In today’s business world, decisions are no longer made in boardrooms filled with hunches and gut feelings. The key to success lies in data driven decision making (DDDM), where cold, hard facts guide your every move. With the power of data, you know exactly what your customers want, how to optimize your marketing, and where to invest for maximum growth.
But how do you unlock this power? You’re a busy leader, not a data scientist. Fear not! This guide is your roadmap to DDDM mastery.
In this guide, Designveloper (DSV) will break down the 7 simple steps to implement DDDM, along with real-world examples of companies applying this approach. We’ll even show you how technology can be your secret weapon, with insights from app-making experts at DSV.
But beforehand, let’s take a quick look at what DDDM means and how it benefits your business.
What is Data Driven Decision Making?
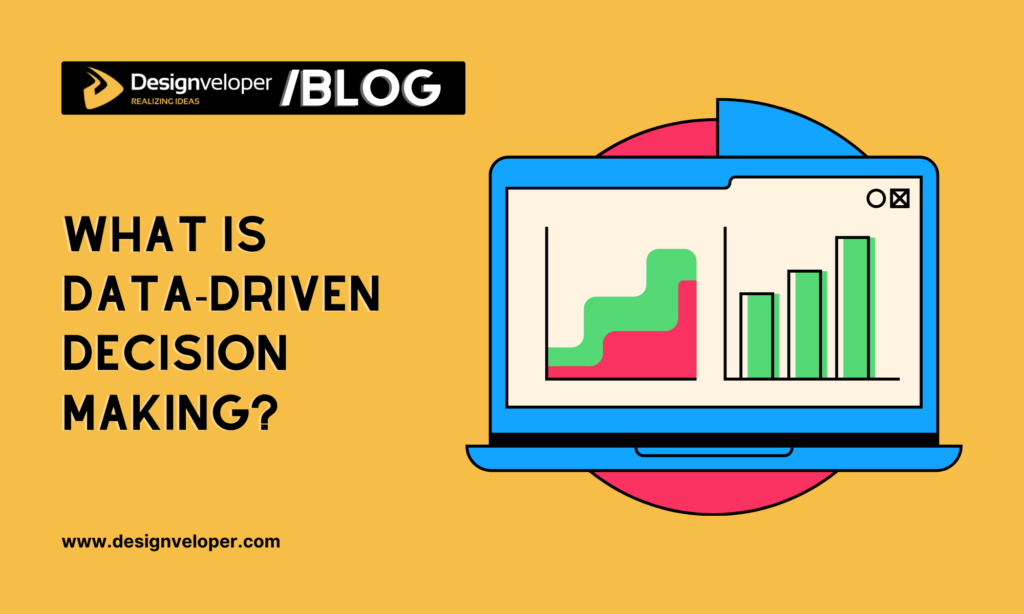
Data driven decision making (DDDM) is a process where decisions are based on actual data rather than intuition or observation alone. It involves leveraging measurable data and insights to make informed choices that align with your business goals.
For example, your company might collect survey responses to identify products your customers would like, or analyze demographic data to determine business opportunities.
The shift from traditional decision-making processes to data-driven approaches is no passing fad. In the past, those involved in business strategy or management roles often made decisions based on personal experience or intuition. However, with the advent of new technologies and the increasing availability of data, more and more businesses are turning to data to inform their decisions.
Statista indicated a 12% increase in implementing DDDM within global organizations from 2018 to 2020.
Why the surge? Because data unveils hidden trends, customer preferences, and market opportunities that intuition simply can’t. By leveraging data, businesses can gain valuable insights, leading to better outcomes and increased success.
How Data Driven Decision Making Benefits Your Business
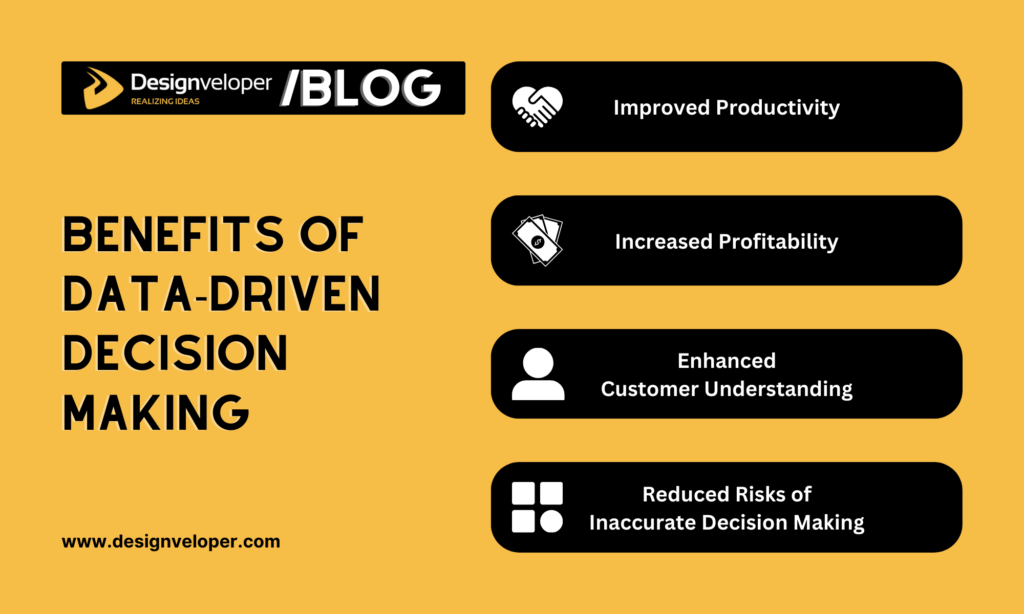
To further understand why DDDM is that important to your business, let’s delve into the numerous benefits it offers.
Improved Efficiency and Productivity
According to a Harvard Business Review (HBR) study for Google Cloud, data and AI helped companies achieve 81% efficiency in their operations, higher than those who don’t (58%).
Why? By analyzing data, companies can identify patterns, trends, and insights that can help streamline processes, reduce waste, and optimize resource allocation. Accordingly, they can boost overall productivity and business efficiency.
Increased Profitability
Data plays a crucial role in increasing revenue. The HBR research also found that companies with strong data analytics capabilities enjoyed 16% higher profitability than their competitors
But how? Data unveils hidden sales opportunities, like untapped customer segments or underperforming marketing channels. Besides, it allows your business to understand your customers and identify market trends better. Accordingly, you can inform marketing, pricing, and product development decisions that can unlock new avenues for growth and profit.
Enhanced Customer Understanding
Data-driven companies can obtain 77% customer loyalty or retention, almost doubling those who don’t.
By analyzing customer data, companies can gain insights into customer behaviors, buying habits, and lifestyle preferences. This information can be used to personalize customer experiences, develop products and services that meet customer needs, and improve customer satisfaction.
Reduced Risks of Inaccurate Decision Making
Intuition has its place, but for critical business decisions, solid data is your shield against costly mistakes.
Using data helps you reduce the reliance on assumptions and guesswork when evaluating your current business performance and predicting future trends.
Based on factual, reliable, and up-to-date information, you make more accurate decisions with confidence and avoid unexpected risks.
FURTHER READING: |
1. How to Increase the Quality of Your Data |
2. What Is Data Classification: Best Practices And Data Types |
3. What Is Big Data Analytics and How It Useful for Business? |
7 Steps of Data Driven Decision Making
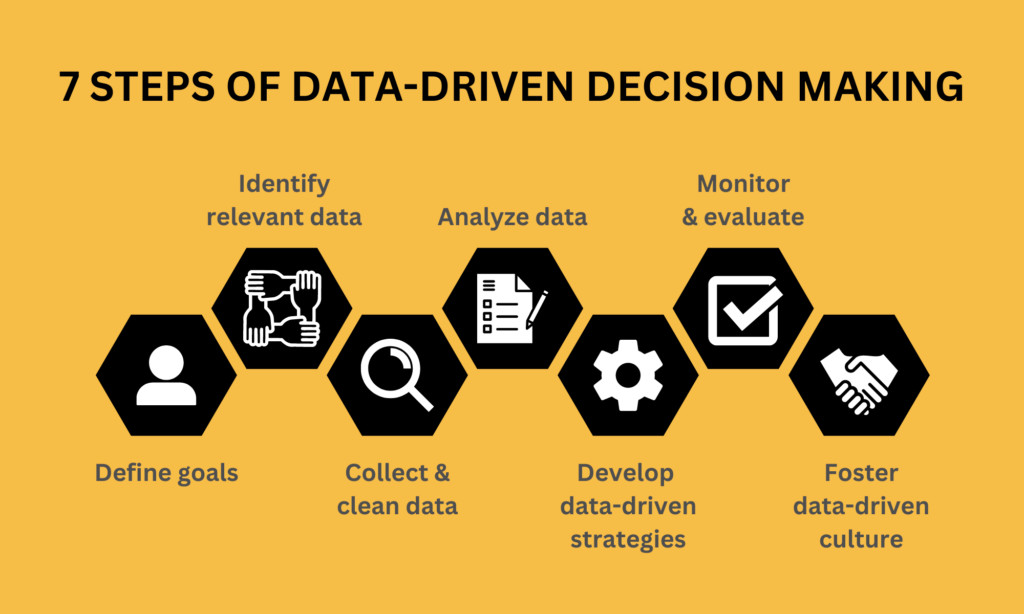
You understand what data driven decision making means, coupled with its outstanding benefits. And now, understanding how to effectively harness its power isn’t less crucial. This section, therefore, provides 7 simple steps to make data-driven decisions:
Step 1: Define Goals and Objectives
The first step is to clearly define your goals and objectives. What exactly do you want to achieve with data driven decision making (DDDM)? This could range from improving business efficiency and increasing customer satisfaction to predicting future trends.
Your goals should be SMART – Specific, Measurable, Achievable, Relevant, and Time-bound. This means they should be well-defined, quantifiable, realistic, aligned with your strategic direction, and have a specific timeline for achievement.
By setting clear goals and objectives, you establish a direction for your DDDM journey. This step is crucial as it sets the foundation for the subsequent steps and helps ensure that your efforts are aligned with your desired outcomes.
Step 2: Identify Relevant Data
Not all data is necessary for your business goals. So, it’s essential to determine what kind of data you need. Then, identify where it can be obtained from and how it can be collected.
The data you identify should be relevant and directly contribute to the achievement of your goals. For instance, if your goal is to improve customer satisfaction, relevant data might include customer feedback, purchase history, and customer support interactions.
Remember, not all data is created equal. The relevance of data is more important than its volume. It’s not about having a large amount of data; it’s about having the right data.
In this step, you might also need to consider any potential ethical, legal, and privacy issues related to data collection and usage. Always ensure that your data practices comply with all relevant regulations and respect user privacy.
Step 3: Collect and Clean Data
After identifying the relevant data, the next step is to collect it. Data can be gathered from a variety of sources such as databases, APIs, web scraping, surveys, or even manually entered. It’s important to ensure that the data collection methods are reliable and consistent to maintain the integrity of your data.
After data collection, you need to clean, transform, and format it to prepare for seamless analysis. Data cleaning involves checking for and handling missing values, removing duplicates, correcting inconsistent entries, and dealing with outliers. This step is crucial as the quality of your data directly impacts the accuracy of your insights.
Step 4: Analyze and Interpret Data
With clean and relevant data in hand, the next step is to analyze and interpret it. This involves using statistical methods, machine learning algorithms, or data visualization tools to extract meaningful insights from your data.
The choice of analysis method depends on your goals and the nature of your data. For example, you might use regression analysis to understand the relationship between variables, clustering to group similar data points, or sentiment analysis to gauge customer sentiment.
Interpreting the results correctly is just as important as the analysis itself. Be mindful of potential biases and ensure that your interpretations align with your initial goals and objectives.
Step 5: Develop Data-Driven Strategies
Next, you need to translate the insights gained from your data analysis into actionable strategies. This involves using the knowledge you’ve gained to inform your decision-making and develop plans that align with your goals and objectives.
For example, if your analysis reveals that customers are most satisfied with your product’s ease of use, you might focus your strategy on further simplifying your product. Alternatively, if your data shows a strong correlation between marketing spend and sales, you might decide to increase your marketing budget.
Step 6: Monitor and Evaluate
After implementing your data-driven strategies, it’s important to monitor progress and evaluate the effectiveness of your decisions. This involves tracking key performance indicators (KPIs) that align with your goals and objectives, and assessing whether your actions are leading to the desired outcomes.
Monitoring should be an ongoing process. As you collect more data over time, you’ll gain a better understanding of trends, patterns, and the impact of your decisions. If your strategies are not yielding the expected results, don’t be afraid to adjust them.
Step 7: Foster a Data-Driven Culture
The final step, and perhaps the most challenging, is fostering a data-driven culture within your organization.
The 2022 Report by Deloitte indicated that 50% of chief data officers (CDOs) considered developing data culture within their organizations as one of their top priorities. However, 54% of CDOs said data literacy among employees was challenging their organizations.
Therefore, to effectively build a data-driven culture, it’s essential to promote data literacy, encourage data driven decision making at all levels, and ensure that everyone understands the value of data.
Fostering a data-driven culture is not just about having the right tools or processes in place. It’s about changing mindsets and attitudes towards data. It’s about creating an environment where data is not just seen as numbers or charts, but as a valuable resource that can guide decision-making and drive innovation.
By fostering a data-driven culture, you’re not just improving your own decision-making abilities, but empowering everyone in your organization to make better, more informed decisions.
4 Real-World Examples of Data Driven Decision Making
While understanding the steps of DDDM is crucial, seeing how these steps are applied in real-world scenarios can provide invaluable insights.
In this section, DSV will present examples of companies that have successfully implemented DDDM. These inspiring examples offer lessons that you can apply in your own DDDM journey.
Netflix
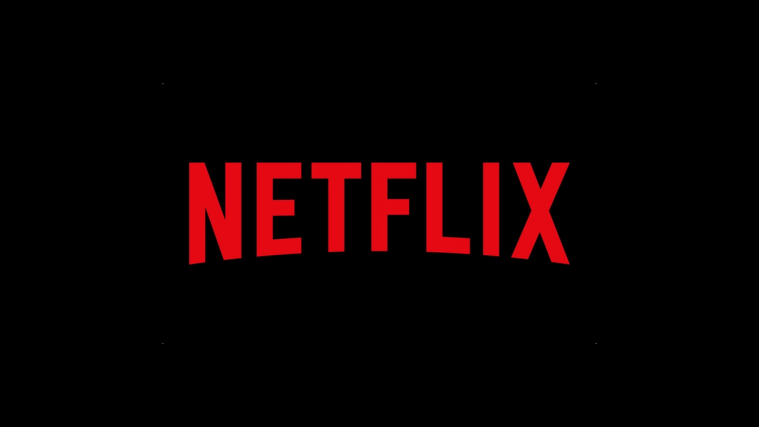
Netflix, a leading streaming service, has successfully made data-based decisions to achieve remarkable results. Here’s how:
Data in Every Business Facet
The Netflix team delves deeply into personalized customer data, using it to determine even cover designs for new original content based on their impact on subscriber viewing habits, recommendations, ratings, and more.
They leverage advanced machine learning to personalize everything, offering improved recommendations and informing their upcoming content.
Having access to such comprehensive data, from both existing customers and market reactions to competitors, enables the Netflix team to pose more insightful questions and make informed decisions.
They no longer rely on smaller focus groups or other traditional forms of testing during the production stage, but instead, depend on extensive data to guide every decision, regardless of its magnitude.
Data-Driven Marketing
Netflix also utilizes external data sources like social media to decide where to concentrate their marketing efforts.
For instance, when the latest season of Gilmore Girls was launched in 2016, they tested the audience’s interest with a few tweets. Seeing the viral response, they capitalized on this enthusiasm, even resorting to offline tactics like recreating the iconic Luke’s Diner in select cities to maximize the season’s release.
McDonald’s
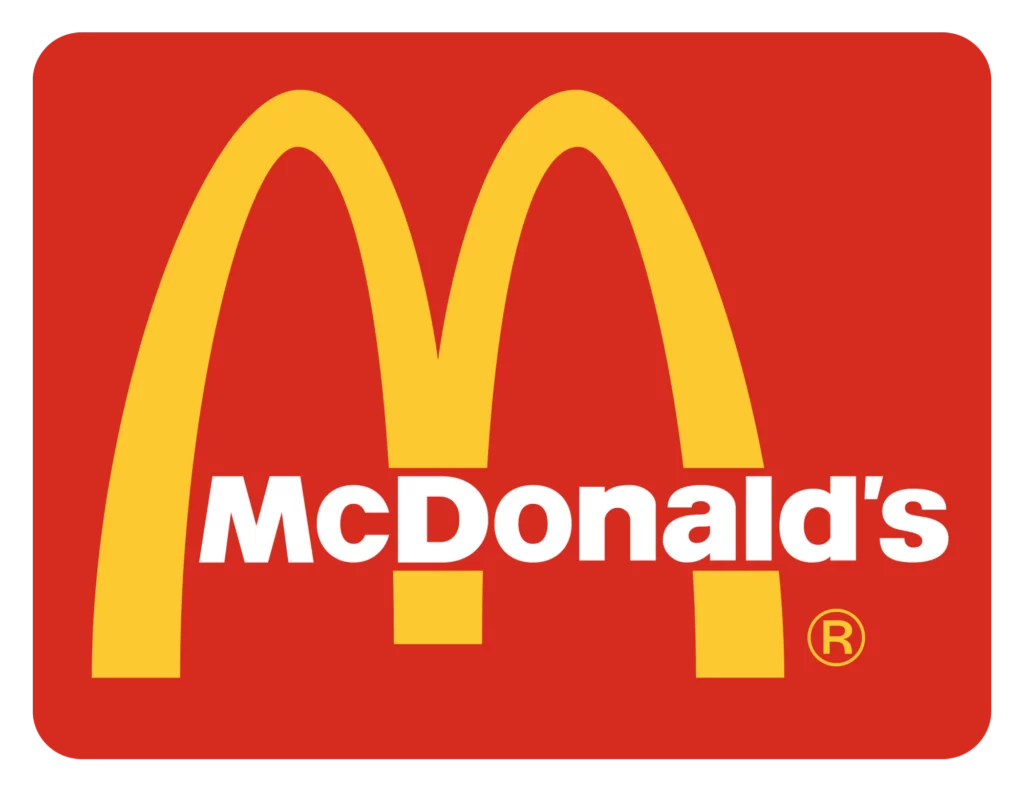
To stand out in the highly competitive fast-food market, McDonald’s has built a scalable environment that helps manage its global data, perform daily executive reports, and provide data analytics about everything from menus to the supply chain.
This robust, scalable environment used Amazon Redshift as a cloud data warehouse, Talend for data integration, and Tableau for data visualization. Accordingly, it benefits McDonald’s in the following aspects:
Understanding Customer Preferences
McDonald’s invested in big data to better understand its customers. By analyzing data across all their stores and creating data visualizations, they were able to gain a more holistic view of their customers. Also, such data allows McDonald’s to send personalized discounts to target customers based on their interests.
Optimizing Operations
McDonald’s used kitchen data to perfect cooking methods for taste and temperature, and drive-thru data was leveraged to restructure the flow for faster completion. They also used in-store data to make new iterations in the layout and design of the store.
Enhancing Delivery Services
McDonald’s collected and analyzed key performance indicators (KPIs) such as customer satisfaction, average check sales, guest counts, and total delivery time to measure the growth and performance of restaurants on deliveries made in partnership with third-party delivery services.
Coca-Cola
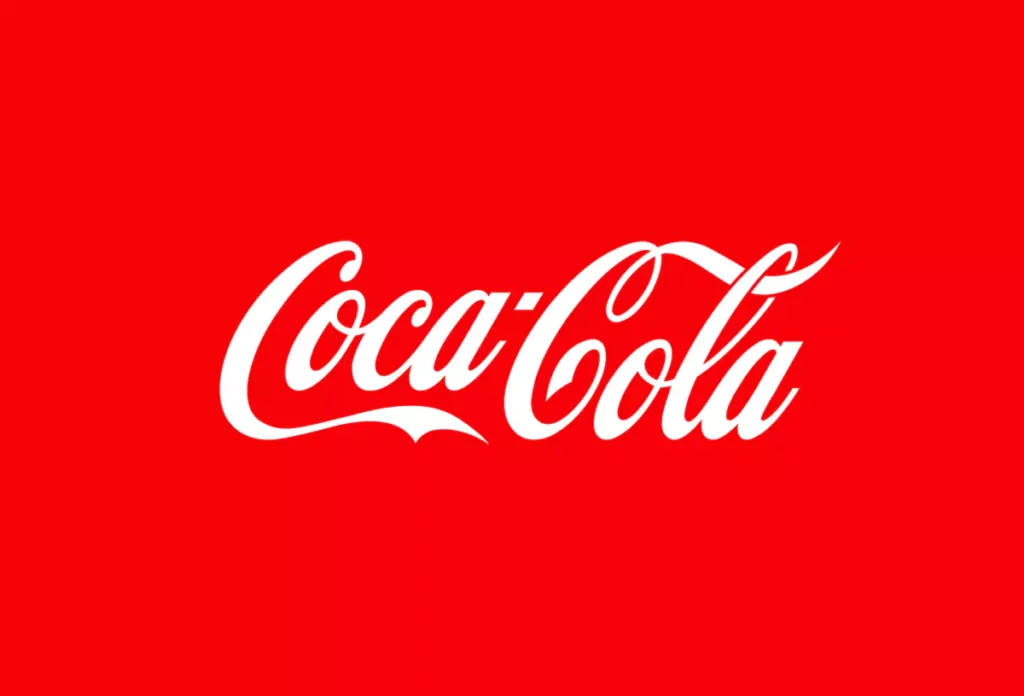
Coca-Cola is the world’s largest beverage company. As one of the first globally recognized brands using Big Data and AI, Coca-Cola has provided customers with intelligent, tailored experiences.
Here are some specific ways Coca-Cola has used data:
Understanding Consumer Preferences
Coca-Cola has strategically invested in advanced techs, especially AI, to harness the vast data from their global customer base. This investment provides the company with a deeper understanding of consumer trends, particularly in terms of flavor preferences and healthier choices in specific regions.
Innovative Product Development
In 2008, Coca-Cola revolutionized the beverage industry with the introduction of their Freestyle fountain drink dispenser. This innovative device, equipped with an interactive touch-screen display, offered customers the freedom to mix and match beverages and flavors from Coca-Cola’s extensive portfolio.
The data collected from these unique combinations gave Coca-Cola invaluable insights into consumer preferences, effectively enabling them to crowdsource new product ideas. A testament to this strategy’s success is the creation of Cherry Sprite, a product directly inspired by popular combinations identified through Freestyle dispensers.
Fast forward to 2018, Coca-Cola further enhanced their data-driven approach by integrating artificial intelligence (AI), machine learning (ML), and real-time analytics. With the advent of mobile apps, customers can now pre-order their drinks, providing Coca-Cola’s data scientists with a wealth of information to track and analyze long-term consumer behavior in unprecedented detail.
Social Data Harnessing
By allowing customers to register for the app via their social media accounts, Coca-Cola has gained access to a treasure trove of social media activity.
These insights empower Coca-Cola to craft personalized marketing campaigns and customer experiences, taking their customer engagement to new heights.
DBS Bank
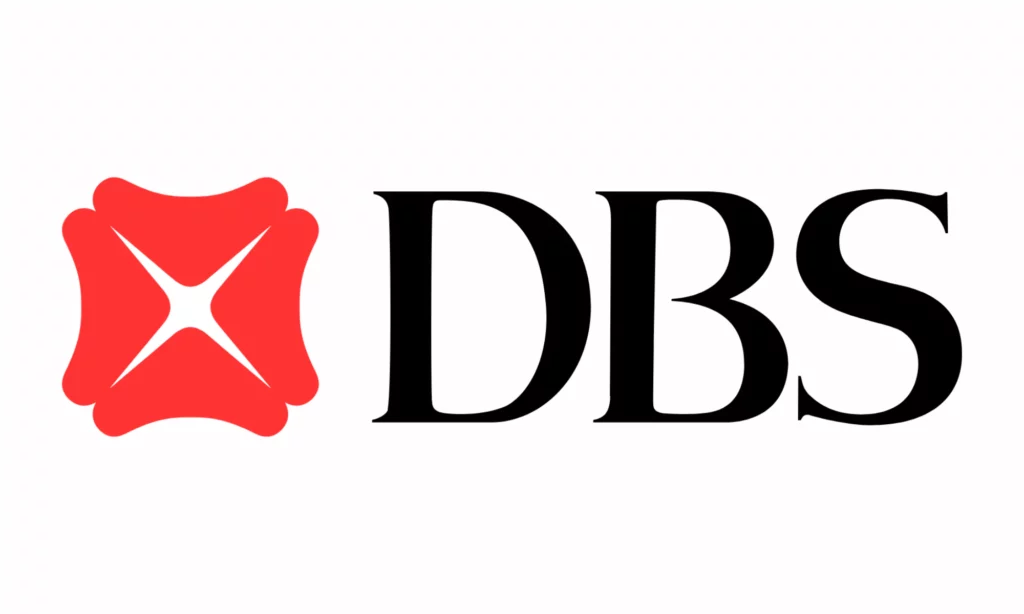
DBS is the largest bank in Southeast Asia headquartered in Singapore. Recognizing the higher demand of their tech-savvy customers, DBS Bank re-debuted as a tech start-up in banking. Here’s how it’s used data to stay competitive in this rapidly changing digital era:
Data-Driven Organization
Partnering with McKinsey, DBS Bank has changed its data-driven operating model. The bank takes smart decisions based on data and not instincts. They built a central data team and enterprise data hub, enabling DBS to scale out more economically and experiment more.
To change the whole company’s mindset of responsibly using data, the leaders enabled their employees to think of data first. Then, provide them with the capabilities and tools to use data and analytics effectively while making decisions.
Fraud Detection
DBS Bank used data to find out where fraud is happening in the company. For instance, they started looking at data other than invoices and transactions to predict the possibility of fraud.
Employee Skill Development
DBS Bank equipped its employees with skills to become every day “Data Heroes”. They created network analysis models to identify shell companies within money-laundering networks, used big data to halve attrition rates of new-to-bank wealth planning managers, and developed a system to identify abnormal transactions while tackling trade finance mandates.
Also, by combining data analytics with AI, DBS Bank puts end-to-end money laundering under surveillance and supports HR in employee retention.
The Power of Tech in Data Driven Decision Making: DSV’s Insights
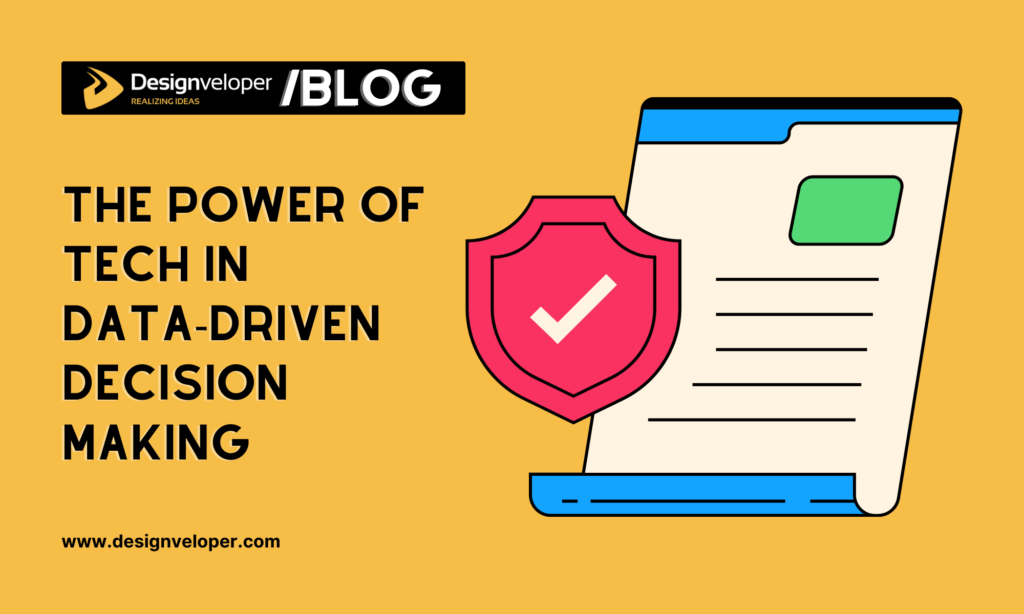
We’ve explored the transformative power of data driven decision making. But let’s be honest, harnessing data effectively can feel overwhelming. That’s where technology steps in as your secret weapon.
As Designveloper, we’ve built our app-making expertise on the foundation of DDDM. We understand that raw data is just potential; it’s the technology and methods that unlock its true value. So, how can we leverage techs to harness data for our app-making decisions? Let’s explore:
Market Research
Understanding current customer demands, market trends, and competitors is a foundation for implementing essential features for apps or software.
DSV, therefore, leverages technology to conduct comprehensive market research. We use advanced data analytics tools to gather and analyze data from various sources, including social media, online forums, and customer feedback. This data is then processed and analyzed to identify market trends, customer preferences, and potential opportunities.
Technology plays a crucial role in this process. It allows us to handle large volumes of data and derive meaningful insights from it. These insights help us understand the market better and make informed decisions about product features, target audience, and marketing strategies.
Testing
We use technology to implement A/B testing and multivariate testing. These methods allow us to test different versions of our product with different groups of users and gather data on their responses. The insights gained from these tests are then used to make data-driven decisions about product updates and improvements.
Post-Release
Once the product is released, techs continue to play a vital role in data driven decision making. DSV provides our clients with data analytics and reporting capabilities built in apps/software to collect user feedback and monitor product performance.
As such, our clients can track various metrics (like user engagement, retention rate, and customer satisfaction) to understand how the product works and how users engage in their apps.
Accordingly, clients can validate whether features resonate with their users, spot underperforming areas, and make adjustments based on data.
Final Words
The future of the business world is data-driven. Accordingly, companies will make informed decisions based on reliable, essential data. With the support of advanced techs (like AI/ML or interactive dashboards), your data analytics and reporting become much easier.
Through this guide, we equipped you with the basics of data driven decision making, from steps to implement DDDM in your business and inspiring examples to how techs can be your secret weapon in harnessing the power of data.
Now, it’s your turn to take the reins. Step out of the shadows of uncertainty and into the light of data-driven clarity. Wisely implement data driven decision making in your organization today and witness the transformation.
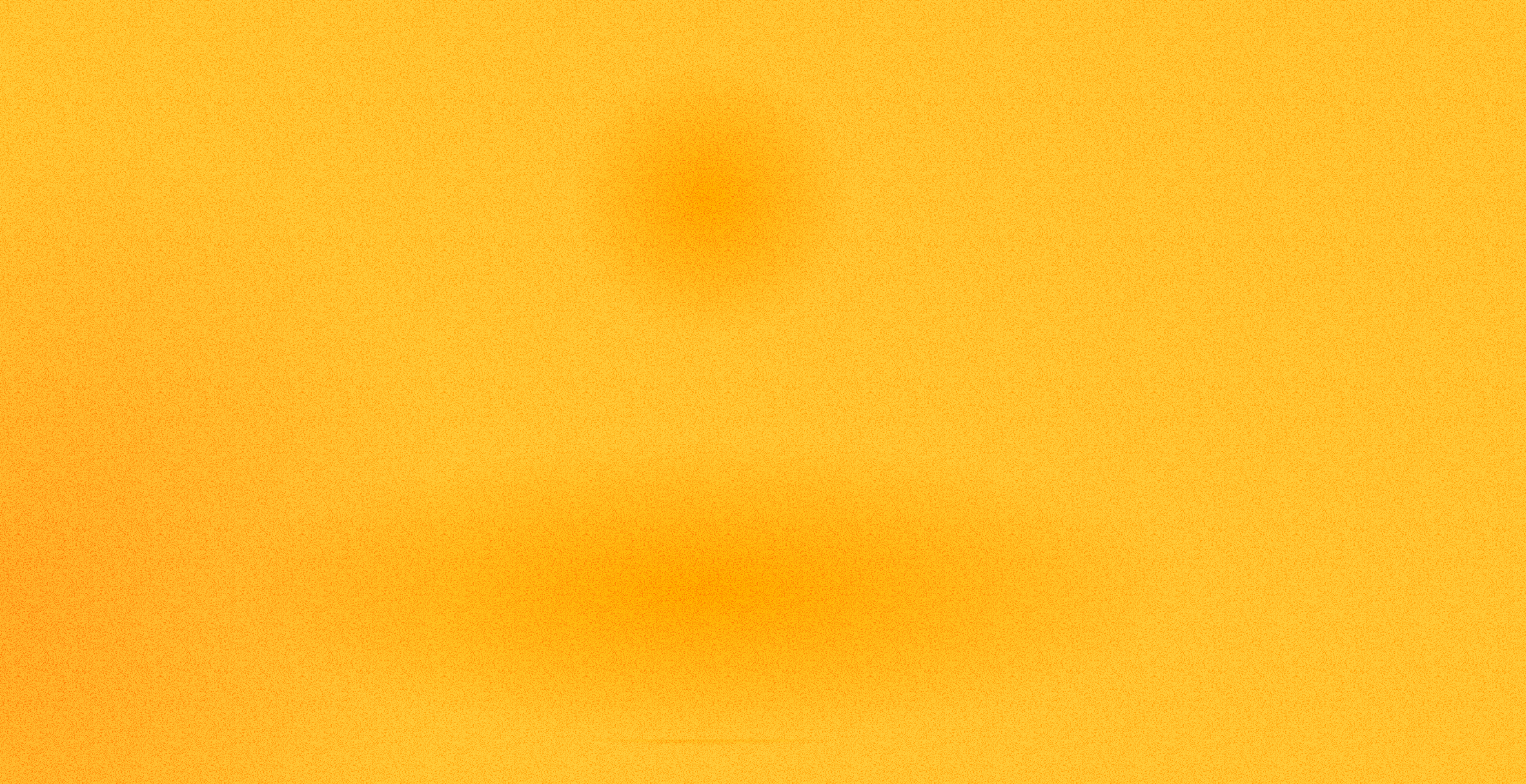
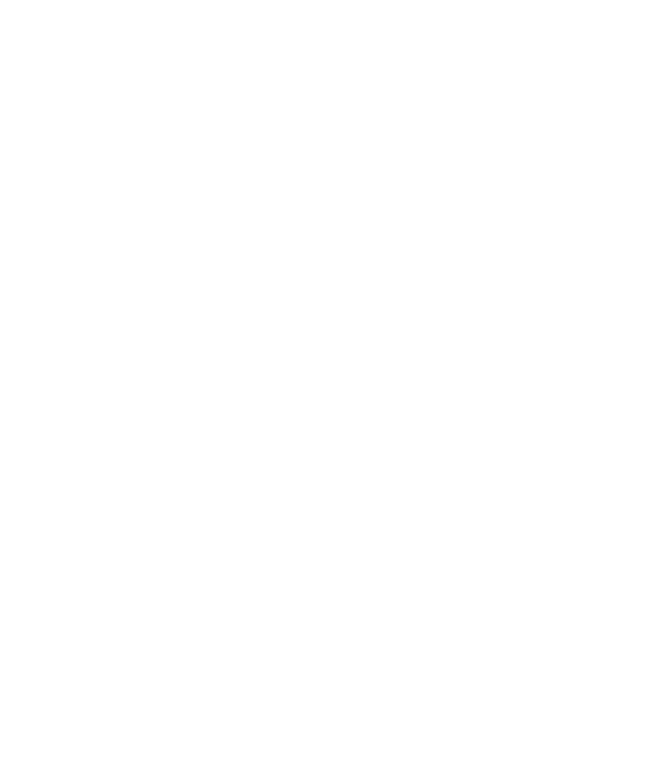
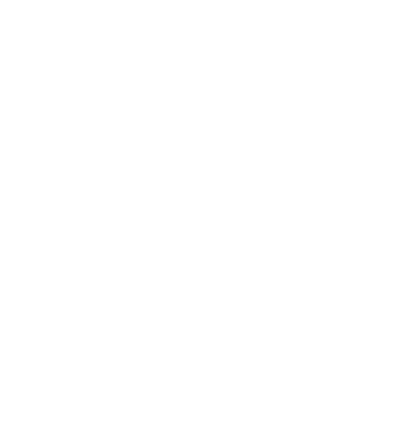
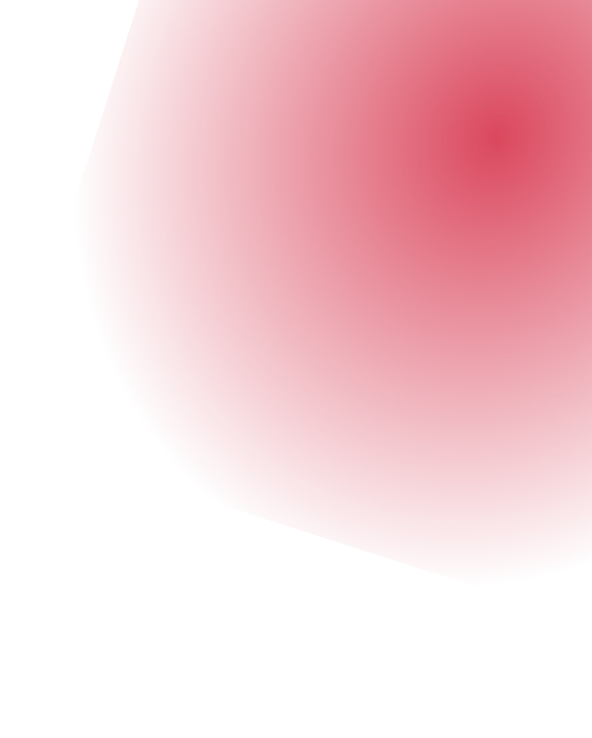
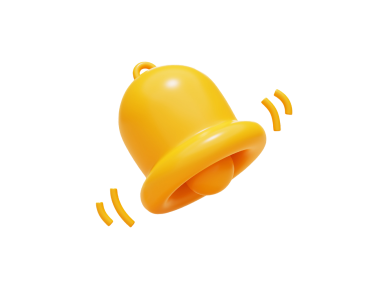
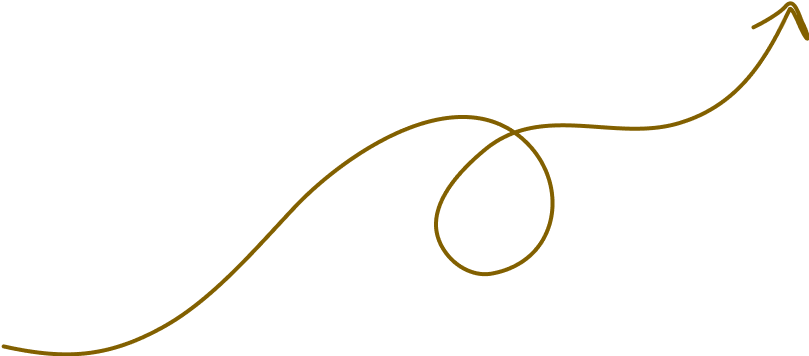
Read more topics
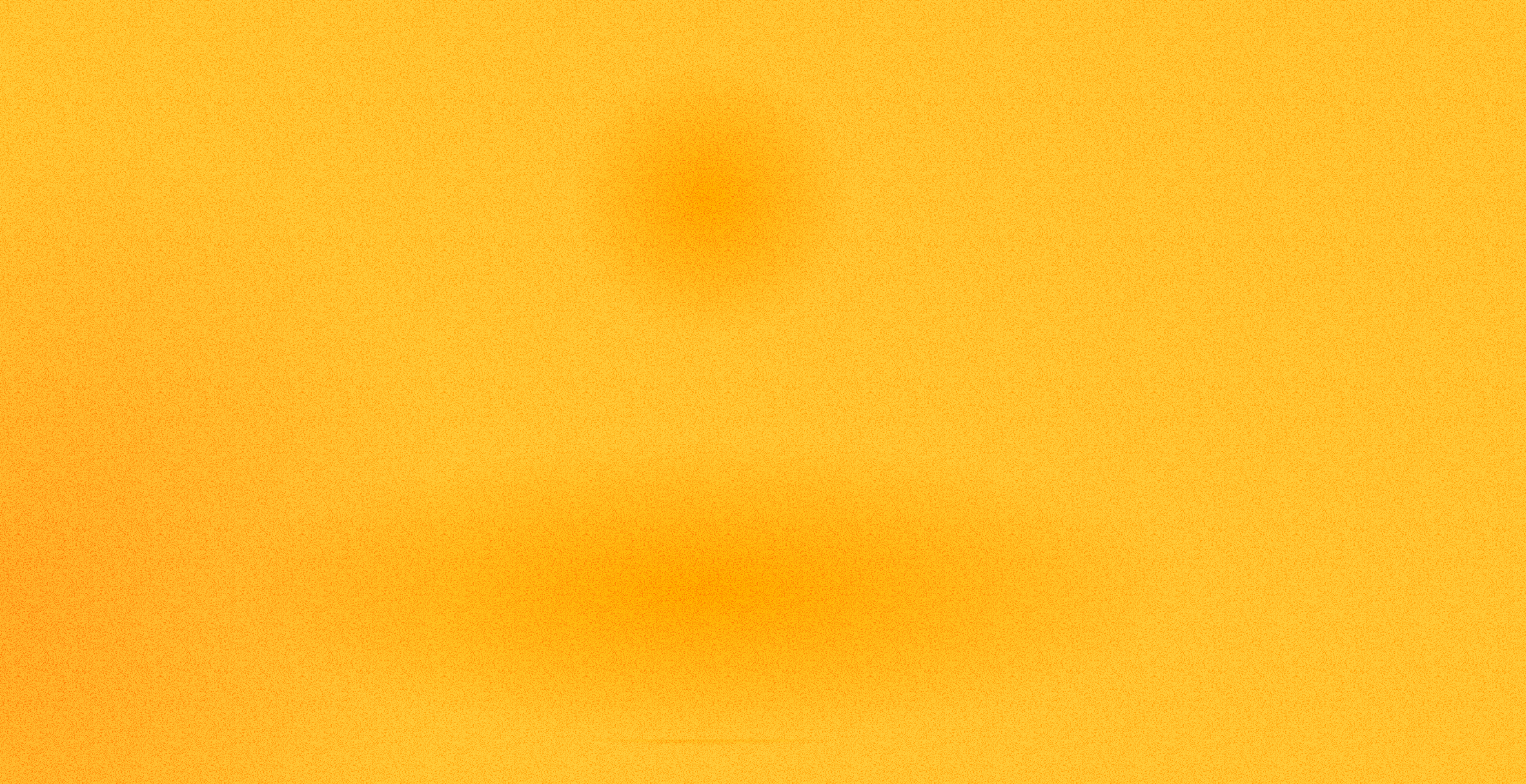
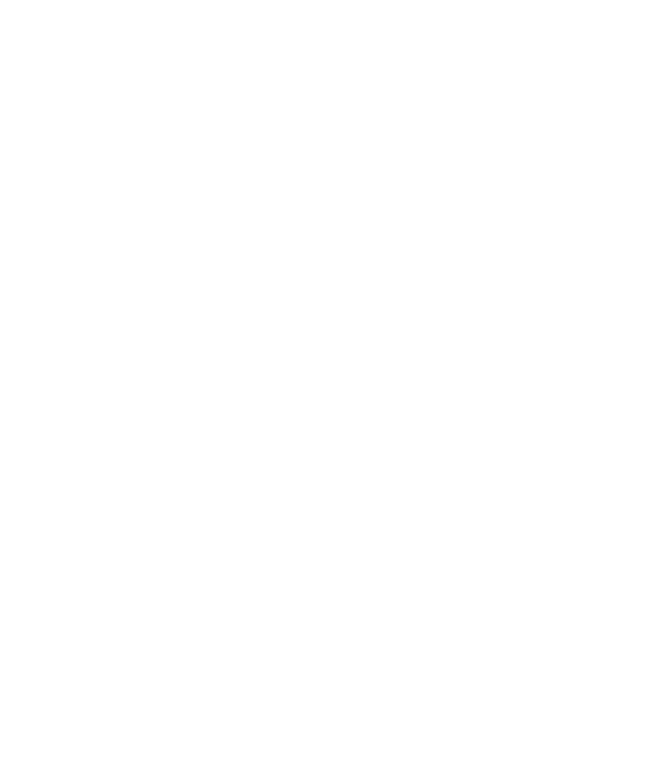
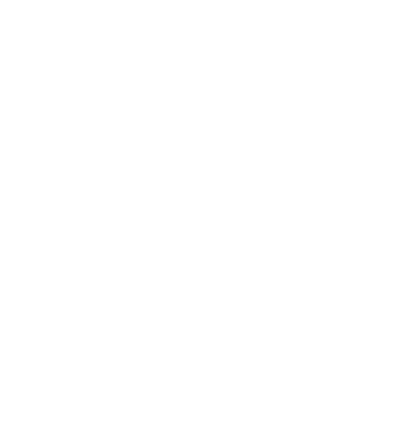
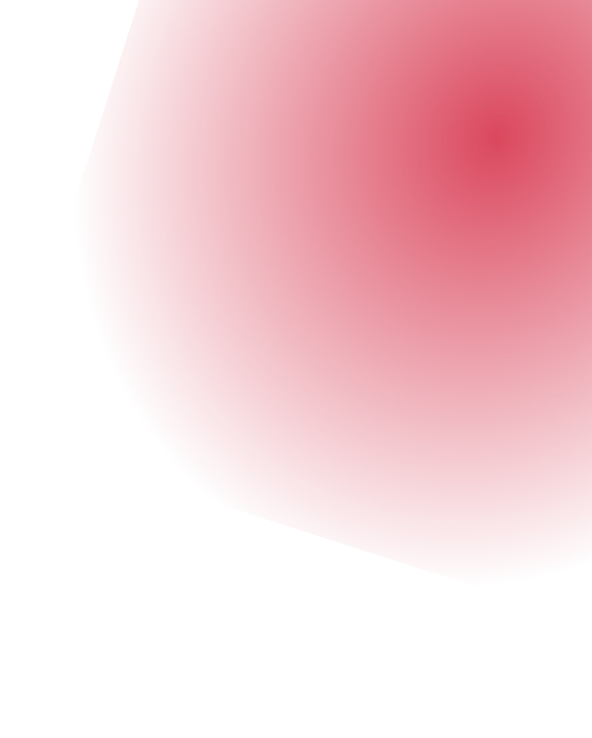
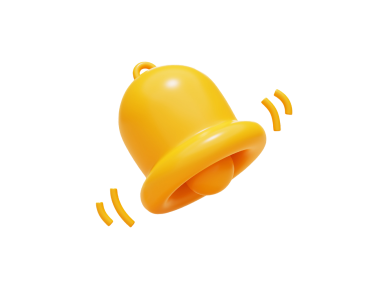
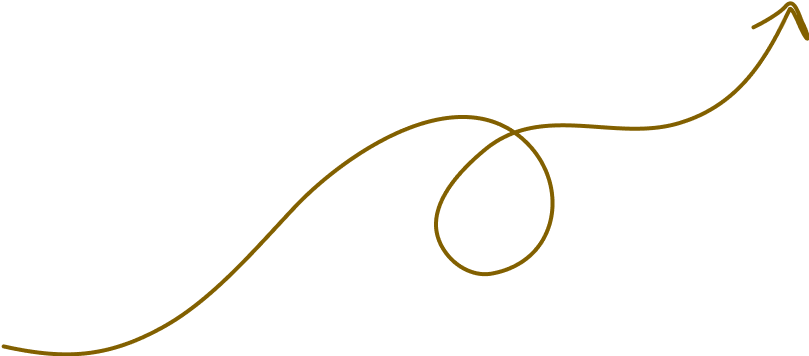