Data science has evolved from traditional analysis to cutting-edge machine learning and AI applications. Today, it serves an essential purpose in heterogeneous industries, from driving strategic decisions to personalising customer experiences and shaping public policies. As the field sees technological advancements, let’s explore future trends and innovations shaping data science.
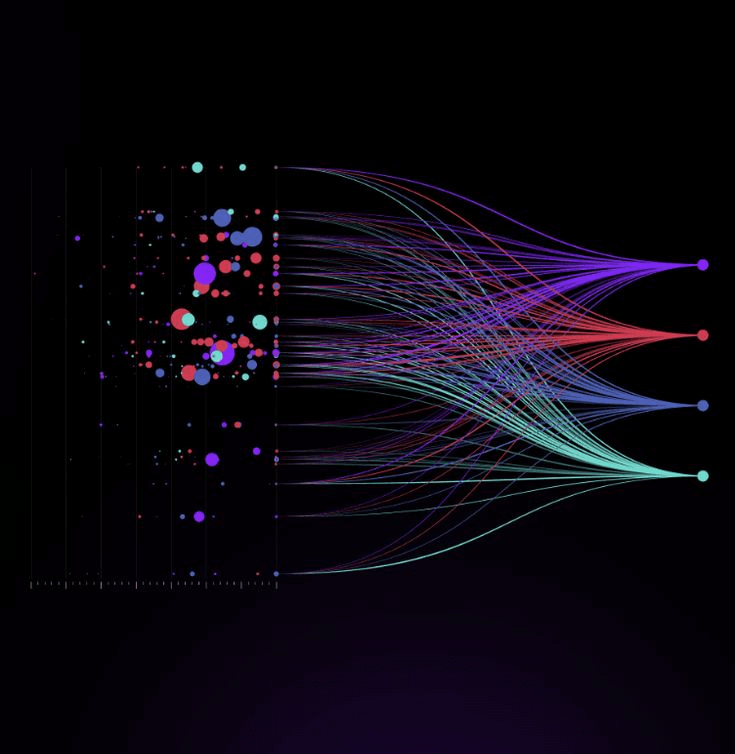
Heightened Emphasis on Ethical AI and Data Privacy
As data science evolves, so do ethical AI and data privacy concerns. Regulations like GDPR have set new principles for the usage of user data, making companies more responsible for the ethical usage of data. Other organisations, such as Data Science UA, dealing with computer vision software development, have transitioned to transparent AI models so that their algorithms act responsibly towards the users, eradicate biases, and stay in the periphery of the ethical dimensions. This trend represents a paradigm shift toward responsibility with AI and helps the users set up and maintain trust with the service providers.
AutoML: The Rise of Automatic Machine Learning
Automation in machine learning democratises the domain of data science by automating such mundane tasks as choosing data preprocessing and model selection for building a model. Thus, it changes the analytics landscape by bringing the power of machine learning to a wide range of users, business analysts, and domain specialists and making quick, efficient decision cycles across many industries a reality. Therefore, in the context of machine learning innovations, automation could assure innovation and improve competitive advantage in an increasingly data-driven world.
Real-Time Data Analytics and Edge Computing
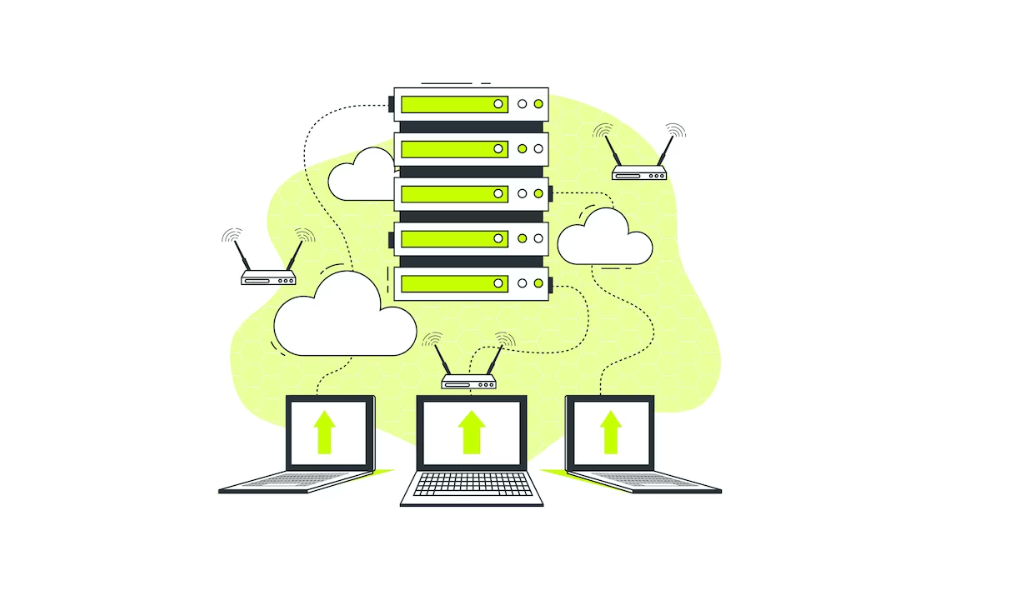
Real-time analytics processes the data in real-time; hence, it diverges from the traditional way of processing in batches. If integrated with edge computing that is processing data closer to where they are sourced, thus reducing latency to give faster insights is incredibly revolutionary to industries like IoT, finance, and health where real-time decision-making counts since it not only offers improved user experiences as well as improved operational efficiency but also provides companies with the vital competitive edge in this highly fast-paced market.
Enhanced Analytics for Data Democratization
Augmented analytics leverages AI to automate data preparation and generate insights and explanations. It enables users to interact with data in natural language to democratise information and make data analysis possible without requiring expert knowledge in non-technical employees. This will hugely upgrade decision-making processes across organisations by supplying intuitive tools like Tableau and IBM Watson Analytics, which empower all employees toward a very strong data-driven culture that permeates every department and drives innovation and efficiency throughout the enterprise.
Enhanced Natural Language Processing (NLP) and Language Models
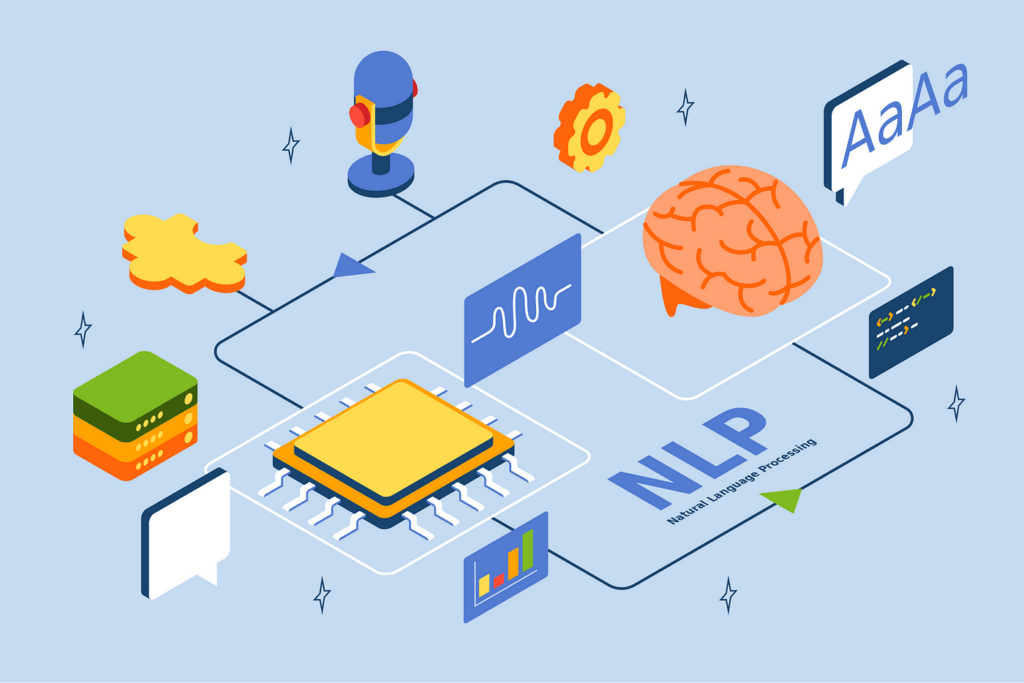
Breakthroughs in NLP by GPT and BERT have opened doors to language understanding and generation in ways nobody could have imagined. Models, ranging from chatbots and sentiment analysis to the automatic translation of languages, allow for unparalleled accuracy. NLP models can be complex, produce responses similar to humans, improve customer service, and open the gates to content creation. Applications are so versatile that NLP will soon be an even more integral part of any data-driven organisation.
Quantum Computing in Data Science
Quantum computing can revolutionise data science, unleashing the capability to solve complex data problems at extremely high speeds. It is still in its early stages, but it has the potential to significantly change cryptography, optimisation, and large-scale simulation in the coming years. Companies like Google are investing in quantum research, seeing it as crucial. This technology will eventually allow data scientists to process large datasets faster than ever, unlocking insights that were previously unreachable.
Data Science for Environmental and Social Good
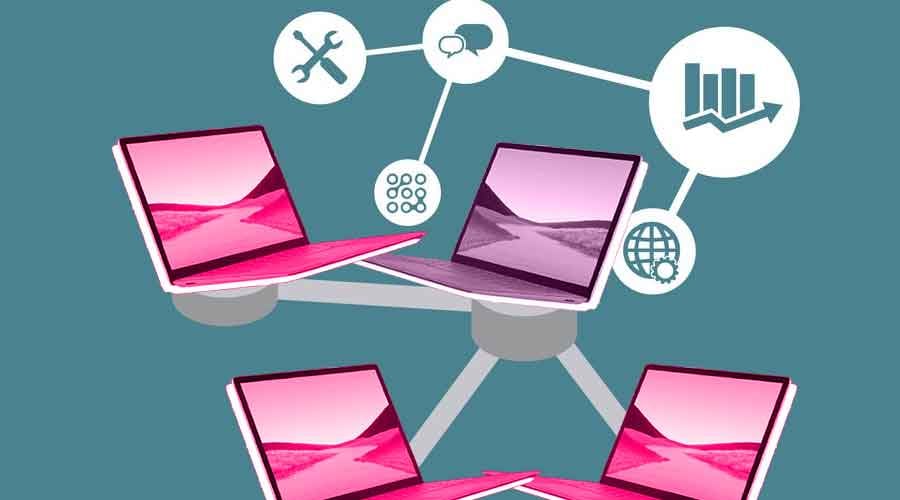
Data science primarily applies to business applications. However, it is also increasingly used in climate change research or developing predictive healthcare models for societal issues. Data-driven projects help organisations monitor environmental impact, improve resource usage, and shape policies that can lead to sustainable lifestyles. This trend highlights data science’s role in fostering socially responsible approaches to global challenges.
Innovations to Watch: AI-Driven Data Science Tools and Platforms
New tools powered by AI are starting to emerge that will support data scientists in data wrangling, visualisation, and predictive modelling. Richer functionality on platforms like DataRobot and RapidMiner simplifies sophisticated data tasks and unlocks faster and more accurate analyses. Equipping organisations with tools helps them stay competitive. These tools accelerate time-to-value and drive efficiency in data science processes. The future evolution of data science tools will facilitate easier workflows in data-driven areas. Additionally, these tools will empower businesses to better utilise insights. This will promote innovation and foster a culture of continuous improvement across all levels of the organisation.
Conclusion
Data science is an ever-evolving field that transforms industries and decision-making for businesses. More organisations are realising that data-driven insights can create operational efficiencies and encourage innovation. To compete in today’s data-driven world, businesses must exploit the latest trends in data science. This includes AutoML, ethical AI, and quantum computing. These advancements are necessary not only for automating workflows but also for resolving complex challenges with unprecedented speed and accuracy.
Firms should follow these developments in data science to use them strategically for long-term value creation. In a data-driven world, these advancements enable organisations to adapt to changing market dynamics. They also help create better experiences for customers. Additionally, businesses can make informed decisions that align with their long-term objectives.
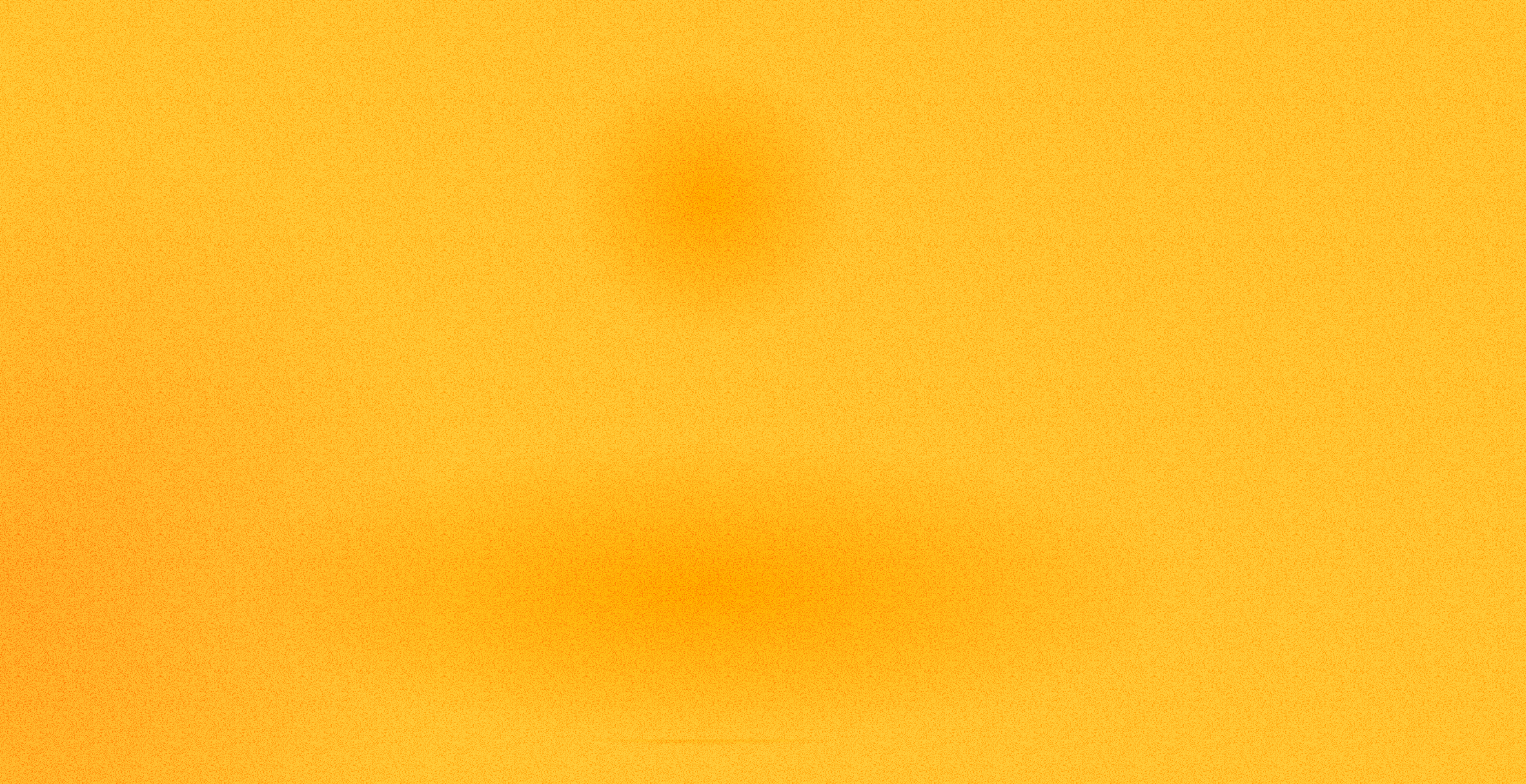
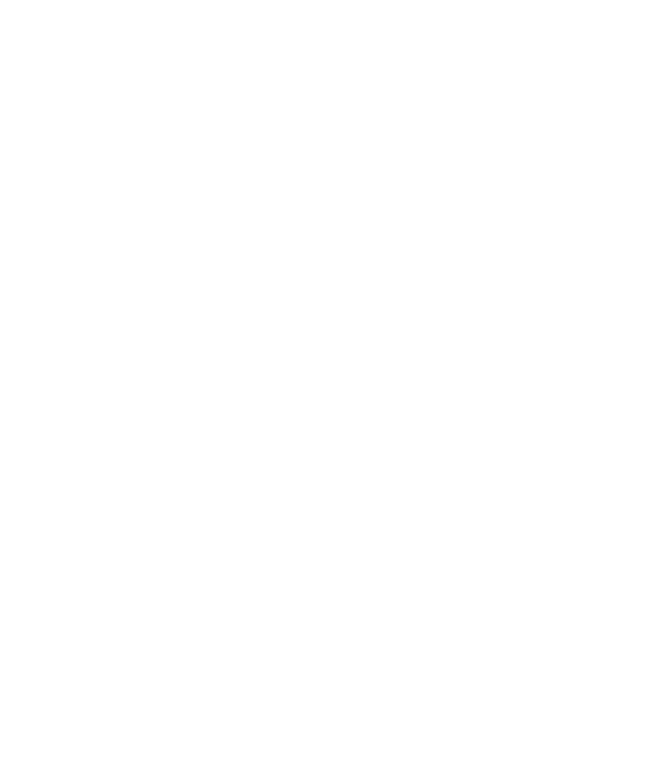
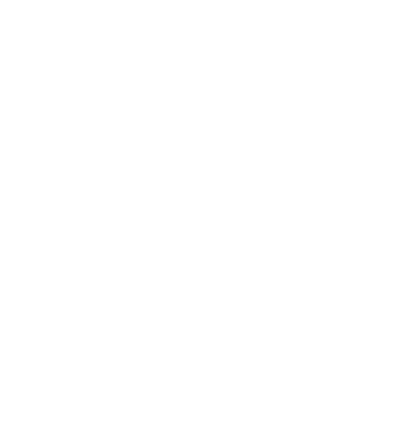
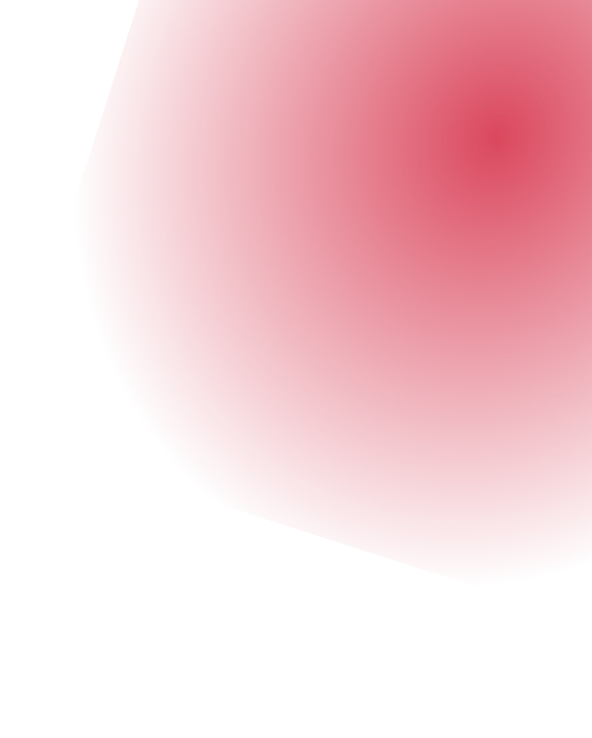
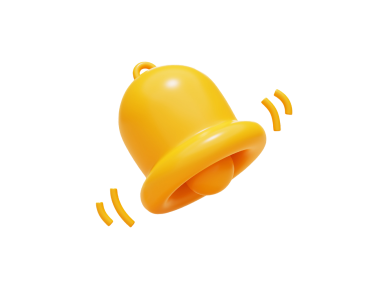
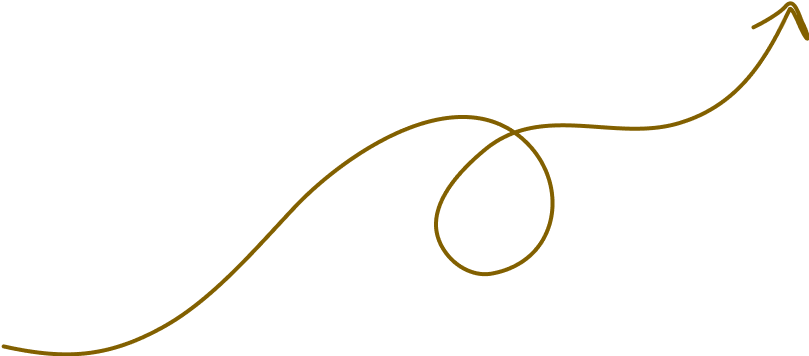
Read more topics
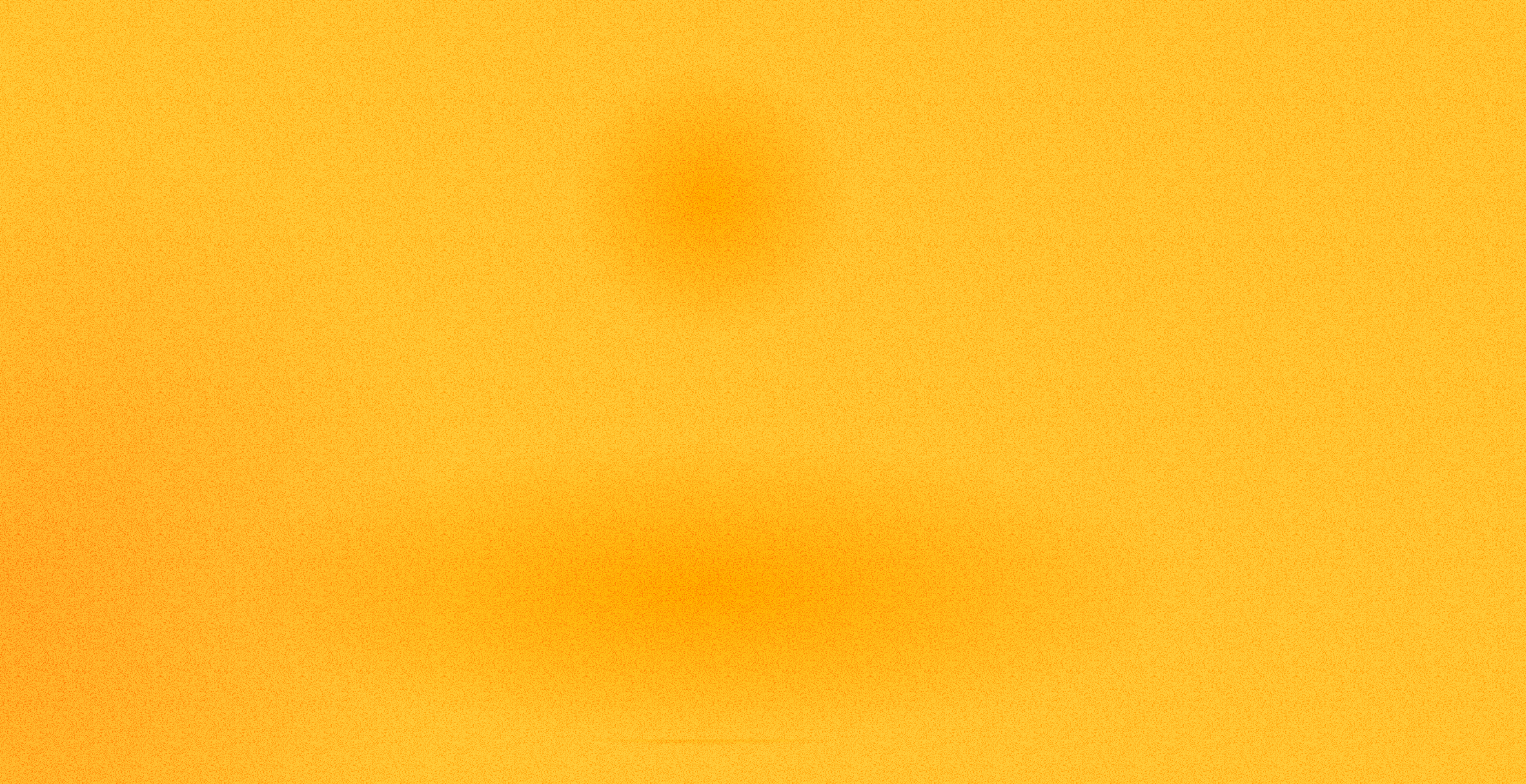
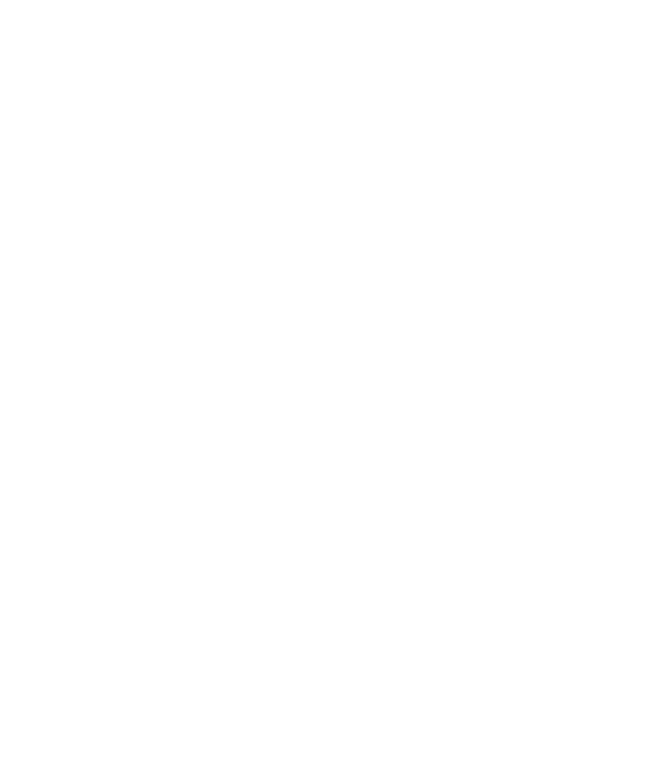
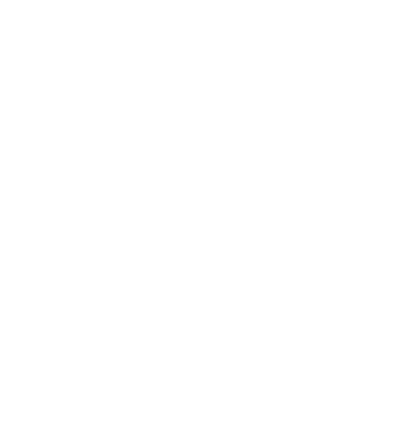
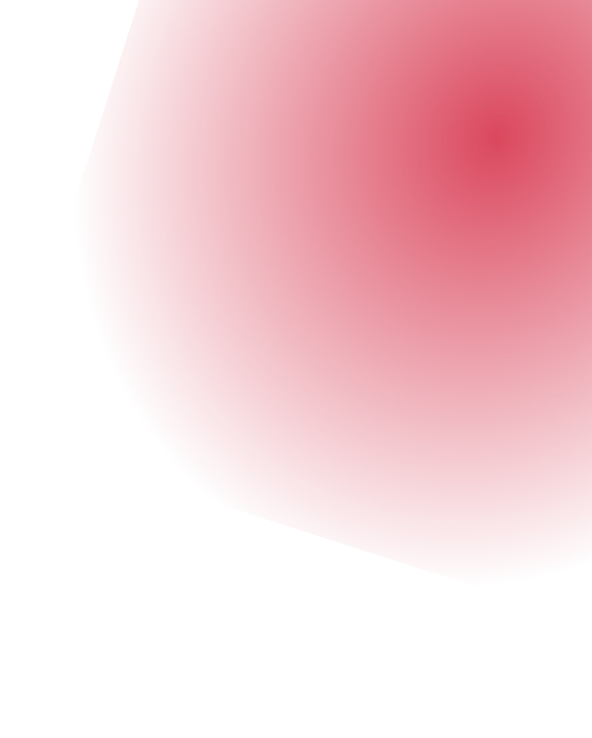
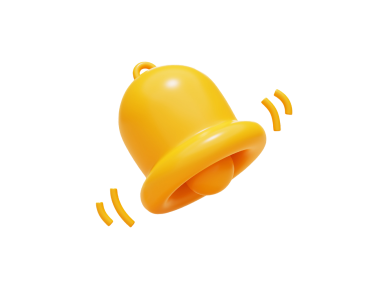
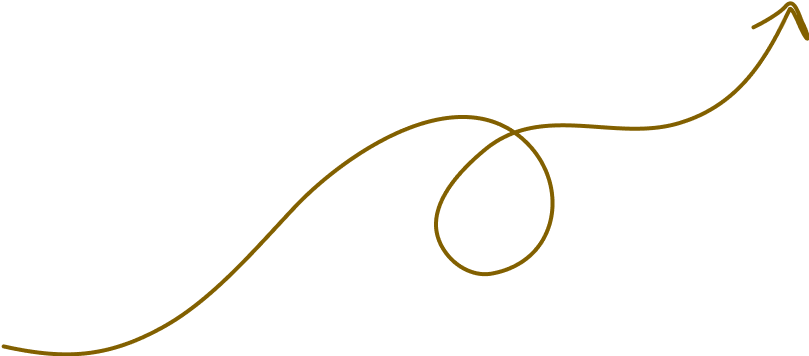