​The integration of machine learning in IoT is revolutionizing industries by enabling devices to process data intelligently and autonomously. Experts refer to the combination of Artificial Intelligence and IoT as the Artificial Intelligence of Things (AIoT), which enhances operational efficiency and decision-making across various sectors.
Analysts project that the AI in IoT market will grow globally at a compound annual growth rate (CAGR) of 19.8%, reaching a value of USD 9.25 billion in 2024 and climbing to USD 47.78 billion by 2033. The rapid growth is a proof to the global trend in the adoption of AIoT solutions.​
According to the AI in manufacturing, companies, which deploy AI in industrial training plants, have been able to increase its production by 10 to 15 percent and lessen EBITA by 4 to 5 percent. These improvements highlight the tangible benefits of integrating machine learning in IoT within industrial settings.
By the end of 2024, experts project the number of connected IoT devices to grow by 13%, reaching 18.8 billion. This expansion reflects the rising demand for remotely connected devices with intelligent data processing capabilities.
With more advanced machine learning algorithms, IoT devices offer the opportunity to automate, gain efficiency, and innovate in ways we’ve never been able to before. Machine learning and IoT, in this regard, is not just a trend but a transforming power that will decide the future of technology.
Real-World Applications Driving Adoption
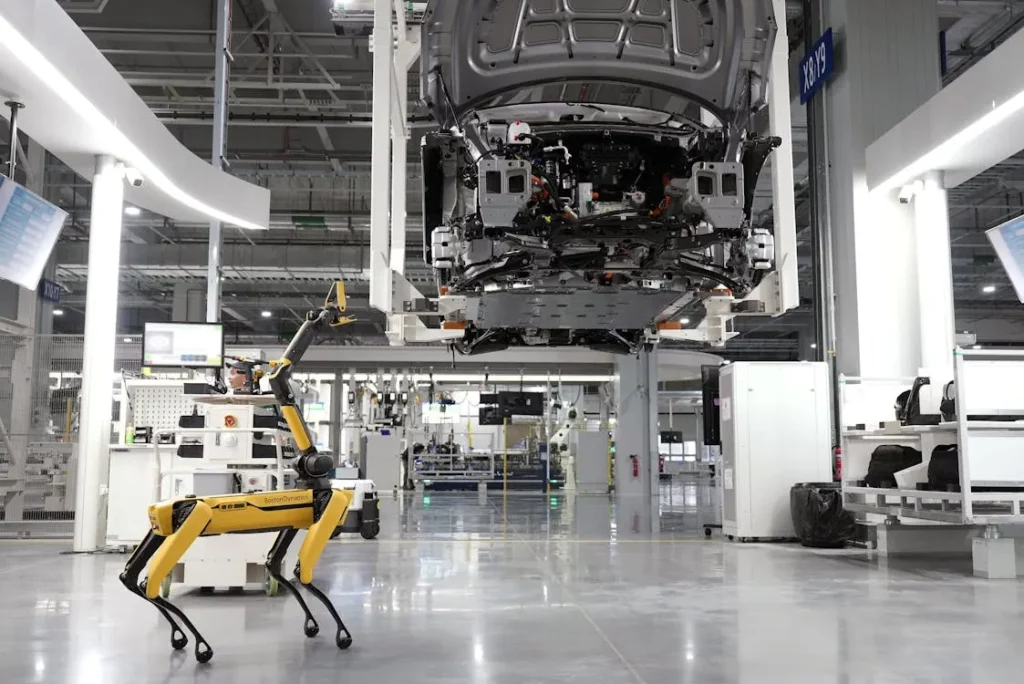
​Machine learning in IoT is transforming industries by enabling devices to process data intelligently and autonomously. This is an integration that increases operational efficiency and increases the quality of decision making across many sectors. The AIoT market, currently at USD 10.8 billion in 2023, is to grow from USD 26.9 billion in 2024 to USD 183.6 billion by 2032 at a 24.6% CAGR owing to the rising government initiatives in digital transformation. The rapid growth also reflects a growing trend of AIoT solutions adoption in the world.​
Smart Manufacturing: Predictive Maintenance and Quality Control
Machine learning in IoT is revolutionizing smart manufacturing by enhancing predictive maintenance and quality control processes. IoT sensors integrated with machine learning algorithms enable manufacturers to forecast when their equipment will fail, before it rears its ugly head. Such an approach ensures substantial downsizing of unplanned downtime and maintenance costs. For example, predictive maintenance can achieve 30% to 50% decrease in equipment stoppages as well as 20% to 40% increase in equipment life. ​
The global predictive maintenance market accounted for another USD 10.93 billion in 2024 and is to reach USD 70.73 billion by 2032 pushing a CAGR of 26.5%. This growth underscores the increasing reliance on machine learning in IoT for maintenance strategies.​
In quality control, machine learning algorithms monitor data in real-time via IoT sensors collection, in order to detect defects or deviations from the production standards. It allows for the immediate corrective actions in real time, preventing wasting and achieving increased product quality. For instance, Siemens made IoT possible and in place in quality control processes that decreased defect rates by 18% and customer satisfaction improved. ​
Concurrently, the AI in predictive maintenance market is to achieve USD 2,306.2 million by the end of the year 2033 and is to grow at a CAGR of 12.3% over the period of 2024 to 2033. This trend highlights the expanding role of machine learning in IoT within the manufacturing sector.​
Smart Cities: Traffic, Energy, and Waste Management
​Machine learning in IoT is transforming smart cities by enhancing traffic, energy, and waste management systems.​
Traffic Management
AI driven traffic systems are being implemented in cities to relieve congestion. A $350,000 trial has also seen South Australia put AI powered road cameras onto the roads, which use traffic flow information to change signal timings in an attempt to reduce delay and economic losses.
At a CAGR of 32.2%, the global AI for Smart City Traffic Optimization market, worth USD 7.5 billion in 2024, is to reach a market value of USD 122.3 billion during 2034. ​
Energy Management
The integration of IoT and the machine learning technique allows for smart energy usage in the smart city. Machine learning algorithms analyze data from smart meters and sensors to predict energy demand and optimize distribution, costing less to produce and also reducing the negative impact on the environment. Such systems can have a great benefit on improving the energy efficiency in urban areas. ​
The IoT Energy Management is to expand from USD 71.23 billion in 2023 to USD 311.86 billion by 2032, with a CAGR of 17.89% during 2023 – 2032. ​
Waste Management
Machine learning in IoT is also improving urban waste management. With sensors, smart bins can help monitor the number of waste and optimize the route, thus saving operational costs and helping reduce the environmental impact. For instance, in Wyndham City, Australia, smart bins have reduced garbage truck trips by 80% within six months.
Healthcare: Patient Monitoring and Anomaly Detection
​Machine learning in IoT is revolutionizing healthcare by enabling continuous patient monitoring and early anomaly detection. Smartwatches and biosensors, bearing wearable IoT devices, record real time data of all of their vital signs including the heart rate, blood pressure and oxygen saturation. This data is then analyzed by machine learning algorithms to detect deviations from the normal patterns for the physicians to take timely medical interventions.​
Remote Patient Monitoring
According to a new report, the global remote patient monitoring devices market is to register 19.1 percent compound annual growth rate (CAGR) from 2024 to 2032, reaching USD 203.68 billion by 2032, from the revenue level of USD 50.39 billion in 2024. The growth signals the steadily rising demand for IoT devices for continuous health tracking. For example, wearable sensors can automatically take health measurements from patients in places other than patient –visited clinics and save the need for frequent in –person visits .​
Anomaly Detection
Anomaly detection is possible with the help of the machine learning algorithms that diagnose unusual patterns in the data collection from the IoT devices that indicate that there could be some health issues. According to a study, via AI, the anomaly detection in smart healthcare can alert providers about possible health issues in real time to provide better patient care and lower costs .​
Case Study: Wearable Health Monitors
Devices like the Embrace2 smartwatch exemplify the integration of machine learning in IoT for healthcare. By monitoring patients’ physiological signals — including heart rate variability and skin temperature — and employing machine learning algorithms, Embrace2 can detect anomalies in epilepsy patients such as seizures. This innovation provides real-time monitoring and alert system for patient safety and ensures quality of life.​
Challenges and What’s Changing
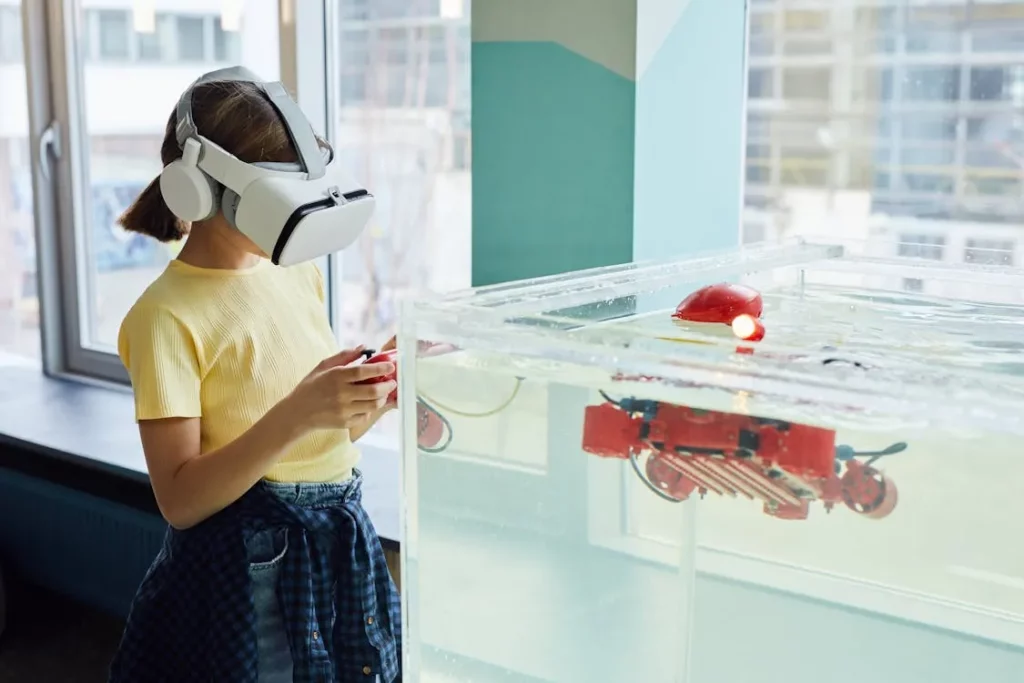
​Machine learning in IoT is transforming industries by enabling intelligent data analysis and automation. However, there are several challenges that organizations have to deal with.​
Data Privacy and Security Concerns
​Integrating machine learning in IoT raises significant data privacy and security concerns. The phenomenal growth of IoT devices has increased the attack surface for cyber vulnerabilities. The number of IoT devices is expected to increase 13% from 2023 to the end of 2024, up to 18.8 billion. ​
It has caused the surge in these attacks targeting these devices. Specifically, one in three data breaches now involves an IoT device — and the fact remains that they’re prone to be vulnerable. ​In home networks, the average volume of attacks per device, per day, is ten. Security solutions like Bitdefender block approximately 2.5 million threats daily, underscoring the persistent risks. ​
One of the major reasons for such vulnerabilities is the poor security implementations of many IoT devices. A survey found that 44.5% of consumers do not even have any mobile security solution in use on their devices which makes them prone to malware, phishing, and data breaches. ​
This is also financially expensive for businesses. The average costs of data breaches involving the Internet of Things devices have proven to be higher and 34 percent of the affected enterprises have $5 million to $10 million of the losses. ​
Organizations are thus investing in advanced security solutions to address the challenges. According to Global Market Insights, the global IoT security market was worth $34.2 billion in 2023 and is to grow at a CAGR of 18.5%, reaching $112.4 billion by 2030. ​
With machine learning algorithms, we can implement IoT security to detect such threats and subsequently mitigate them in real–time. With these, such algorithms are able to use patterns and anomalies to provide proactive defense mechanisms to prevent cyberattacks.
Hardware Limitations and Data Volume
Integrating machine learning in IoT presents significant challenges due to hardware limitations and the vast volume of data generated by connected devices.​
Hardware Limitations
IoT devices can be limited in both computational resources, processing power, memory, and energy capacity. These limitations affect the deployment of such complex machine learning models directly on the devices. This is because typical microcontrollers in IoT devices may not have enough capabilities to run complex algorithms efficiently. In order to tackle this, researchers are designing models suitable for resource constrained hardware. There are various techniques for delivering the models on microcontrollers, such as model quantization and pruning. These models can achieve meaningful compression without sacrificing much accuracy, enabling more efficient on device processing. ​
Data Volume
There has been an exponential increase in data generation from IoT proliferation. By 2024, the world will generate a data base on the digitization of activities around the globe and the data volume is about 149 zettabytes. Additionally, processing and analysis of this immense data flow and storage challenges are tremendous ones. Latency and bandwidth constraints present such large scale data to traditional centralized cloud infrastructures in handling such data in an efficient way. However, to ease these problems, something called edge computing is taking up the process of processing of data closer to its source. This approach decreases latency of, and bandwidth usage for, machine learning applications in such IoT environments. ​
Emerging Solutions
Efficient machine learning models are not deployed enough, which incurs runtime latency, and current methods to quantify their cost do not work on very small devices. This development and reducing the need of constant data transmission to central servers remove the bandwidth issues and improve the real time processing capabilities. For instance, tailored accelerator chipsets have been developed to support the efficient on-device processing of machine learning algorithms and to allow the use of device side AIu functions in constrained resources environments. ​
Why It’s the Future
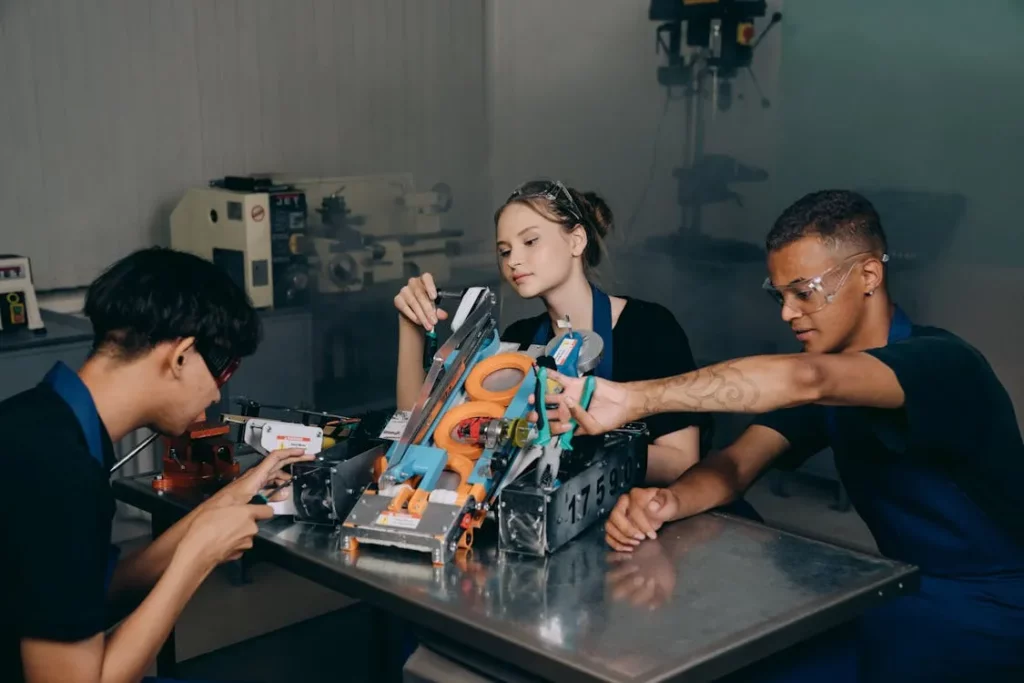
​Machine learning in IoT is rapidly becoming a cornerstone of technological advancement across various industries. Despite all the challenges, the increasing reliance on intelligent data processing to enhance operational efficiency and decision-making is apparent.
Scalability of Machine Learning in Iot Ecosystems
​Due to the expansion of the Internet of Things (IoT) at a rapid pace, scalable machine learning solutions are necessary to manage and analyze the large amount of data. According to the forecasts, at the end of 2024 there will be 18.8 billion connected IoT devices, with a 13 percent increase compared to previous year. ​
Challenges in Scalability
The amount of data coming from billions of IoT devices is too immense to manage and process. Systems that utilize traditional centralized computing find it difficult to cope with these types of large-scale data due to the latency and bandwidth constraints. To get around these problems, machine learning models are being built on a large scale that can quickly mine valuable intelligence from the data generated by IoT. ​
Edge Computing Solutions
Processing data closer to its source has emerged as a critical solution and has become a very effective solution to the problem of edge computing reducing latency and bandwidth usage. This increased the efficiency of machine learning applications in the IoT ecosystem. ​
Cloud-Based Scalability
Cloud environments are to enable scalable machine learning models for the IoT data analytics. With cloud resources, large scale data processing can become easier and thereby one can take care of real time analysis and decision making. ​
Future Outlook
In current times, managing the influx of increasing amounts of data and improving the operational efficiency becomes essential for IoT ecosystems, where the scalable machine learning models can ensure a smooth flow. With the proliferation of IoT devices, the kanban approach will be critical for organizations that want to use IoT data.
Ongoing Investment and Innovation Trends
​The integration of machine learning in IoT is experiencing significant growth, driven by substantial investments and continuous technological advancements. According to TMR, the global artificial intelligence in IoT market stood at a value of USD 9.25 billion in 2024; it is to witness a CAGR of 19.8% from 2024 to 2033, reaching a value of USD 47.78 billion in 2033. ​
Investment Trends
More and more investors are realizing that there is something to combine AI and IoT technologies for the future. In fact, the amount of connected IoT devices is projected to increase by 13 percent by the end of 2024, hitting 18.8 million devices worldwide. Essentially, the surge speaks to the increasing ecosystem support for machine learning applications throughout the IoT world.​
Innovation Trends
Technological innovations are enhancing the capabilities of machine learning in IoT. The rollout of 5G networks is ongoing, and the viability of edge computing is possible due to that, whereby local devices can process data with less latency. Furthermore, there was development on specialized hardware including edge AI microcontrollers to process the feature better on the device. For example, STMicroelectronics announced the STM32N6 series microcontrollers specifically for use in edge AI applications and to achieve that, audio and image processing is done locally, without the need of bigger computers or data centers. ​
Market Predictions and Expert Outlook
​The integration of machine learning in IoT is heading for substantial growth. The global artificial intelligence (AI) market was at approximately USD 638.23 billion in 2024 which is to reach USD 757.58 billion by the end of 2025, growing at a compound annual growth rate (CAGR) of over 19.20% from 2025 to 2034. ​
In particular, AI in the IoT segment is growing in good measure. The market size was USD 5.8 Billion in 2023 and is expected to grow at a CAGR of 10.8% from 2024 to 2033, to reach USD 16.2 Billion by 2033. ​
Experts attribute this growth to the rapid increase in connected devices and advancements in various AI technologies. They expect the global IoT devices market to grow by 13% by the end of 2024, reaching 18.8 billion devices. It is due to this surge that development of machine learning applications in these IoT cubes is becoming common.​
Support for the use of AI together with IoT is universal amongst industry leaders. The combined effect of these technologies is expected to spur innovation in different sectors, improving operational efficiency and creating new capabilities.
Conclusion
The convergence of AI and IoT, often referred to as AIoT, is a significant trend shaping the future. Because of this integration , systems become smarter and hence more capable of complex decisions , which actually leads to higher operational efficiency and creates more opportunities for innovation. With the number of connected IoT devices expected to reach almost 30 billion by 2030 , machine learning will play a major role in controlling and oversimplicity data produced by IoT devices.​
At Designveloper, we’ve seen this transformation firsthand. As a leading software development company in Vietnam, we’ve built intelligent, scalable systems that combine real-time IoT data with powerful machine learning algorithms. Furthermore, our experience includes over 100 successful projects across sectors—from smart logistics to AI-driven healthcare apps. If you’re looking to build the future today, we’re ready to help. Let’s transform your ideas into intelligent, data-driven platforms—secure, scalable, and ready for what’s next.
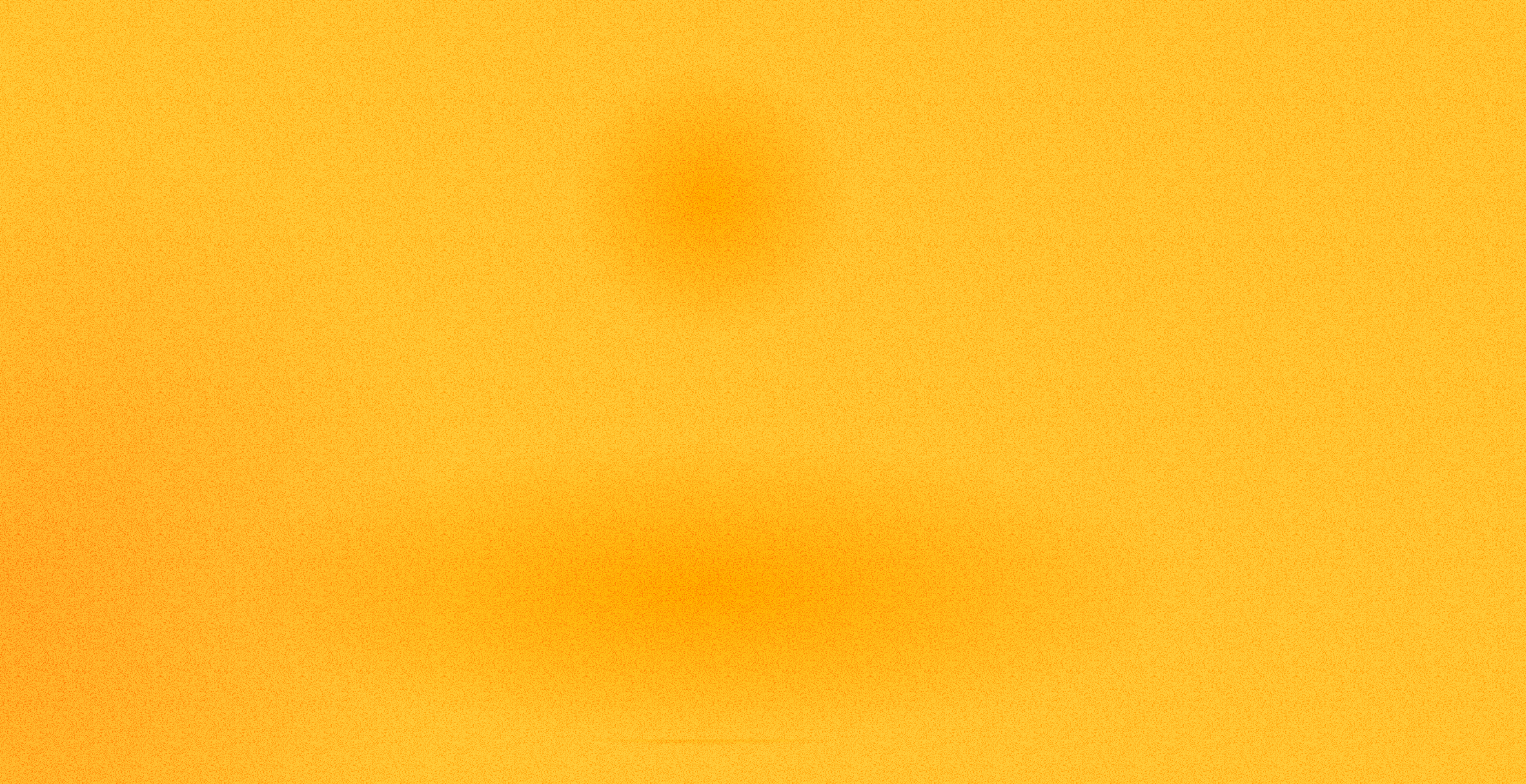
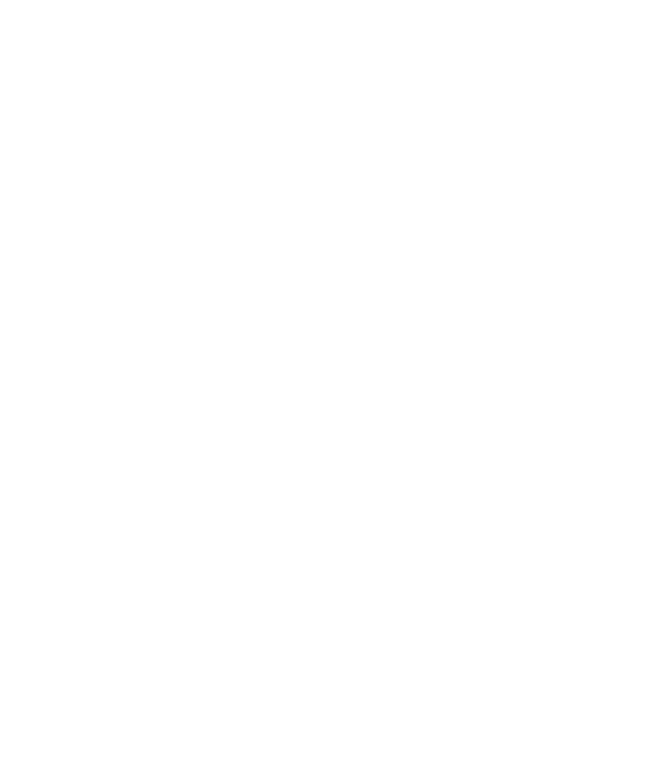
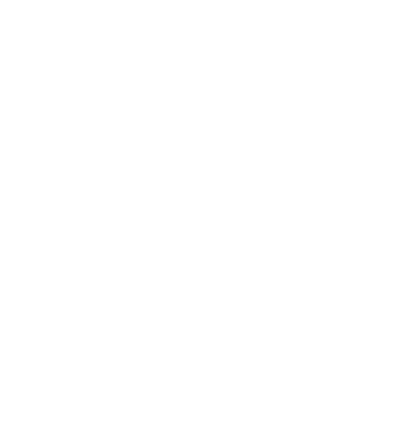
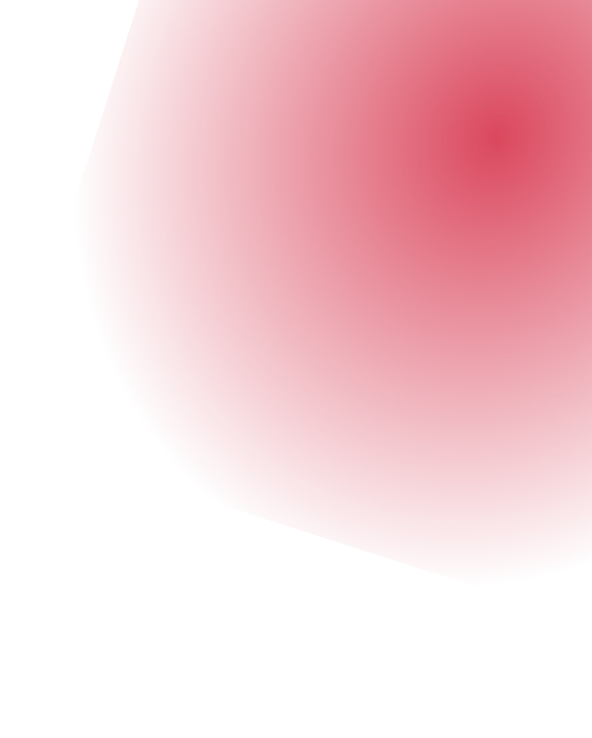
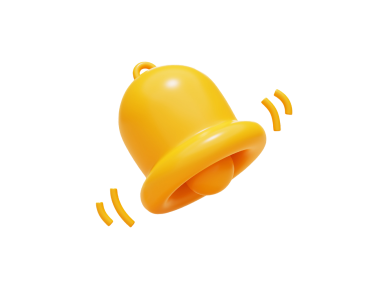
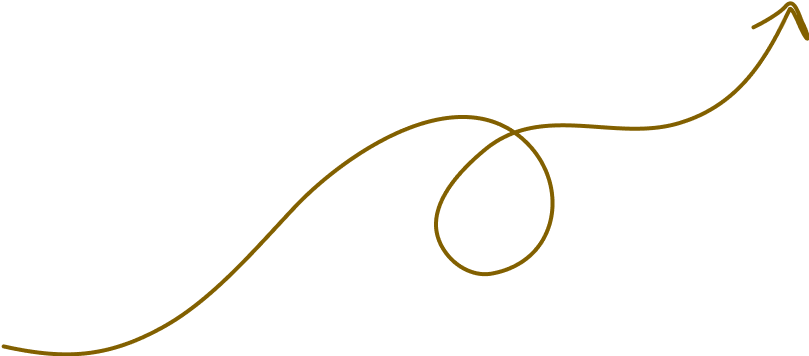
Read more topics
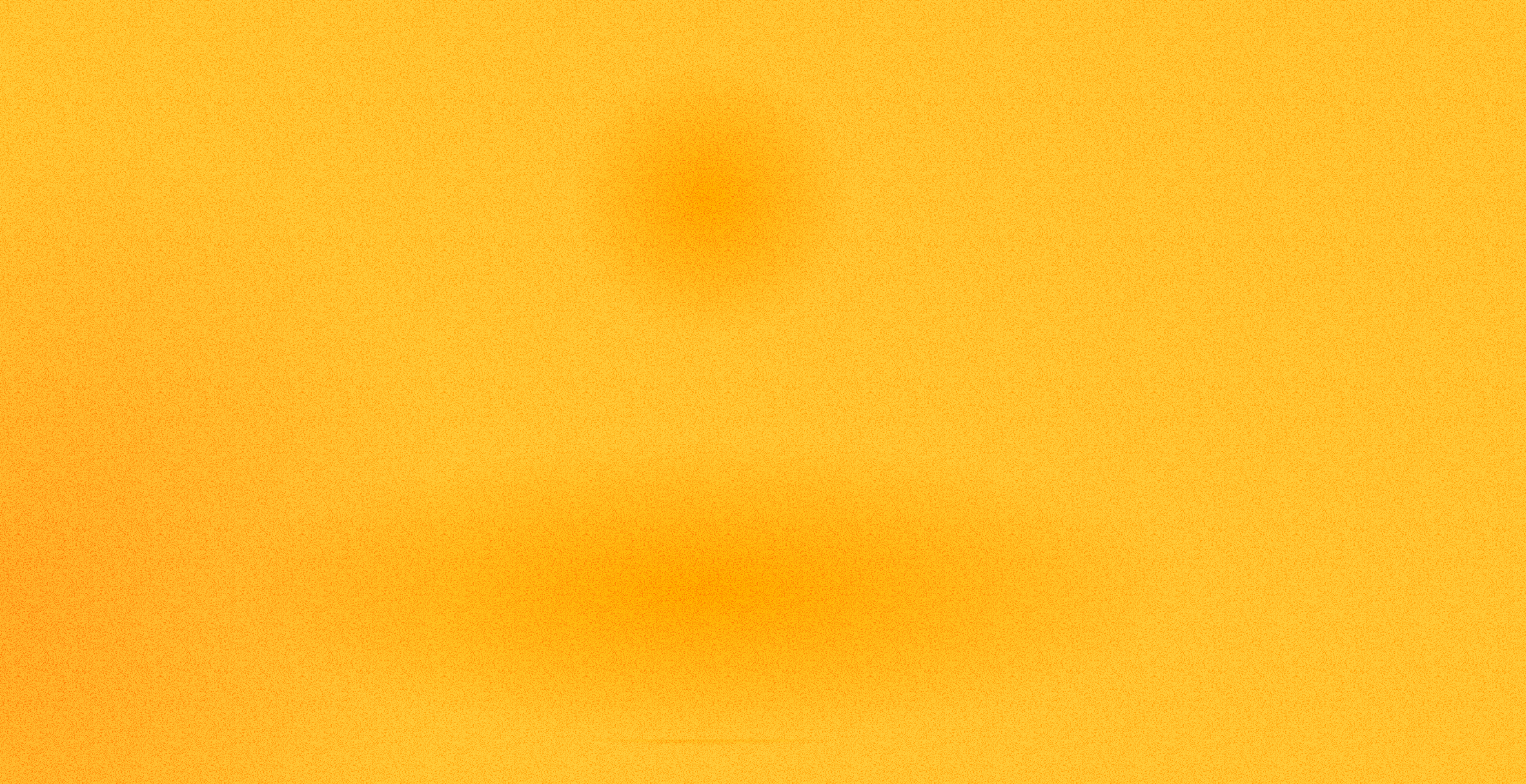
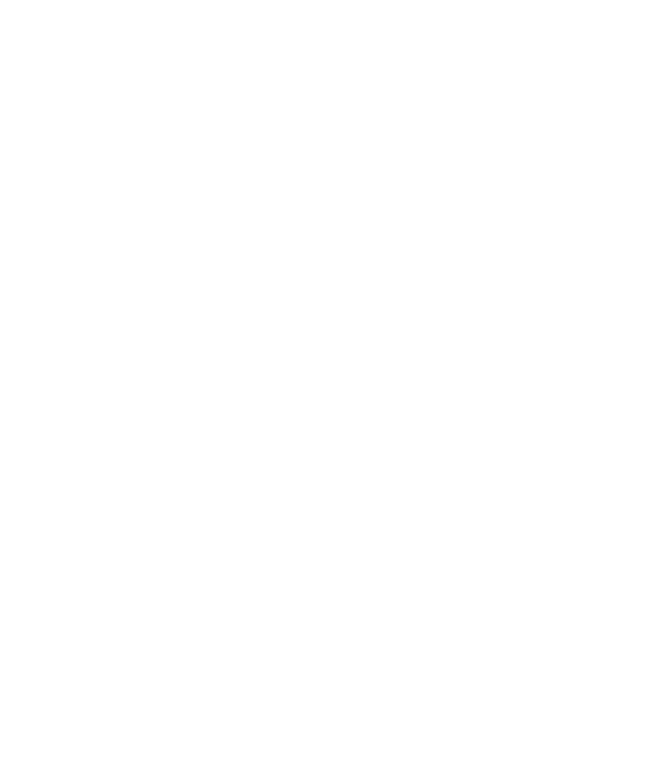
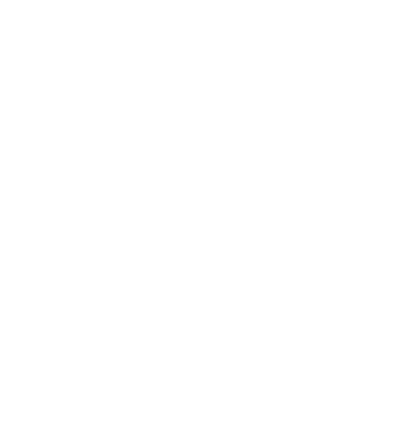
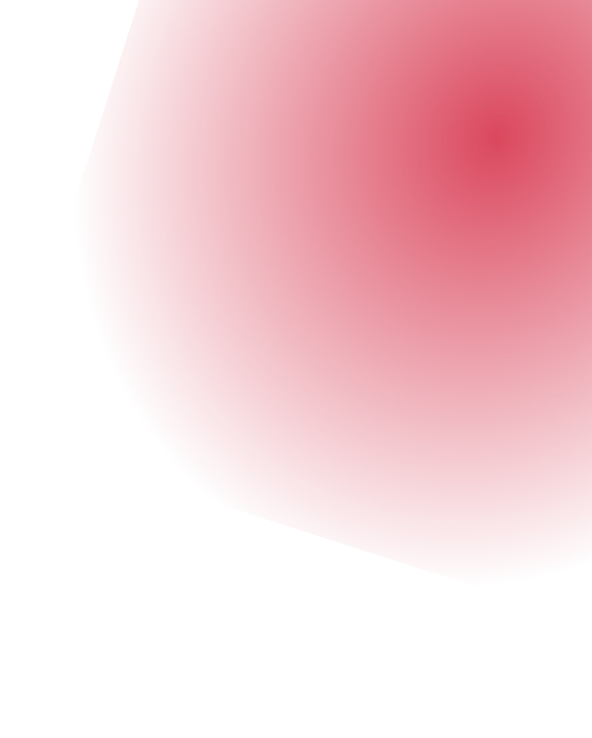
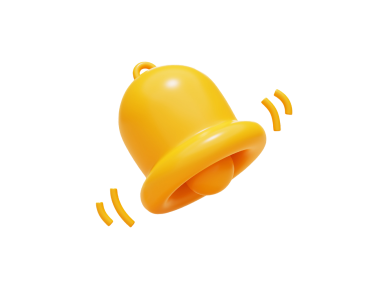
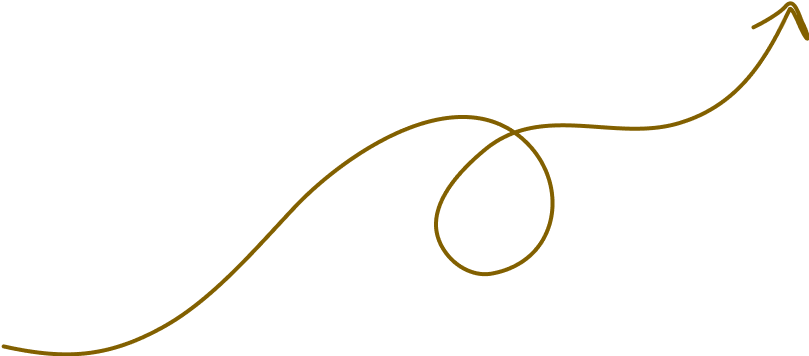