Today, there is progressively confusing usage of the terms machine learning vs AI as if they meant the same thing, though they are actually different fields. Understanding what exactly machine learning is and how it differs from AI is quite necessary if you want a claw in the ever evolving world of tech. As per the latest AI Index report by Stanford University, AI has already surpassed human performance in image classification, visual reasoning and beyond. However, machine learning is just a subset of AI and it is about the algorithms that enable a computer to learn based on data.
One application people are most familiar with is recommendation engines on streaming platforms, but some other examples include automated stock trading. On the contrary, AI encompasses many other technologies such as robotics, natural language processing, and so on. For instance, OpenAI’s GPT-4 is sophisticated enough to practically understand and produce text that appears to come from human writers. Machine leaning models are also progressing, but the industry is driving the change.
For the latest trends and statistics, read this detailed article from Designveloper.
Definition of AI vs Machine Learning
AI and Machine Learning has been rapidly changing the industries all around the world. A report stated that there would be an increase of 40 percent in AI and ML jobs till 2025. Artificial intelligence refers to the ability of the machine to mimic human intelligent behaviour, to learn from given data and to respond to stimulus in the manner we humans do. AI consists of Machine Learning, which is the technique to make computers learn from data and can predict data out of it.
Definition of Artificial Intelligence
By definition artificial intelligence (AI) is the capability of a computer system to perform tasks that are normally done by humans. These tasks include natural language understanding, pattern recognition, making decisions, solving problems, etc. By being able to learn from the data, it is able to adapt to new input and run human-like tasks.
The latest statistics indicate that the global market of AI is anticipated to contribute at least $15.7 trillion by 2030. The rapid rise in AI industry growth can be seen that it is playing a larger role in different fields.
Under the umbrella of AI, there are many technologies that can be used such as machine learning, deep learning, and natural language processing. For example, machine learning algorithms can analyse large datasets and calculate trends or predict trends using their knowledge. An example of significant application is in healthcare where the AI systems can assist in identifying diseases and suggesting treatments on the basis of data of the patient.
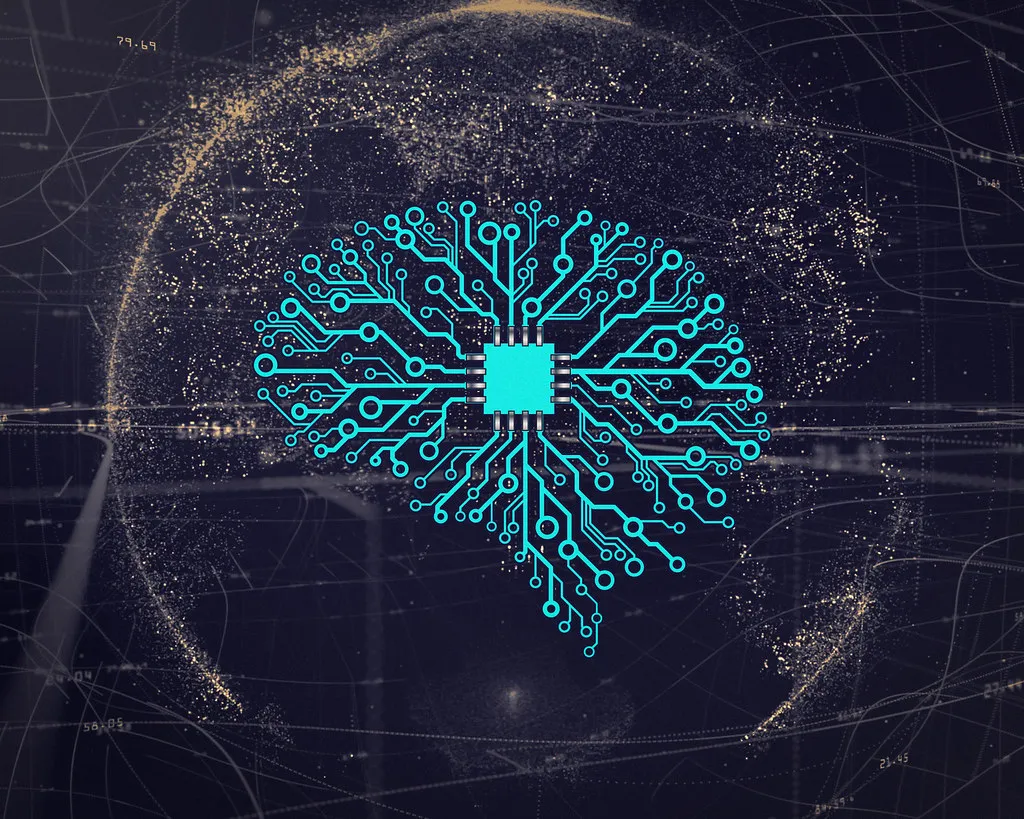
In addition, AI has seen major advances in the way it is incorporated into everyday life. Over 50% of U.S. mobile users use voice assistants such as Siri and Alexa every day and that’s where AI comes into play, in order to understand a user query and to respond to that query. Also, the AI driven Chatbots are an excellent tool in providing customer support in realâ time, improving customer experience and response time.
Although AI offers many advantages, there are also challenges. Some recent news was found when the BBC reported that AI chatbots are highly inaccurate when presenting summaries of news stories. This argues a necessity for continuous improvement and regulation of the AI world.
Definition of Machine Learning
Artificial intelligence or AI is the broader concept that everything from smartphones to machines can be made to perform tasks typically requiring human being involvement while machine learning is a narrow subset of AI that specifically deals with building systems that can learn from data (or can learn without being explicitly programmed) and make predictions in the form of decision making. Unlike how traditional programming works, in traditional programming rules are explicitly coded while in the case of machine learning algorithms, they use statistical techniques to help computers learn from and make decisions based on data.
According to recent statistics, the market of machine learning is going to grow significantly. GlobeNewswire reports that approximately $8 billion worth of the machine learning market was recorded in 2019 with an expected $117 billion value by 2027 at a CAGR of 39%.
There are various applications that use machine learning such as recommendation systems, speech recognition and computer vision. For instance, Netflix uses machine learning algorithms to make suggestions to its users in regard to movies and shows that they will most likely enjoy based on what they have watched in the past. And similarly, virtual assistants, like Siri and Alexa, employ machine learning to listen to and correspond to a phone call.
Core Differences Between Machine Learning vs AI
Artificial intelligence (AI) and machine learning (ML) are used as synonyms but they are not the same. For anyone getting into the realm of AI and ML, it is crucial to understand the major differences between machine learning vs AI. Statista reports that those AI technologies will rise from $200 billion in this year to $1.8 trillion in 2030. This distinction is important for one fact, that BI is growing at such a rate.
Scope and Goals: AI as the Broader Concept vs ML as a Subset
Knowing the difference in scope and goals of machine learning vs AI will be helpful in the long run, since you can better plan and understand your projects depending on the tools being used.
Scope of AI
AI seeks to develop systems that can carry out activities that, on the whole, demand human intelligence. It takes the form of problem solving, decision making, and learning. There are a lot of AI applications, which include: self-driving cars, virtual assistants like Siri and Alexa, AI powered chatbots, etc.
Scope of ML
Specifically, ML is a subset of AI that specializes in algorithms able to teach computers how to learn from data while improving over time without any specific guidance. It consists of training models on data used to make predictions or decisions without being programmed for each task. Some of the examples of ML applications are: recommendation systems on sites like Amazon and Netflix and predictive analysis in healthcare for early disease detection.
Goals of AI
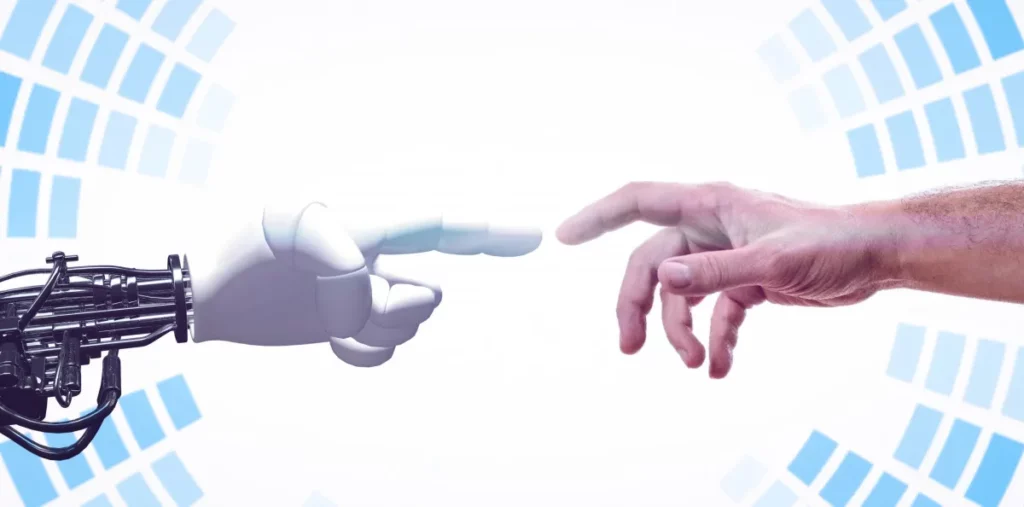
The main purpose of Artificial Intelligence is to build systems that perform tasks that previously needed human thought and reasoning. These include improving productivity, automating repetitive tasks and improving the decision making processes.
Goals of ML
The most important goal of ML is to design algorithms that can learn from the given data and make accurate predictions or decisions. One is by improving the performance of systems over time as more data is exposed to the system.
Functionality: AI’s Ability to Perform Tasks vs ML’s Learning from Data
In a recent report, it is expected that AI will provide a 21% net increase to the Gross Domestic Product (GDP) of the United States, by 2030. This demonstrates the leading role of AI in economic growth. On the other side, ML is making their industries better by automating repetitive tasks and improving efficiency. For example, ML algorithms help detect early sign of diseases through medical imaging in healthcare.
An example is obvious, such as self-driving cars or virtual assistants. In conversation we can mention the use of Tesla self-driving cars or ChatGPT, which are the ideas of AI which already exist and are commercially available. On the other hand, ML learns from data in predictive analytics and in e-commerce recommendation.
In short, AI is full of different functionalities, but when concentrating on learning by data, ML comes into play. In this sense, both are key technologies for the future of innovation and digital transformation.
Examples of Use Cases: AI vs ML
Artificial Intelligence (AI) and Machine Learning (ML) have their exclusive capabilities in transforming the business of several industries. Some specific examples of the use cases are as follows:
AI Use Cases
- Chatbots and Virtual Assistants: AI powers chatbots and virtual assistants like Siri, Alexa, and Google Assistant. These tools assist customers with their inquiries, give recommendations, set reminders and manage schedules and other related tasks.
- Customer Service: AI chatbots can assist routine customer service tasks that drive the need for customer support, thus decreasing customer wait time and satisfaction. According to the report, an example of use cases of AI chatbots is a company like Bank of America.
- Healthcare: AI powers diagnostic tools, personalized treatment plans and virtual health assistants. IBM’s Watson Health is an AI healthcare example that helps diagnose and treat patients with the aid of doctors.
ML Use Cases
- Image Recognition: The use of ML algorithms in image recognition is included in those such as facial recognition, medical imaging and autonomous vehicles. ML is used by Google Photos to identify and categorize images, for instance.
- Predictive Analytics: Predictive Analytics model that uses historical data to predict future trends of data. Forecasting demand and inventory management is done by retailers like Amazon through the use of predictive analytics.
- Fraud Detection: ML is used by Financial institutions to detect fraudulent transactions by applying patterns and anomalies in the transaction data. PayPal and other payment platforms leverage ML for this purpose.
For more detailed insights, you can refer to reports like the Stanford AI Index Report and the IBM Global AI Adoption Index.
How Designveloper Can Help Leverage the Power of Machine Learning and AI
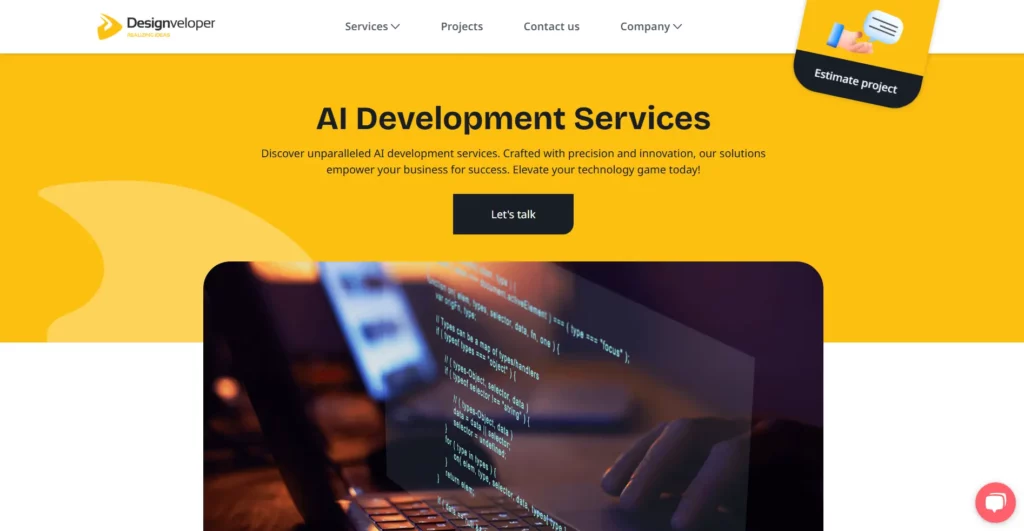
Designveloper is on the driver’s seat of the revolution induced by Machine Learning and AI, changing industries in great measure. Using our knowledge in AI development, we enable our clients to exploit these technologies as the tools for innovation and efficiency. Here’s how we can assist you:
- Custom AI Solutions: Designveloper is capable of creating custom AI solutions that are suitable across your sector. Regardless of whether you are seeking to automate urgent processes, raise the engagement with your customers or gain deeper insights of your data, our team of professionals will help you.
- Generative AI Services: We apply advanced algorithms when using generative AI services, and let it do dynamic and innovative solutions. Our AI solutions can impress and engage through creation of content as well as design of products to drive your business forward.
- AI Chatbot Integration: Enhance your customer engagement with our top-notch AI chatbot integration services. Seamlessly integrate AI technologies into your existing platforms to streamline communication processes and improve user experience.
- Data-Driven Insights: Leverage the latest advancements in machine learning to gain valuable insights from your data. With our AI solutions, we guide you to make better data driven decisions, build organisations which are more efficient and most probably grow.
- Scalable AI Applications: Designveloper offers Scalable AI Applications to innovate and boost productivity through reliable and scalable AI applications. We have the expertise in AI development and will trust us to turn your vision till reality.
- Expertise in AI Development: When it comes to AI Development, we know what we are doing. At Designveloper, we have a team of experienced developers and have completed multiple projects regarding AI development for businesses. We offer you the complete set of AI software solutions that can improve efficiency, user experience and advantage.
Conclusion
Knowing the differences between machine learning vs AI is very important to be able to take advantage of each one at their full potential. Recent statistics have shown very good results for AI on image classification and visual reasoning tasks. However, machine learning remains an essential part to advances in automation and data analysis. You can refer the practical use case of machine learning such as, recommendation engines, automated stock trading, etc. This understanding of the differences will help business and individuals make better decisions on how companies can benefit from the use of these technologies in their future growth and innovation.
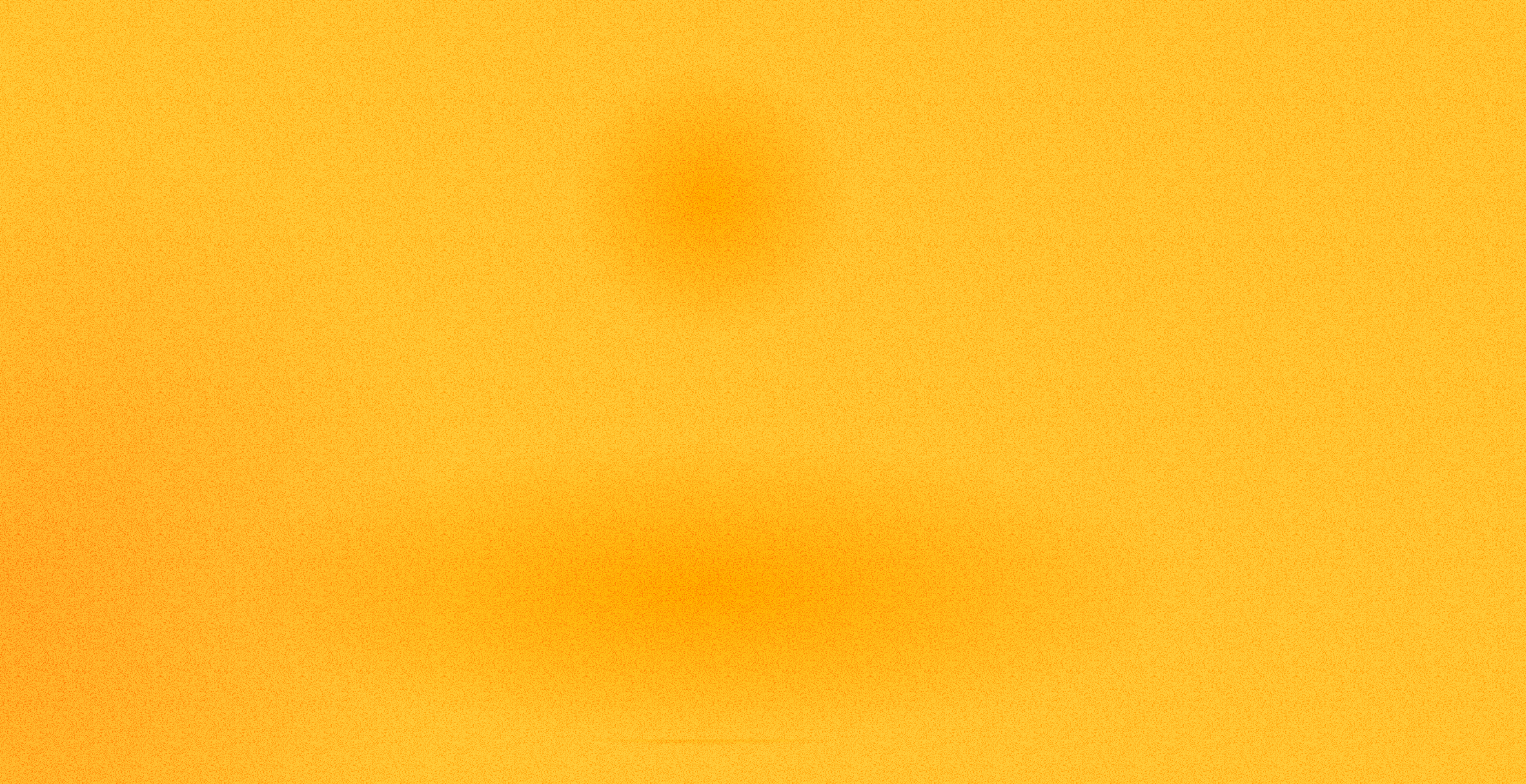
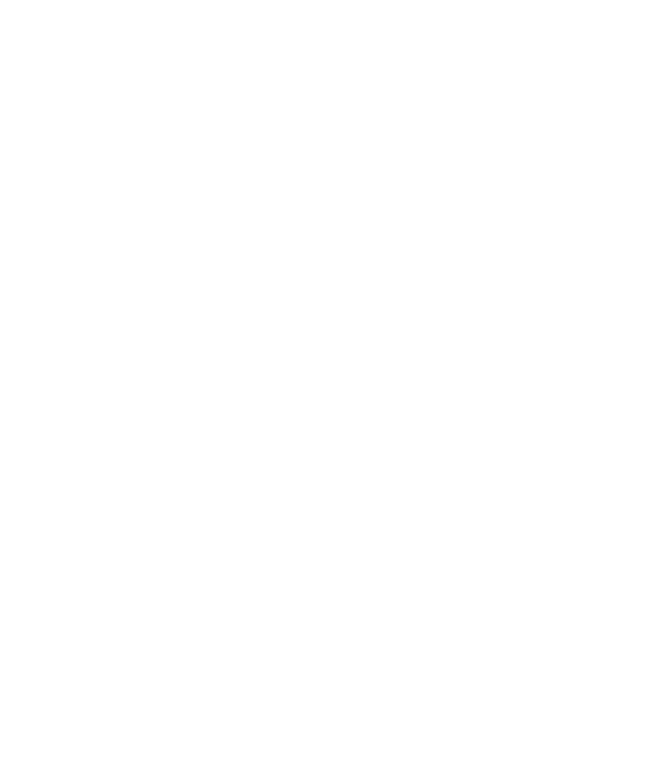
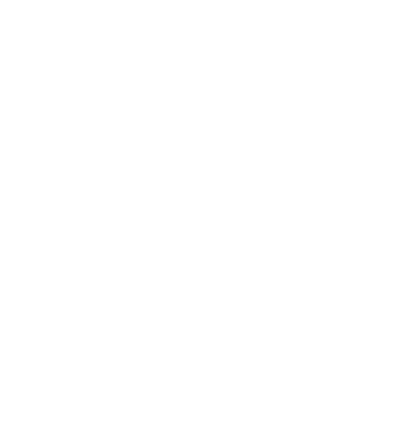
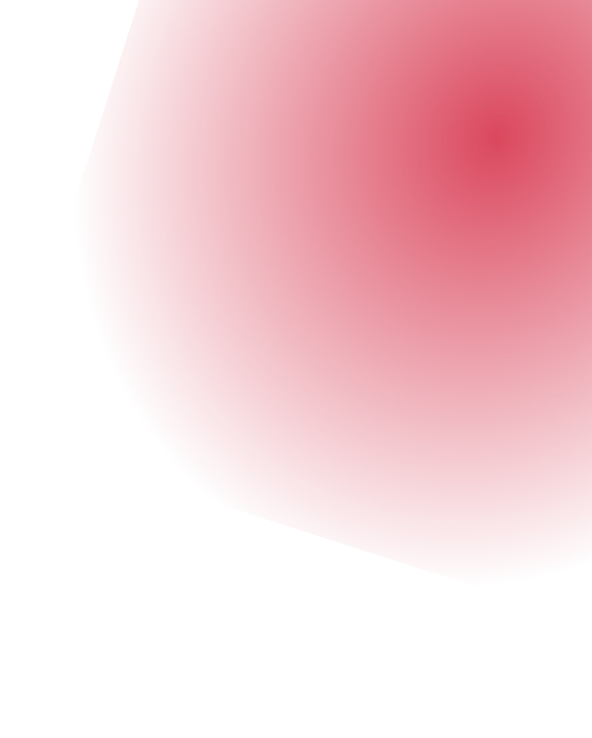
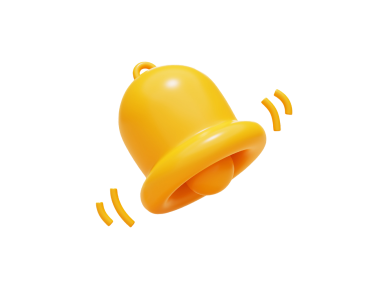
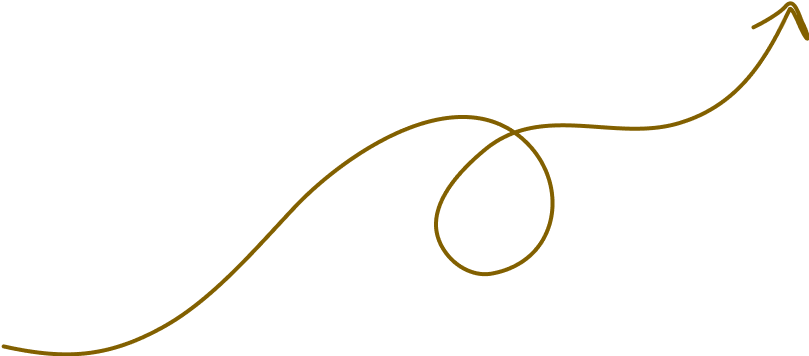
Read more topics
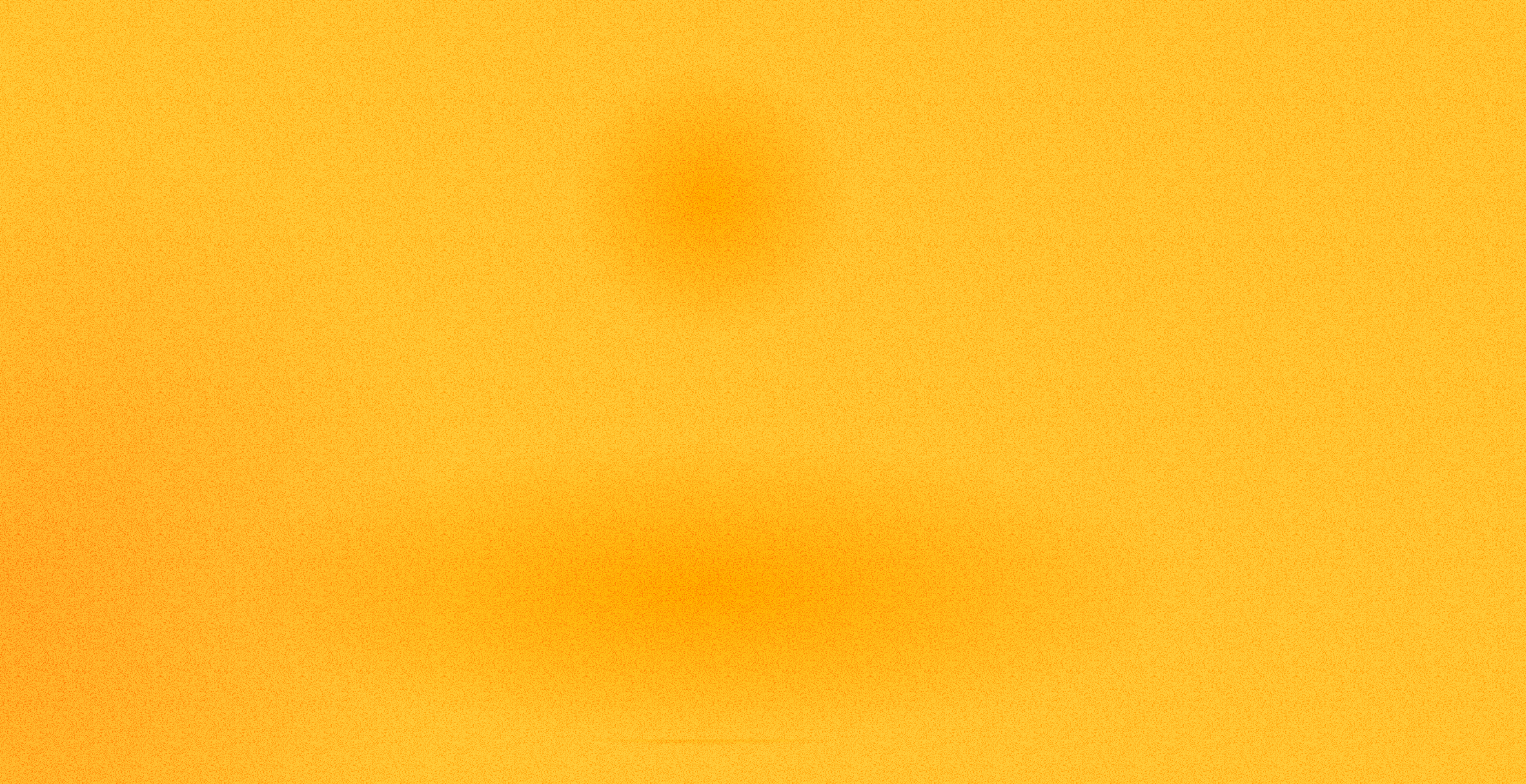
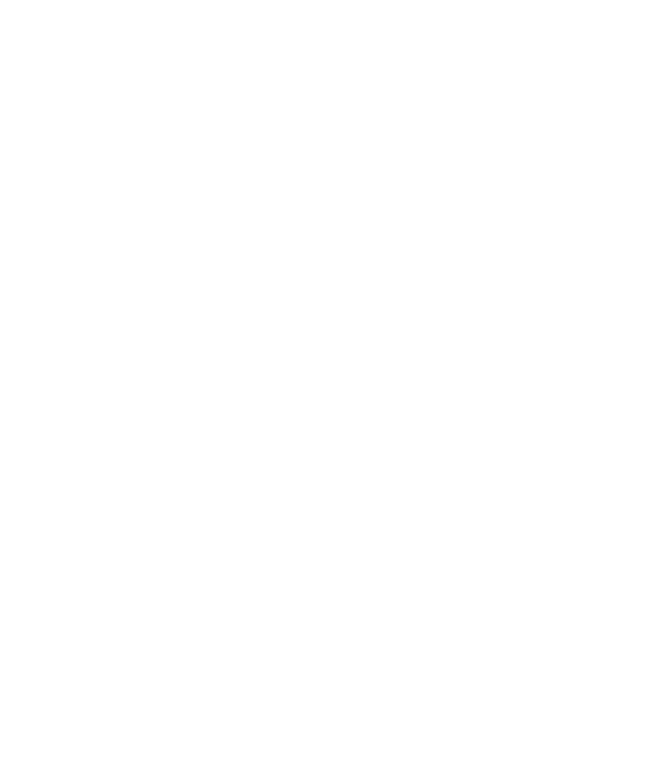
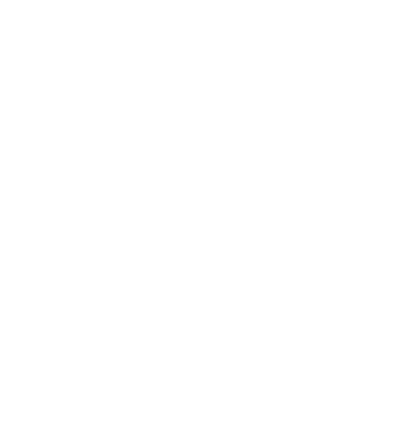
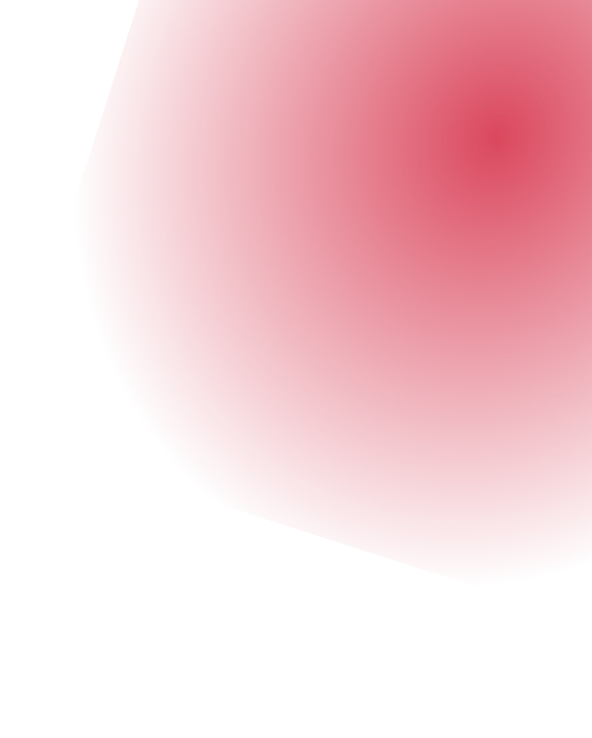
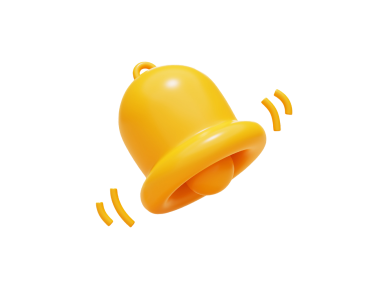
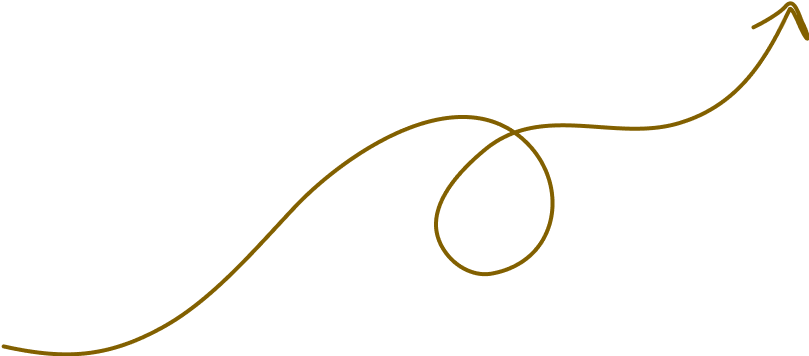