There is a rapid pace in the world of artificial intelligence and machine learning, having new trends every year. As we step into 2025, we have to keep our eyes on recent technology. This article explores 5 AI and machine learning trends to watch in 2025, backed by recent statistics and reports. The methodology and these trends are supposed to change various industries. Let’s dive in and discover what’s on the horizon for AI and machine learning trends in 2025.
Ethical AI Practices
As AI and machine learning trends continue to evolve, ethical considerations remain crucial. At present time, organizations have committed themselves to adopt responsible AI practices in 2025. This is to support both fairness, transparency and accountability.
Most businesses now have a formal AI development ethical charter, compared to only 5% of businesses in 2019. This is significant because of the growth in importance of ethical AI.
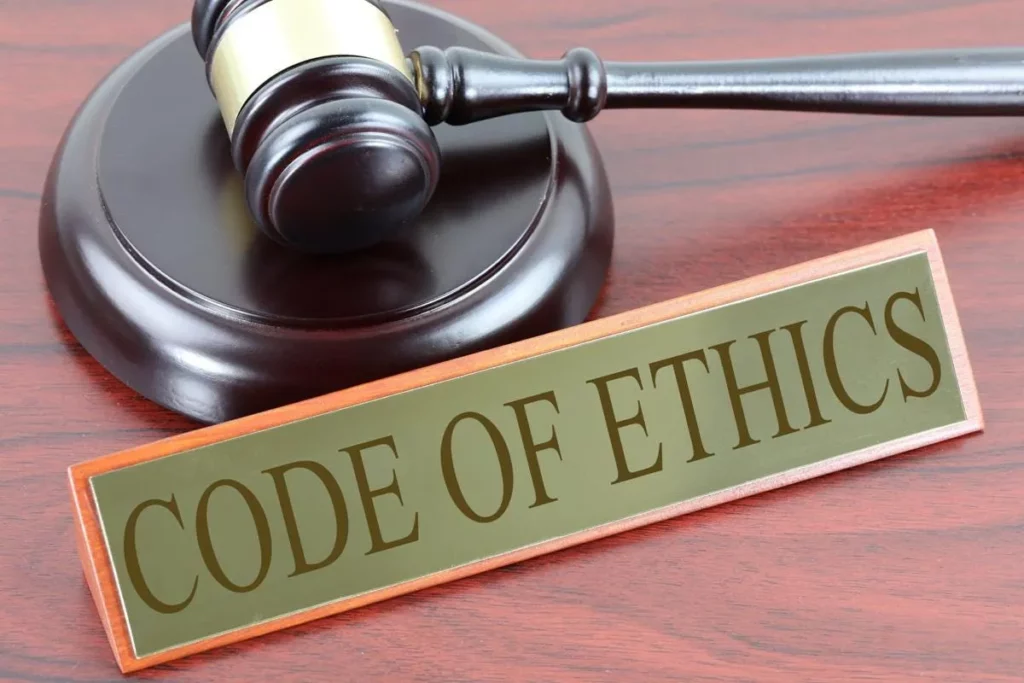
The UNESCO’s ‘Recommendation on the Ethics of Artificial Intelligence’ contains a complete framework regarding AI ethical practices. The World Economic Forum’s AI Governance Alliance also advocates for transparency, accountability, and fairness in AI systems as well.
For example, BMW’s smart maintenance system run by AI cuts the unplanned downtime from 40% by predicting the equipment failures. In this example we see how the ethical AI can improve the operational efficiency by making it fair and transparent.
Policies and Frameworks to Address Biases and Ensure Fairness
In 2025, today’s focus on ethical AI practices has been heightened with new policies and frameworks on biases. Harvard Business Review in a recent write up reported that increasingly companies are realizing the necessity of ethical AI.
One substantial framework is the NoBIAS architecture which consists of a Legal and Bias Management Layers. This framework therefore helps organisations to understand, mitigate for, and account for the biases in AI systems. For instance, using AI to power BMW’s smart maintenance system has cut unplanned downtime by 40%. This is done by predicting before they happen what will cause equipment to fail.
Algorithmic fairness techniques are also used as a key aspect. With these techniques, AI systems are then assured the equality in which they will deal with different groups. Audits are regular to find unfair outcomes and to make changes accordingly. For instance, a DataCamp study explains how a variety of data collection, along with algorithmic fairness, is essential for hiring.
Transparency is also crucial. AI systems must reveal what data they are using and how they are making decisions. Basically, this does help in building trust and maintaining accountability. According to Google, the Machine Learning course provides high quality AI education for everyone, free.
FURTHER READING: |
1. AI-Powered Photography: The Next Evolution |
2. AI and IoT: What Every Tech Leader Needs to Know in 2025 |
3. What is Voice Technology? Definition and Examples |
Generative AI and Transfer Learning
Two of the most exciting trends in AI and machine learning for 2025 are generative AI and transfer learning. Generative AI is a form of AI where the models generate new content like images, text and sound. However, transfer learning lets AI models utilize knowledge gained from one task to another in a faster way.
By 2027, 50% of enterprises are expected to deploy AI agents, given that 25% will deploy AI agents by 2025. This shows that generative AI and transfer learning is becoming increasingly crucial in different industries. According to the same report, data center energy consumption will double by 2030 due to the power-intensive nature of generative AI. It also emphasizes sustainable practices for AI.
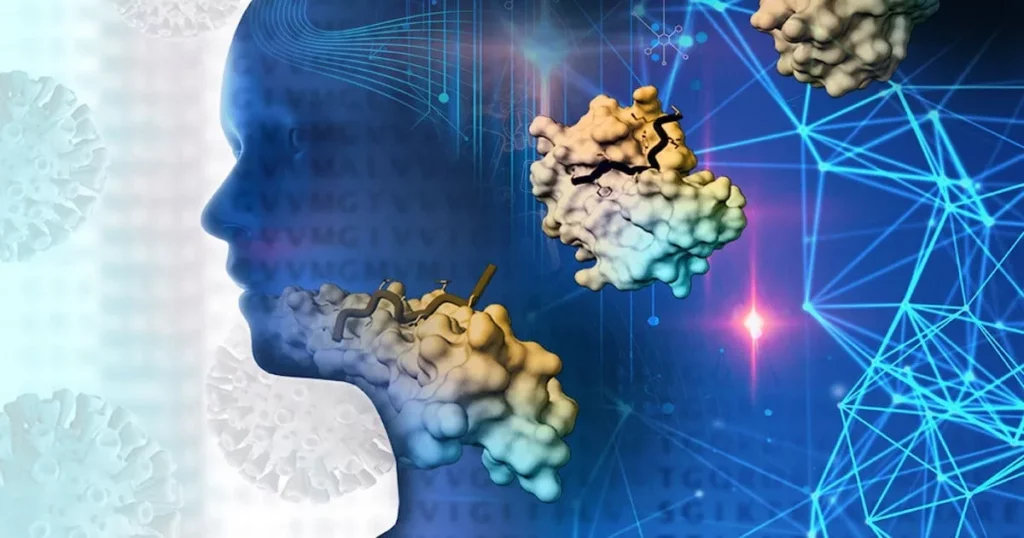
For instance, BMW has taken up smart maintenance systems based on AI, cutting down unplanned downtime by 40%. In this system, the equipment failures are predicted by machine learning before they take place. One more example is Vietnam International Bank, which was able to include a virtual assistant that uses AWS’s generative AI solutions into their digital banking app.
AI as a core part of app design and development is also now a reality. Specifically, AI has become deeply embedded in the systems of coding tools going well beyond simple plugins to AI as the complete environment.
Transfer learning is also a key factor with that, particularly in virtual and augmented reality (VR and AR) technologies. Allowing AI models to transfer knowledge from one context to another securely makes VR and AR environments more realistic and customized.
Role of Generative AI and Transfer Learning
Generative AI and transfer learning are shaping the future of AI and machine learning trends in 2025. A generative AI generates realistic and adaptive content for the virtual and the augmented reality. Transfer learning enables AI models to quickly learn and enhance the knowledge from one task to another. This is to avoid waste of learning resources and time.
According to a DataCamp report, transfer learning is a technique that enhances the model performance. The tricks are tapping into the pre-trained knowledge, minimizing the data required for processing, and more. This is key to creating models such as ChatGPT and Google’s Gemini.
In the area of healthcare, adaptive learning platforms that employ transfer learning are able to personalize patient care. A related concept to this is federated learning which enables decentralized data analysis without losing privacy.
FURTHER READING: |
1. Machine Learning vs AI: Understanding the Key Differences |
2. Future of Machine Learning: Trends & Challenges |
3. NLP and ML in Paraphrasing Tool: How Does It Work? |
Federated Learning in Smart Cities
Federated learning is evolving the smart city by decentralizing data analysis. Processing of data is local to the devices with this approach —data is kept private and bandwidth usage is decreased. By 2025, federated learning will contribute to urban living improvements against data security and resource management efficiency.
A recent report also reveals that federated learning can address privacy concerns in the smart city app. For instance, the federated learning models can predict the usage pattern securely in case of water consumption forecasting. By doing so, people’s privacy is protected and the most efficient allocation of resources is guaranteed.
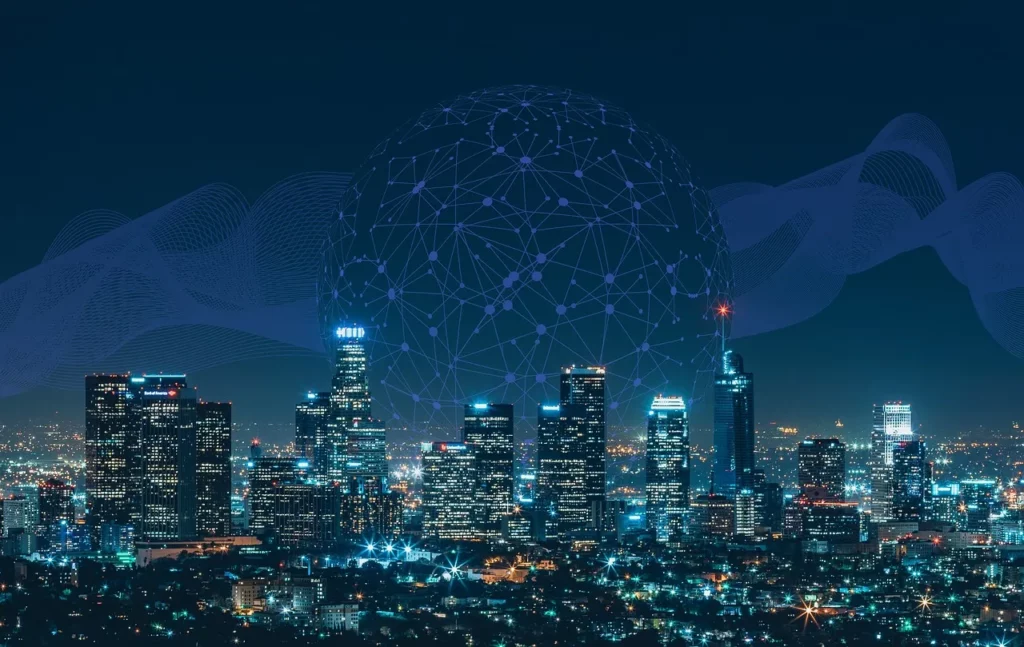
Various services in the smart cities around the world are beginning to adopt federated learning. In Singapore, federated learning is leveraged to improve the public transportation system through analyzing commuter data. It is also used in Barcelona where it is applied to optimize the use of energy in buildings resulting in cost reductiont.
With each city that embraces the benefits of federated learning, smart cities have much potential for the future of technology. If used, this technology will make urban areas more efficient, sustainable, and secure.
Human-AI Collaboration in Urban Environments
Urban environments are rapidly evolving, and AI and machine learning trends are playing a crucial role in this transformation. Among such trends is federated learning, which is slowly but surely changing smart cities. This helps to collaborate model training without raw data being shared and, thereby, we get to preserve privacy and security.
Human AI collaboration in the urban space becomes increasingly relevant, as new stats and information are published. A recent report states that a lot of smart city projects, including fed learning, are now introduced to ensure data privacy while improving the quality of decision-making. With city connections becoming a more integral part of urban life and data gathering becoming many city projects, this trend is likely to grow.
For more detailed insights, you can refer to the comprehensive review on human-computer interaction and human-AI collaboration in advanced air mobility. Opportunities as well as challenges of integrating AI with complex urban environments are also reviewed in this.
Agentic AI
AI and machine learning will be a top trend in the future, for instance, Agentic AI. In other words, AI systems can autonomously plan and act in order to satisfy user defined goals. It is changing the industries with more flexible and efficient solutions.
Healthcare is one such sector that can serve as one of the major drivers of agentic AI. Over subsequent five years, the global agentic AI in the healthcare market is anticipated to witness a CAGR of 35-40%. The need for personalized healthcare combined with AI in diagnostics is fueling this growth. For instance, examples of AI adoption that saw an increase of 28% in 2023 include AI patient monitoring systems.
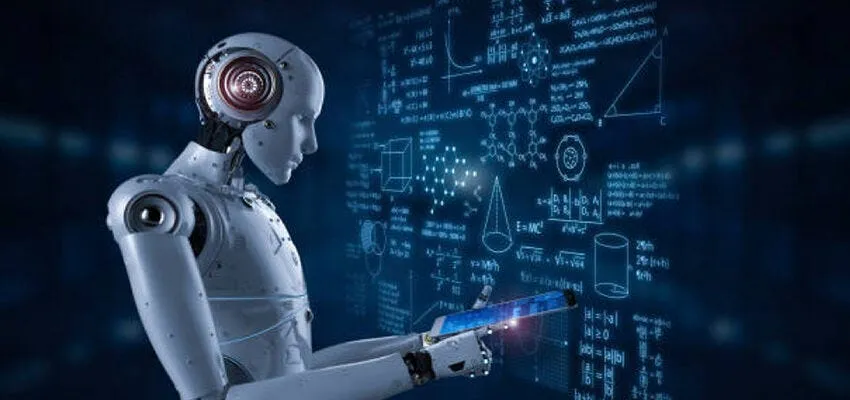
Agentic AI is forecasted to hit USD 80.9 billion in the financial services by 2034. AI Agents are being employed by financial institutions to provide better workflows and customer support. This is because of the need to become more efficient and accurate in financial operations.
Overall, there is no doubt that agentic AI can bring revolution to so many industries with autonomous and intelligent solutions. Furthermore, this trend shows its importance for the companies to invest in AI technologies and secure their competitive edge and growth.
Rise of Autonomous AI Agents
Autonomous AI agents are one of the most exciting trends in AI and machine learning in 2025. These intelligent systems can autonomously function, making decisions and performing tasks without any human control. As per a report by Sellers Commerce, the market for the AI agent will expand from $7.38 billion in 2023 to $47 billion by 2030. The rapid growth here shows that autonomous AI agents get more and more important in different industries.
Presently, autonomous AI agents is used for a variety of applications in finance, cybersecurity, customer service and healthcare. For instance, Microsoft Copilot and Salesforce Agentforce are including these agents into their platform to help make employees more productive and the whole business more streamlined. Then, these agents are able to take care of regular things like taking care of customer service inquiries so the men and women intended to work in such jobs are able to focus on more complicated problems.
The International Telecommunication Union (ITU) is additionally focused on the increase of autonomy of AI agents at their AI for Good Global Summit in 2025. At the summit, the responsible and safe use of these agents will be investigated and how the agents can contribute to a better society as a whole. It will gather experts from different domains to explore problems and opportunities of such autonomous AI agents.
Edge Machine Learning
In 2025, Edge Machine Learning is becoming a lucrative ground. This comes from a trend of deploying machine learning models directly on the device they are located on, i.e., at the data source which reduces the latency and allows for real time processing. By 2025, 75% of enterprise-managed data will be created and processed outside traditional data centers, as is reported by Gartner. This is a very important shift for industries that are built on a time sensitive basis such as healthcare, finance or autonomous vehicles.
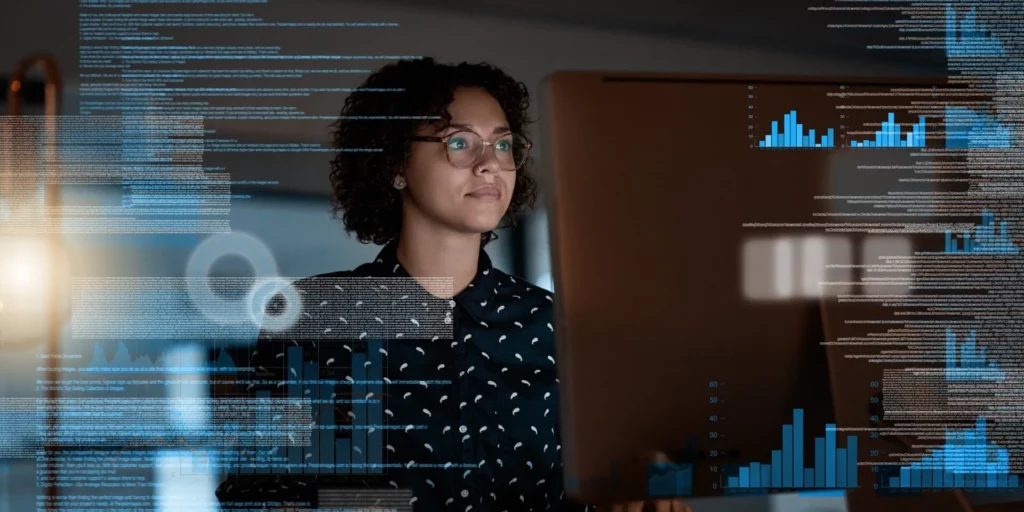
The use of edge ML is not strictly for designated industries. Statista reports also note that the machine learning market is projected to reach $113.10 billion by 2025, where edge computing will have a major part. It is democratizing the access to advanced AI technologies and it is making it possible for small businesses, who otherwise could not have access to machine learning, experience this technology for better operational efficiency and innovation.
Impact on Industries like Manufacturing and Healthcare
The industries such as manufacturing or healthcare, are transforming due to edge machine learning. In the manufacturing world, edge AI enables the optimization of production processes, decrease of downtime as well as increased quality management.
Presently, edge machine learning enables real time patient monitoring, predictive diagnosis, etc. in healthcare. Machine learning algorithms on wearable devices can identify anomalies in vital signs before it can turn into dystocia and provide early intervention for a better outcome in dystocia, in real-time. A research article published promotes the use of edge computing and wearable devices in health care and emphasizes the progress in fall detection, cardiovascular monitoring applications, as well as in disease prediction.
Reports of Edge Impulse and MDPI describe how these benefits and challenges manifest in the integration of edge AI in industrial and healthcare setups. The use of edge machine learning is only going to get more efficient, hone patient outcomes, and completely change the way things operate in industry.
Conclusion
As we wrap up this article on “5 AI and Machine Learning Trends to Watch in 2025,” it’s clear that the landscape is rapidly evolving. Here at Designveloper, we are among those at the tipping point of these advancements as we strive to deliver such services. We offer reliable services ranging from Generative AI to AI chatbot integration that will help you use AI to enhance innovation, efficiency and credibility.
With ethical AI practices and federated learning, the possibility of change is enormous. As such, we are thrilled to keep leading in AI development and to empower our clients to continue to be out in front.
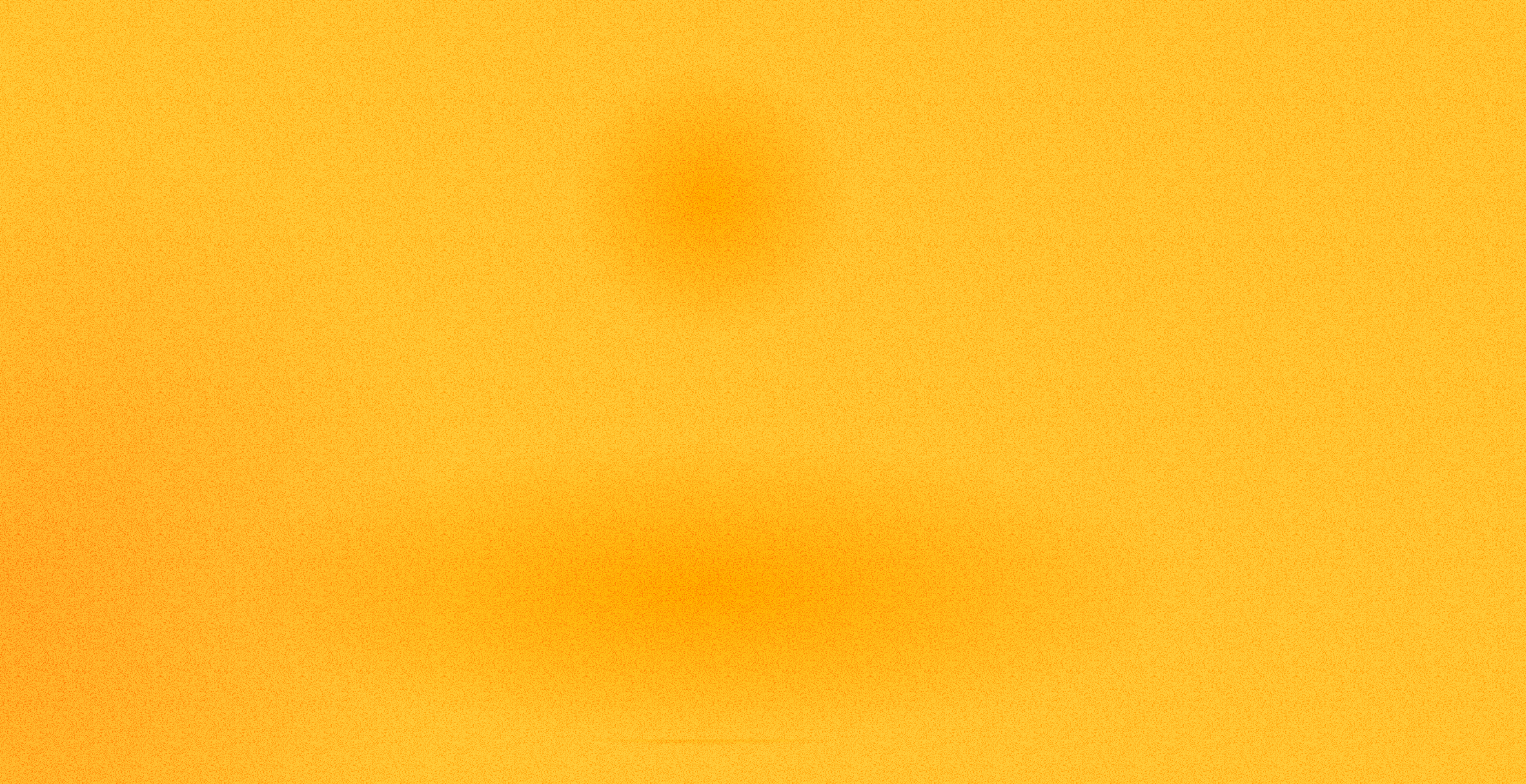
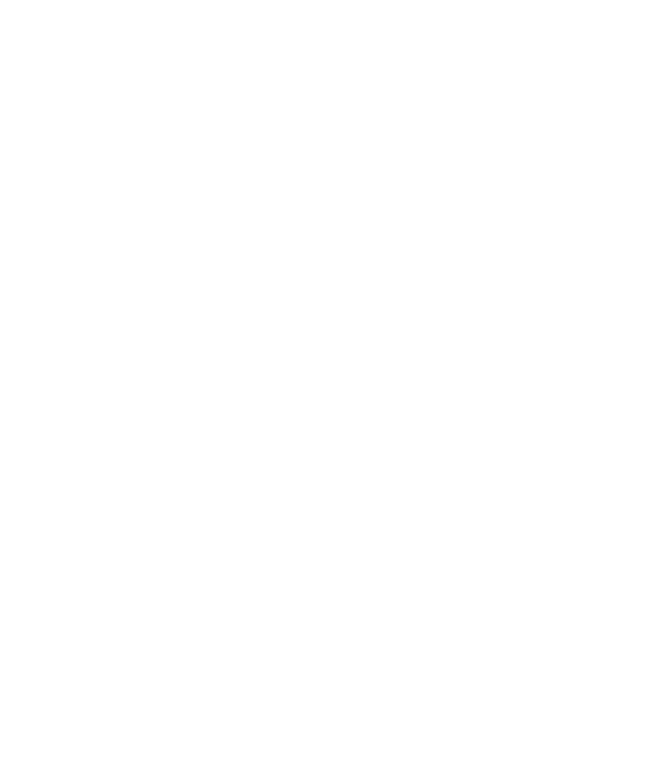
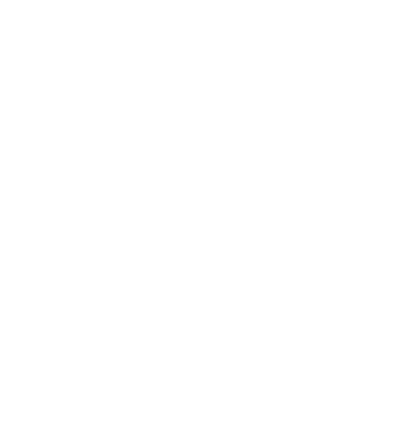
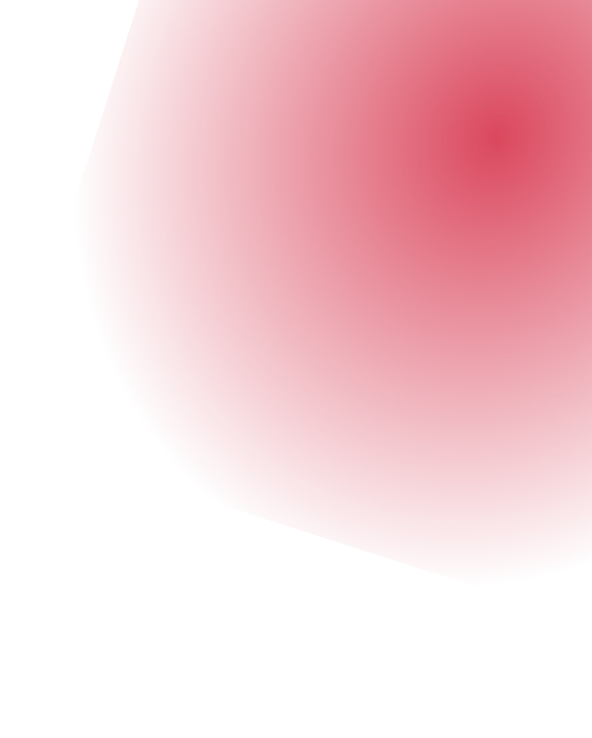
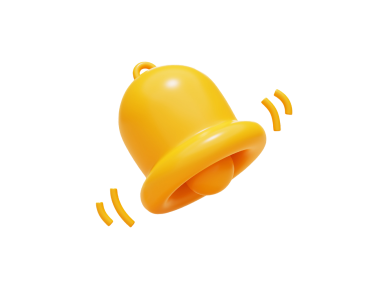
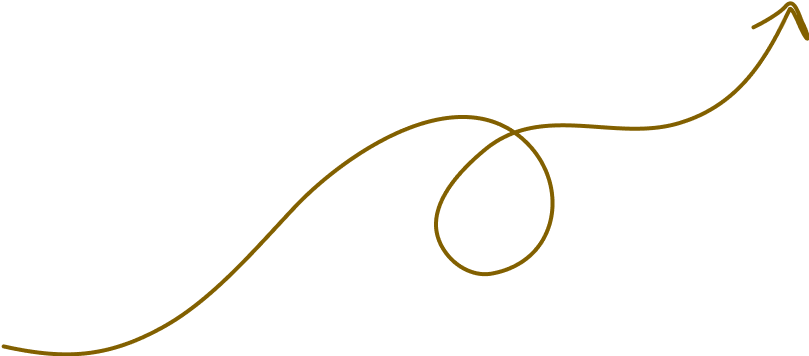
Read more topics
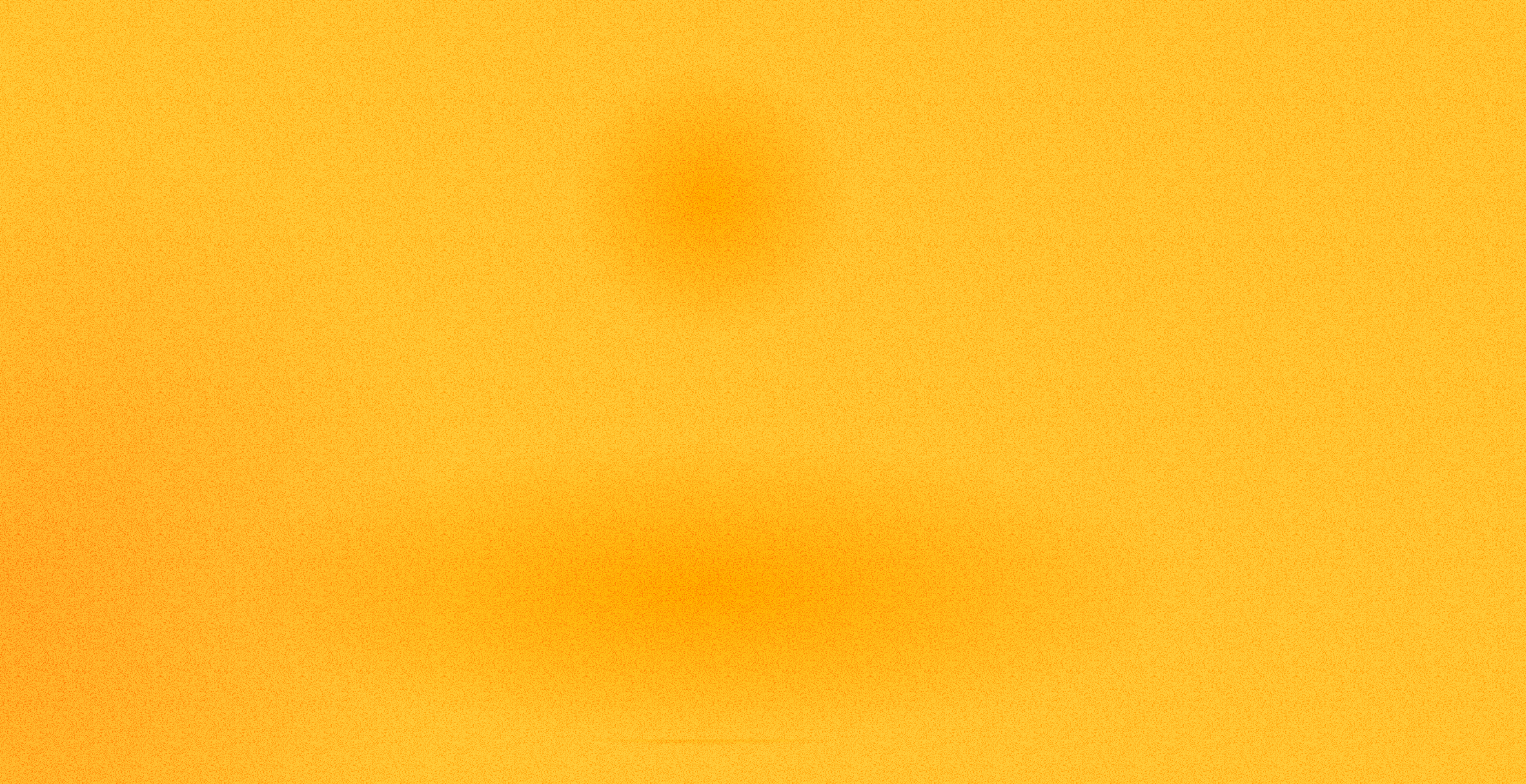
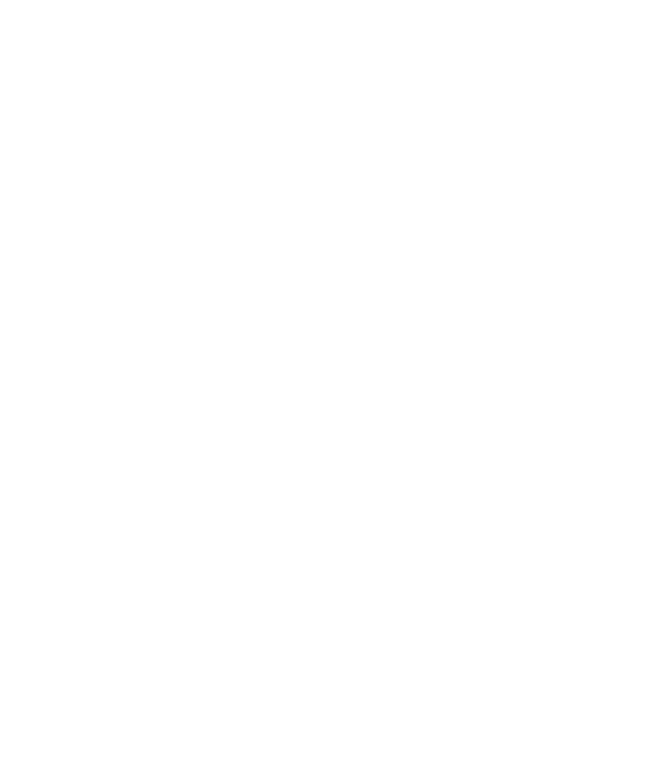
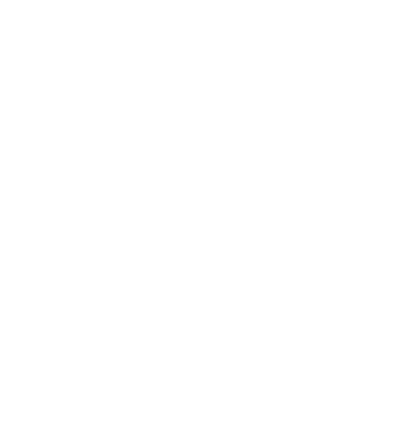
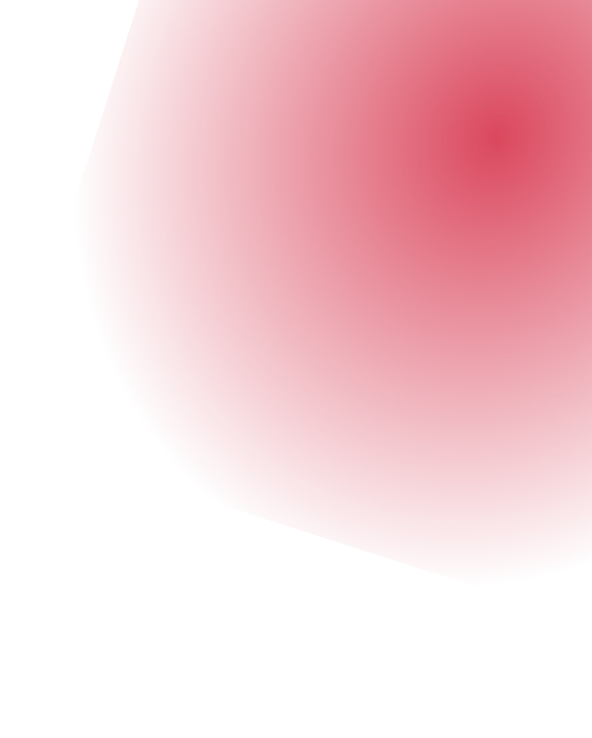
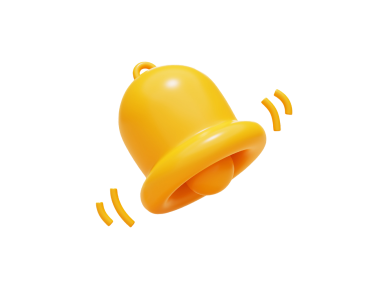
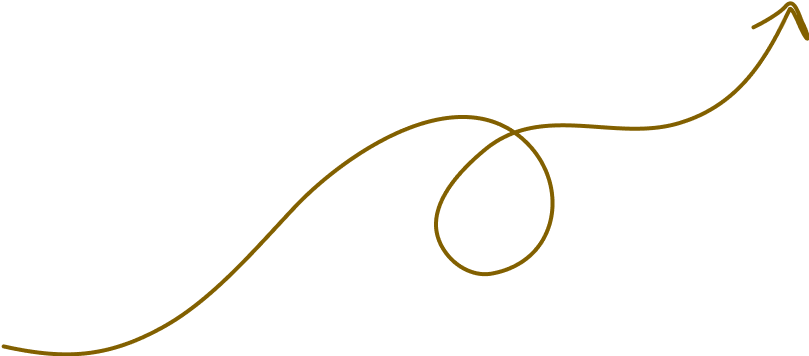