analytics, we made rigorous evaluations and added new functionalities that fit end-user demands. Further, we temporarily deactivated unnecessary features. Consequently, the website gained a good result:
- Higher page views, from 100 visits to 20,000 visits per month;
- Longer time on page, from an average 1.2 minutes to 9.3 minutes per visitor;
- More interactions with new features (particularly, 70% of users showed interest in search bar and filters);
- Higher payment rates, from 12% to 33%.
This eventually drove sales for the retailer.
4. Cleveland Clinic
Cleveland Clinic is leveraging real-time data to make its hospital operations more intelligent and efficient. By analyzing this data, they gain meaningful insights into current and even future hospital status. For example, the hospital can accurately predict how many patients will visit in the next 24 hours, enabling better preparation for staffing and other resources (e.g., beds or equipment).
5. JPMorgan Chase
JPMorgan Chase combines big data and machine learning algorithms to detect fraudulent activity more precisely. By comparing normal patterns learned from historical data, AI can give JPMorgan Chase’s cybersecurity team an actionable insight into whether real-time transactions are suspicious. If it discovers any anomalies, it’ll flag them for further investigation.
5 Essential Steps to Derive Insights from Data
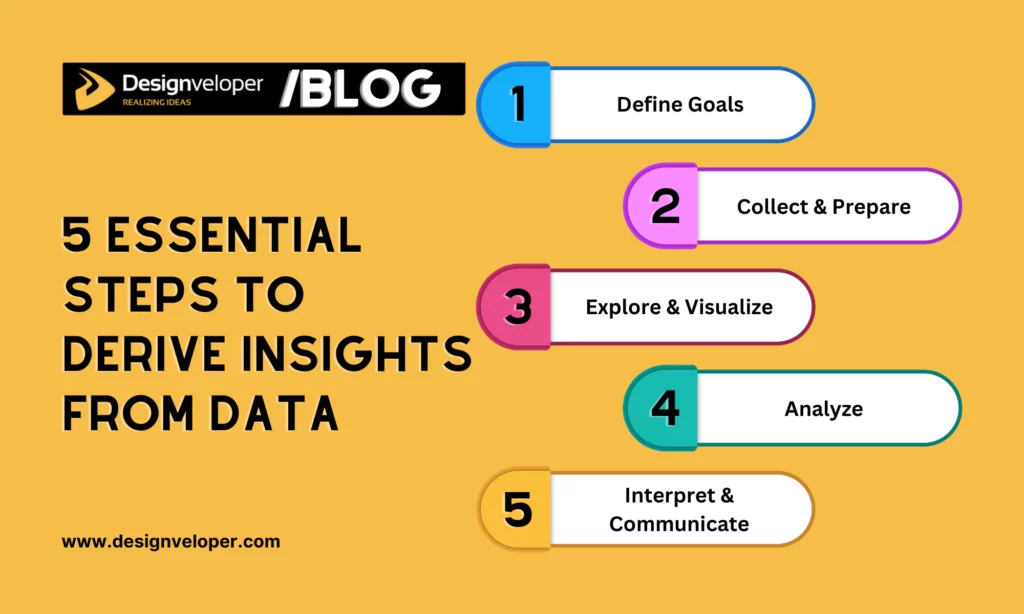
The previous section shows you the importance of data-driven insights in reality. But not all companies can successfully derive actionable insights from their data sources. So here, we’ll elaborate on five important steps to help you make data-driven decisions more efficiently:
1. Define your goals and questions
Today, your business can approach massive amounts of data daily. This data comes from not only internal sources like customer databases and sales records, but also external sources such as social media or industry reports.
Each piece of data helps you answer different questions. So, start with a clear goal to ensure your data analysis will meet your objectives and offer your desired insights. Without a well-defined purpose, you risk wasting time analyzing irrelevant data or overlooking meaningful insights.
To define your goals and questions, you should:
- Clarify what you want to achieve with the data. For example, you want to improve holiday season sales by 20%.
- Generate measurable questions that your data may help answer. Some example questions might be:
- Which customer groups drive the most revenue?
- What marketing strategies generate the highest ROI?
- Why did the sales decline in Q3?
2. Collect and prepare your data
Next, collect and prepare relevant data for your analysis. This step is crucial because it would ensure your data is of high quality to produce reliable and accurate results. So, how can you gather and prepare relevant data? Here’s what you can do:
- Pull data from reliable sources. These data sources can be internal systems (like CRM software), external databases, or APIs. The data you collect can be structured (like spreadsheets) or unstructured (like images or social media posts).
- Clean and pre-process the data. This might involve:
- Eliminate errors or duplicate records;
- Filling in missing values by using statistical methods and interpolation;
- Standardize data formats, for example, writing all dates in the same format (e.g., MM/DD/YY) to ensure consistency.
- Convert data into an appropriate format for analytics, like transforming raw log files into relational tables.
3. Explore and visualize your data
This step provides a foundational understanding of the data. For example, it discovers data trends or patterns like “sales recorded highest in December and lowest in May,” and presents these patterns visually.
In this step, data analysts use descriptive statistics and visualizations (e.g., charts or tables) as the key exploration techniques.
Descriptive statistics refers to calculating such basic metrics as mean, median, mode, range, variance, and standard deviation. For example, when Designveloper worked on an eCommerce website for household appliances, we used descriptive analytics to summarize and describe the data’s features and changes. We concluded some of the key patterns:
- The website’s male customers accounted for 40%, versus 60% of females. All were aged 25-50.
- Portable fans were the top-selling product, making up 17% of the total sales.
Once you’ve completed descriptive statistics, it’s time to use visual tools to present patterns. For instance, you can use a line graph to track sales trends over months or a scatter plot to display customer age versus spending. Through these visualizations, you can identify correlations and unexpected changes in data, like a seasonal surge in sales during holiday months.
4. Analyze your data
In case you want to get deeper insights into your data, move forward to this phase. Here, you’ll combine statistical methods and machine learning to reveal relationships, test hypotheses, and make predictions.
Particularly, you apply statistical techniques like correlation or regression to uncover relationships between data variables. For instance, identify whether endorsements from social media influencers significantly drove sales. Besides, you can use AI-powered analytics tools to suggest solutions or predict outcomes, like forecasting demand in the next quarter.
Data analytics is an iterative process. Nothing can ensure the first outcomes produced by advanced analytics tools are useful for informed decision-making. Therefore, it’s essential to perform numerous analyses to verify results and refine your analysis if necessary. This helps you gain actionable insights like detecting high-value customers or forecasting future trends.
5. Interpret and communicate your findings
Insights are useful only when stakeholders understand and act on them. Therefore, clear communication is a necessity to shorten the distance between data analysis and decision-making. Here are some tips to explain your findings effectively:
- Don’t use technical jargon. Instead, interpret your results in practical terms. For example, you should say, “6 out of 10 customers are likely to leave our website as it’s hard to navigate,” rather than saying, “Customer churn probability is 0.6.”
- Present your insights in compelling, yet easy-to-read infographics, dashboards, or reports.
- Elaborate on how these findings affect your business and propose the next actions. For instance, “Based on our analytics, adding advanced filters could encourage visitors to stay longer on our website, from 1.2 minutes to 9.3 minutes.”
Common Mistakes and Tips to Derive Insights Effectively
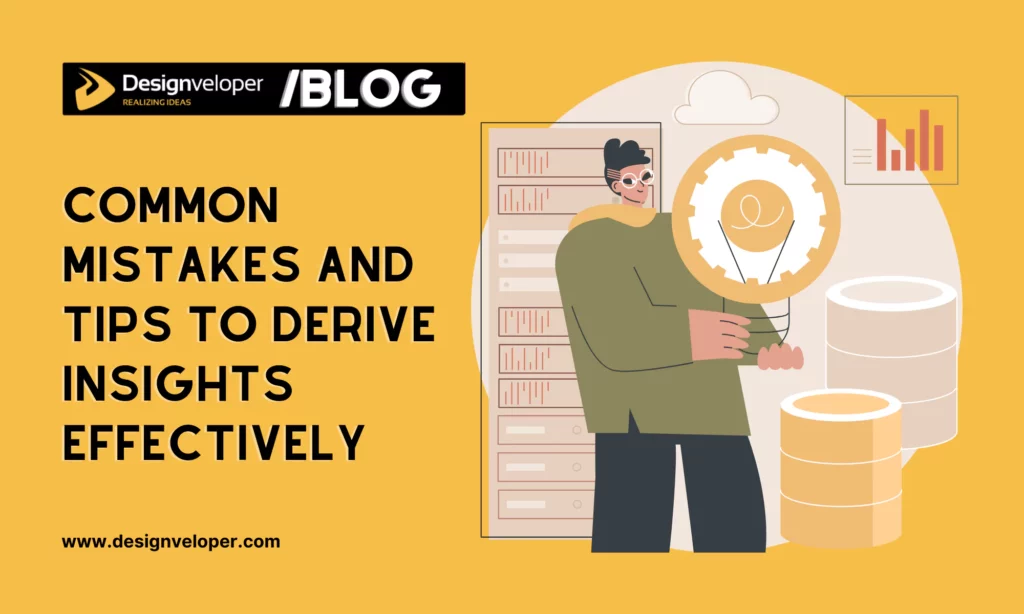
When working with data to derive insights, it’s easy to make mistakes that can result in wrong decisions. Below are common pitfalls and tips to avoid them:
1. Confirmation Bias
This mistake refers to seeking or explaining data in a way that supports their prior beliefs or expectations, without considering contrary information.
Example: Imagine a company starting a new marketing campaign. The marketing team believes that metrics like increased website traffic and social media engagement are indicative of success. So, when the campaign records these positive signs, the team easily interprets it as effective. However, they ignore a dip in conversion rates, which points out the campaign may not be as successful as they initially thought.
How to avoid: To address confirmation bias, you should:
- Approach analysis with an open mind and share your insights with coworkers for feedback. This helps spot and remove possible biases.
- Develop a careful methodology for data analysis, which covers clear goals & questions, data collection, analytics techniques, etc.
- Proactively look for evidence that can disapprove your initial beliefs, instead of only seeking information that confirms them. This helps you get a more complete understanding of the issue.
2. Misunderstand Correlation as Causation
This mistake refers to assuming that one variable is the cause of another. But in fact, there’s not enough evidence to prove this relationship is a causation.
Example: Imagine a company observing higher ice cream sales during the summer. So, they may conclude that hot weather boosts ice cream consumption, without considering other factors like sales discounts or new flavors.
How to avoid: To mitigate this pitfall, you should:
- Investigate underlying causes behind a correlation.
- Conduct controlled experiments to separate the causal relationship between variables.
- Use statistical techniques to quantify the relationship between variables. This helps you predict how one variable depends on another.
3. Overlook Contextual and External Factors
This mistake occurs when you depend only on the data without considering broader contexts and industry trends related to it.
Example: Imagine a company seeing a sudden rise in sales. They might attribute this to the effectiveness of their recent marketing strategy. However, this phenomenon can happen due to a competitor’s temporary stock storage or the act of stocking up more before a hurricane. Without considering these external factors, the company can misconceive the sales data.
How to avoid: To prevent this mistake, you should integrate contextual knowledge and industry benchmarks into your analysis to evaluate your relative position. Accordingly, you can consult domain experts about industry trends and analyze past patterns to stay updated about competitor activity, seasonal factors, or economic indicators.
4. Ignore Data Issues
This pitfall comes from deriving insights from outdated, inaccurate, and incomplete data. Further, data issues might involve data privacy and security.
Example: Let’s say a company uses sensitive customer information with duplicate entries or without consent. This results in skewed results or invades user privacy.
How to avoid: To resolve data issues, you should regularly clean or verify your data to ensure high quality. Plus, use diverse data sources and adhere to data protection regulations or ethical standards during data analytics.
5. Overfitting
This mistake occurs when you develop a machine learning model that works well on the training data, yet fails to analyze novel, unseen data. It’s like a student who can memorize every specific detail of what he/she learned, but struggles to apply the knowledge in practice.
Example: Let’s say a company wants to forecast sales based on historical data. It trains a machine learning model on a massive volume of past sales data (e.g., promotions, holiday season, and economic indicators). The model can identify certain patterns in that dataset. For example, “weekend breaks in summer months lead to high sales at the ABC theme park”. However, when it’s deployed to predict future sales in winter months, it performs poorly. This is because the model hasn’t learned the underlying causes and relationships that generate sales.
How to avoid: To mitigate this problem, you should leverage techniques like cross-validation to make your model generalize well to new situations. Plus, simplify the model with fewer parameters if possible.
Conclusion
This article navigates you through a discovery of how to derive actionable insights from data. Without a rigorous approach and analytics tools, you might struggle with extracting findings to resolve your inquiries. Besides our five key steps, you should consider common mistakes and tips to avoid these pitfalls to conduct more effective data analytics. If you’re excited about this topic, subscribe to our blog for the latest updates!
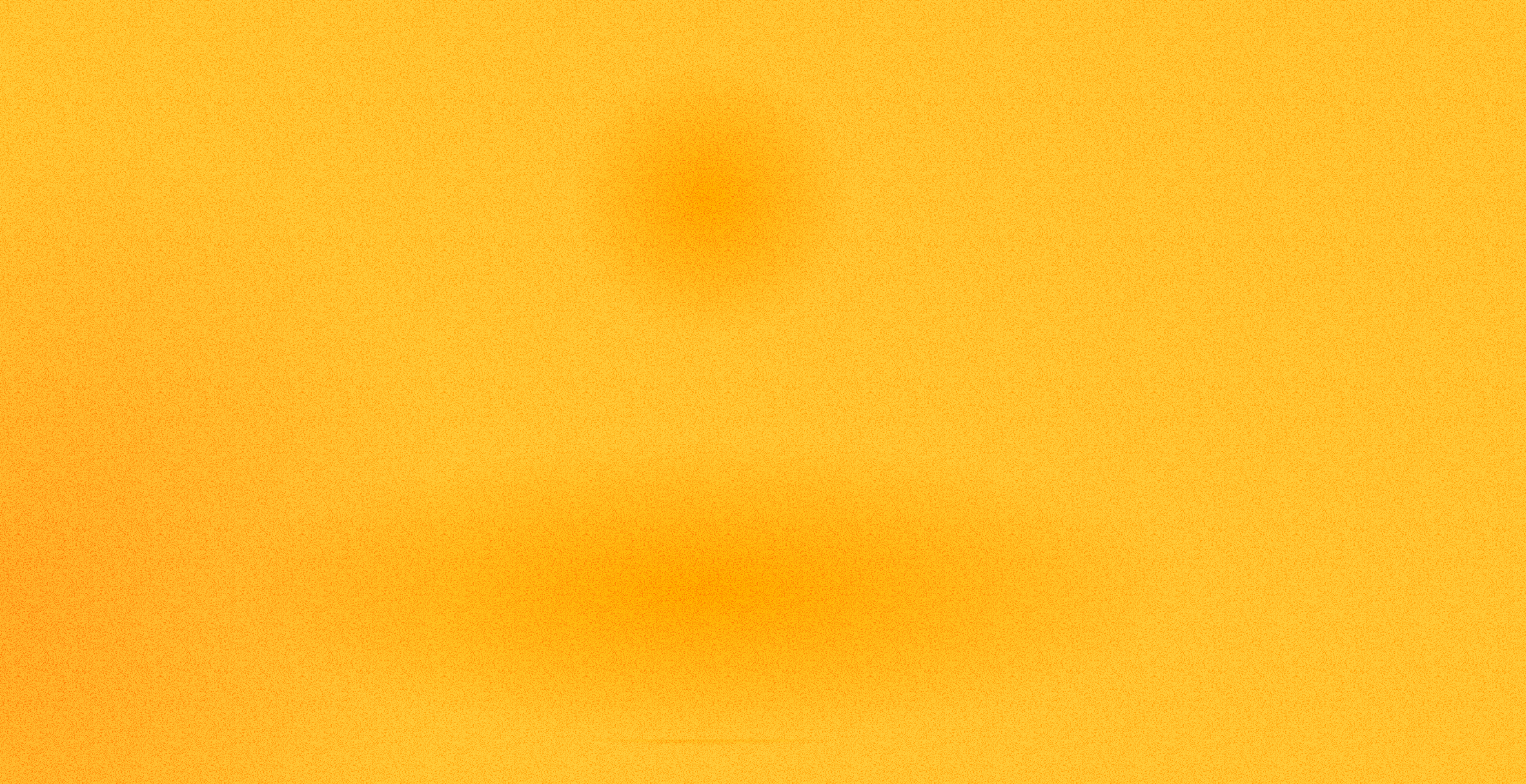
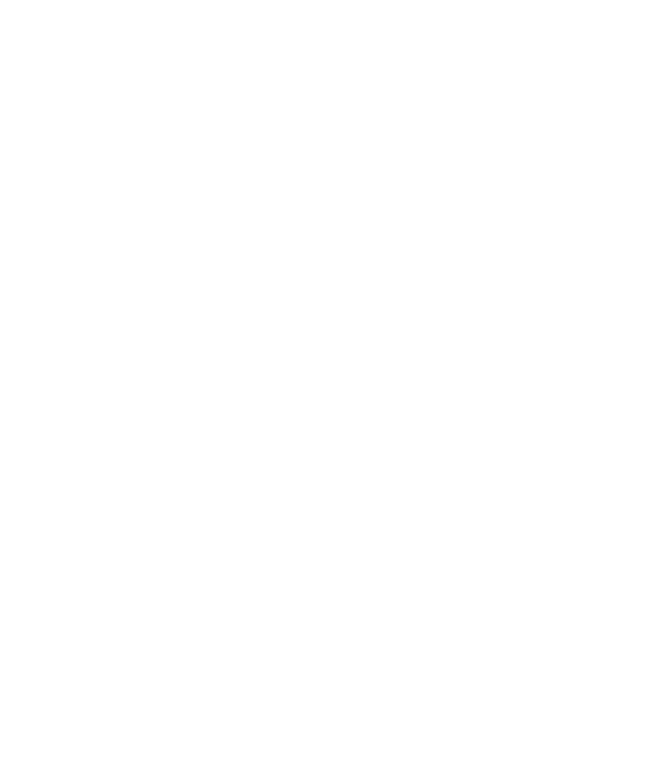
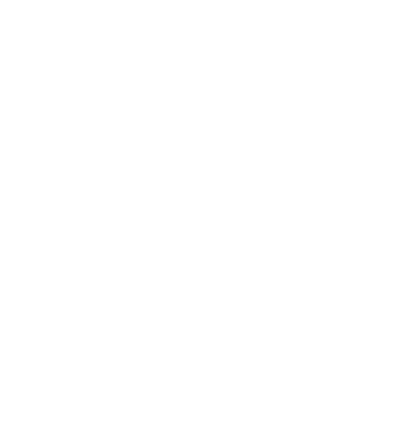
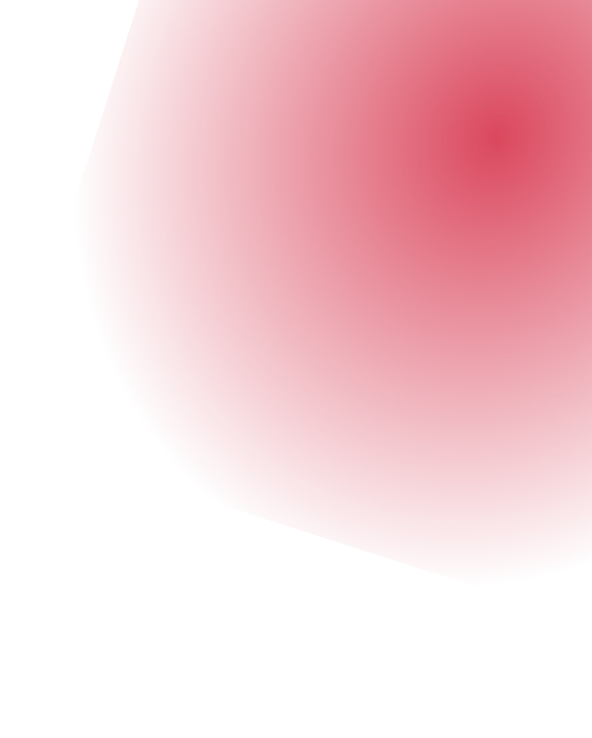
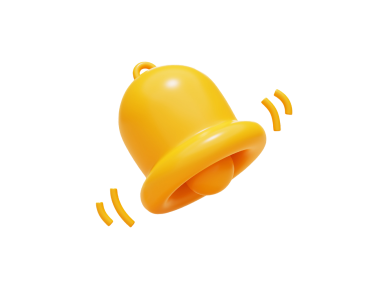
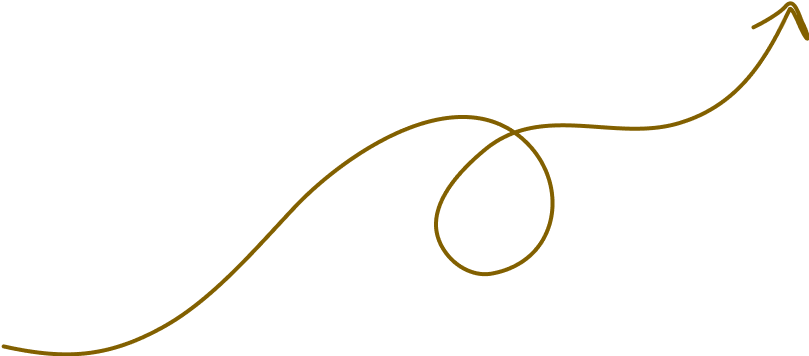