The Impacts of AI on Modern Data Integration
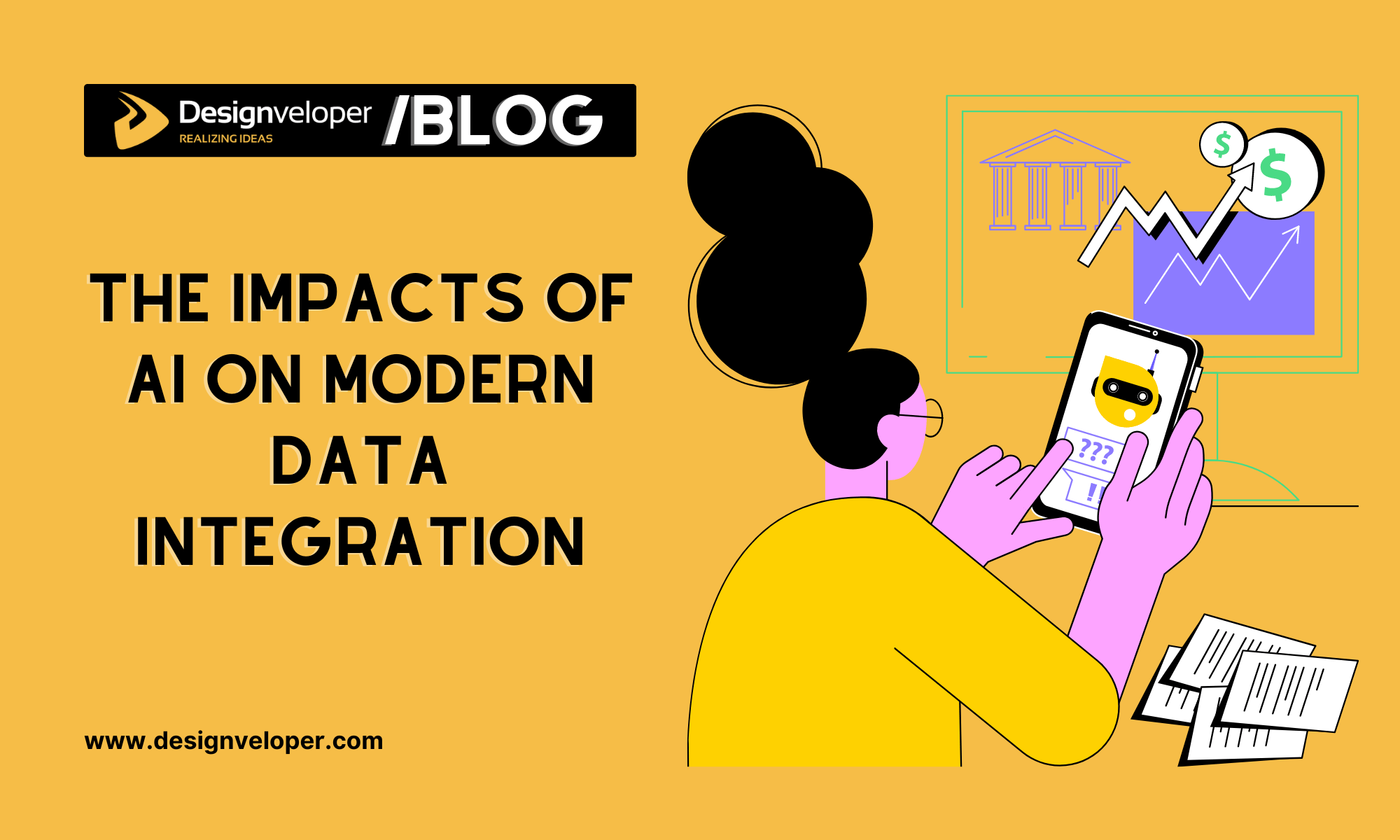
The use of AI in data integration is more than a trend; it is a strategic requirement. With over 57,933 AI businesses active globally, 25% of which are headquartered in the United States, the data integration and AI environment is fast expanding.
According to Statista, the market for artificial intelligence grew beyond 184 billion U.S. dollars in 2024, a considerable jump of nearly 50 billion compared to 2023. This staggering growth is expected to continue with the market racing past 826 billion U.S. dollars in 2030.
Data-driven decision-making is critical for every business looking to thrive in today’s competitive market. Data-driven decision-making with AI platforms can speed up company processes while saving time and money.
To remain competitive, firms must make timely choices based on data insights. Organizations are overwhelmed by the increasing accumulation of varied data sources, which range from established systems to emergent digital channels. Converting this data flood into useful insight has become a major issue.
Currently, firms devote more effort to data preparation than analysis. This inefficiency reduces their capacity to capitalize on key ideas. To solve this, firms are looking for novel ways to speed up data integration and preparation procedures.
Data Integration (DI) paired with Artificial Intelligence (AI) provides a strong strategy for automating data preparation chores and realizing the full potential of big data analytics. By reducing manual intervention, this technique allows firms to focus on gaining insights and making educated decisions.
In this article, we will look at some of the challenges associated with integrating AI into data, as well as the many opportunities that exist if you understand how to do it efficiently.
Types of Data Integration
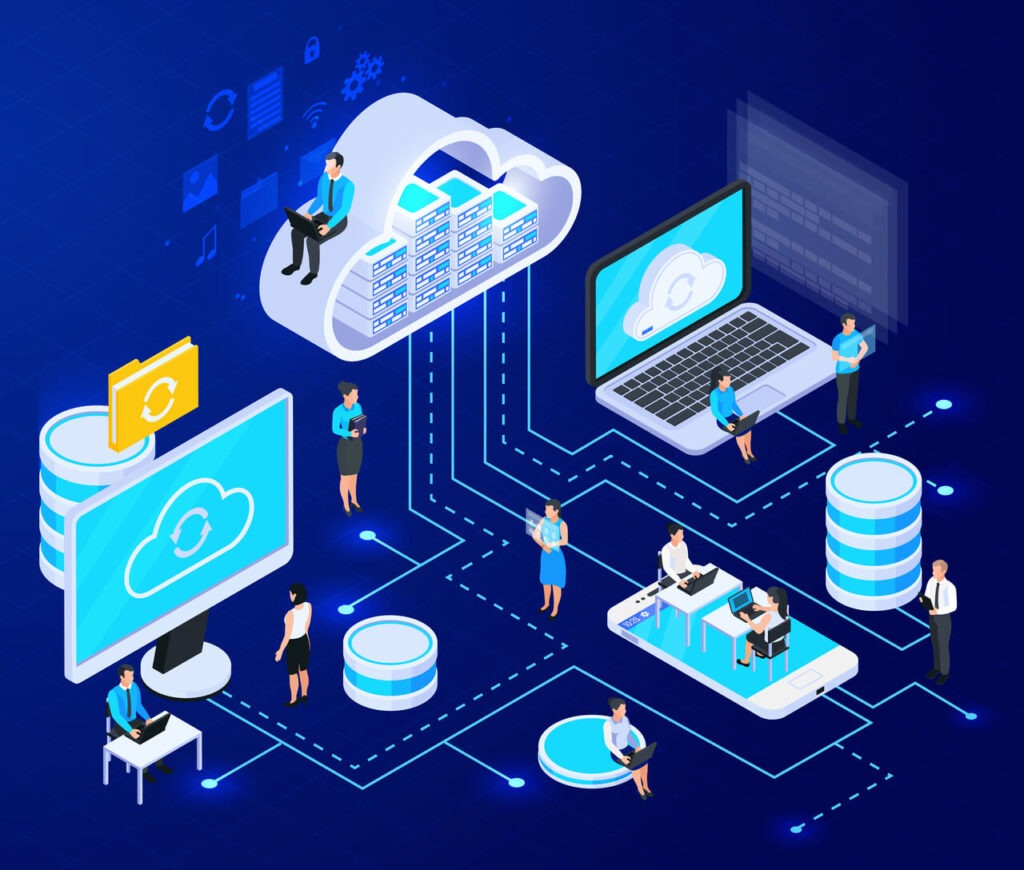
To grasp the advantages of incorporating AI into data gathering, it is essential to examine the various types of data integration. There are 5 typical types of data integration:
1. Manual Data Integration
Manual integration is the most basic — and, in some ways, the most heroic — data integration strategy. This type of data integration requires your company to hire a specialized data engineer who oversees and codes data connections in real-time. A data engineer must establish the proper link to each data source, clean and restructure the data as needed, and manually move it to the desired destination.
2. Data Integration with Middleware
Middleware, as the name implies, is software that stands between programs and transfers data. A middleware data integration solution converts data from a variety of applications into databases. Middleware, which is often automated, may frequently do data modifications to make it compatible with new systems. This is beneficial if your company is transitioning from older legacy systems to a variety of current apps since it generates a network of information available to everybody in your organization.
3. Uniform Data Access Integration (UDAI)
This kind of integration focuses on uniformly showing data for convenience of use while retaining the source data at its original location. Consider it a translation tool for hundreds of languages, each of which represents a unique source of business data. Data replication occurs when you access it, but the data is always stored at the source.
4. Common Storage Data Integration
As corporate data gets increasingly complicated and numerous, many organizations use an integration strategy as their primary storage method. Similar to uniform access, information is transformed before being transferred to a data warehouse, allowing your systems to access only one data source rather than hundreds. Because the data is in one location and available at all times, you may employ analytics and business intelligence (BI) tools as needed. Many data warehouses, as they are commonly referred as, are built around common storage integration.
5. Application-Based Data Integration Tools
Application-based integration software successfully locates, obtains, and transforms data before integrating it into the appropriate destination. This frequently includes automation, pre-built links to a wide range of data sources, and the capacity to connect to other data sources as needed.
The Roles of AI in Data Integration
Current Data Integration (DI) technologies are increasingly incorporating AI capabilities to meet enterprise demands. These AI enhancements in DI platforms are transforming how businesses make decisions:
- Prebuilt Mapping and Metadata Catalog: With prebuilt DI templates and system metadata catalogs, AI can automate the creation of data transformation mappings. This allows business users with limited technical expertise to utilize DI tools through simple drag-and-drop features, enabling them to spend more time on data analysis and trend identification using their domain knowledge.
- Fast Computational Speed: Effective use of machine learning (ML) with appropriate input parameters allows for faster and more efficient extraction of business insights from enterprise datasets compared to traditional business intelligence (BI) techniques. ML offers rapid computation power and requires less coding, helping achieve speed objectives.
- Big Data Processing: ML is highly effective for processing large volumes of data quickly and efficiently. Traditional DI tools often struggle with the speed required to handle vast amounts of data (in the zettabyte range or more) and to manage unstructured or semi-structured data formats to uncover hidden business insights. ML can navigate through big data structures of all formats to generate accurate data models and pipelines with minimal human coding intervention.
- Intelligence Through Autonomous Learning: As AI automates data transformation mapping in the ETL process, business users can focus more on identifying patterns and hidden trends from curated large datasets and applying statistical modeling to derive accurate business insights.
Use cases of AI in data integration
1. Mastering Address Parsing and Geocoding
Parsing and standardizing addresses is essential for logistics, marketing, and more. AI is making this process easier and more accurate.
- Address Standardization: AI models break down addresses into their components (house number, street, city, etc.), ensuring consistency across databases. This is invaluable for delivery services, helping them navigate complex addresses and optimize routes.
- Inference: AI can fill in missing address details by drawing inferences from context. For example, if an address lacks a zip code, AI can deduce it from the city and state information.
2. Helper Bots and Knowledge Empowerment
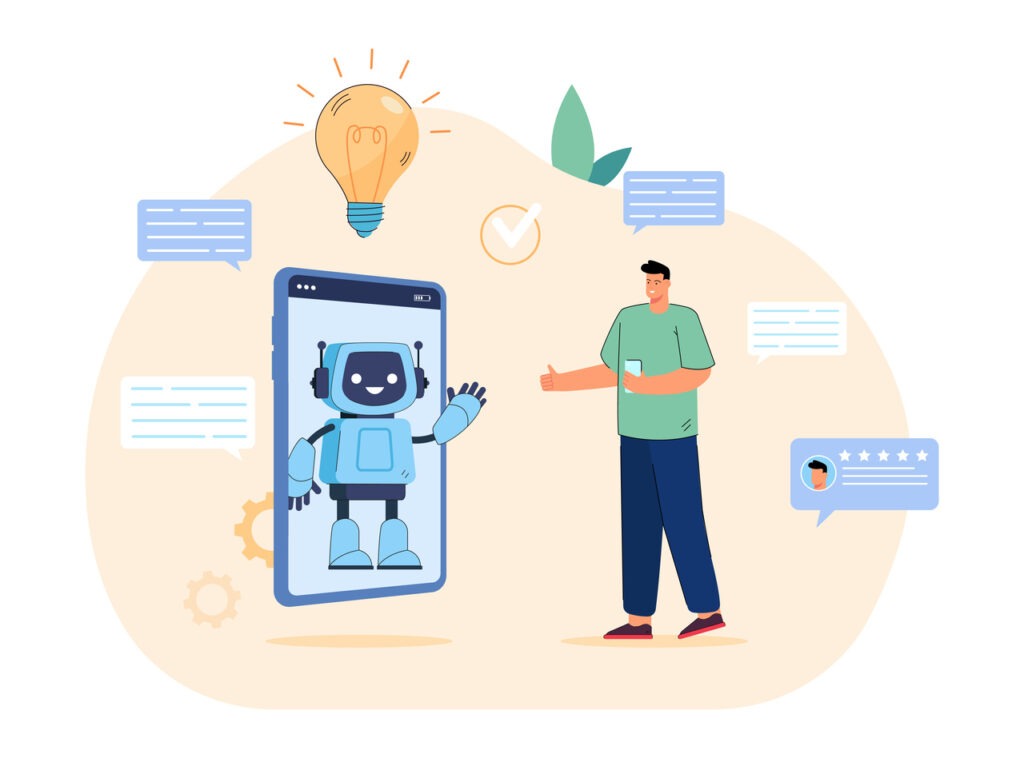
A Useful case of AI in data integration is empowering knowledge. AI-powered bots are becoming essential for accessing and managing organizational knowledge.
- Internal Documentation Bots: Picture an employee asking a chatbot, “What’s our company’s remote work policy?” The bot quickly pulls up the relevant information, saving time and reducing frustration.
- Knowledge Retrieval: AI can efficiently locate specific details within extensive knowledge bases. In customer service, this allows agents to find answers to complex questions swiftly, enhancing customer satisfaction.
3. Semantic Database Matching
AI is revolutionizing database matching by going beyond simple keyword searches and using semantic analysis to connect related data points.
- String Matching: AI algorithms can handle variations in spelling or formatting to match names across different systems. This helps link customer records, providing a unified view and preventing duplicate entries.
- Semantic Analysis: AI understands the meaning behind data, not just the words. This allows it to match concepts even when they’re expressed differently. For instance, AI can connect news articles about “climate change” and “global warming,” recognizing they refer to the same issue.
4. Email Management Gets Smarter
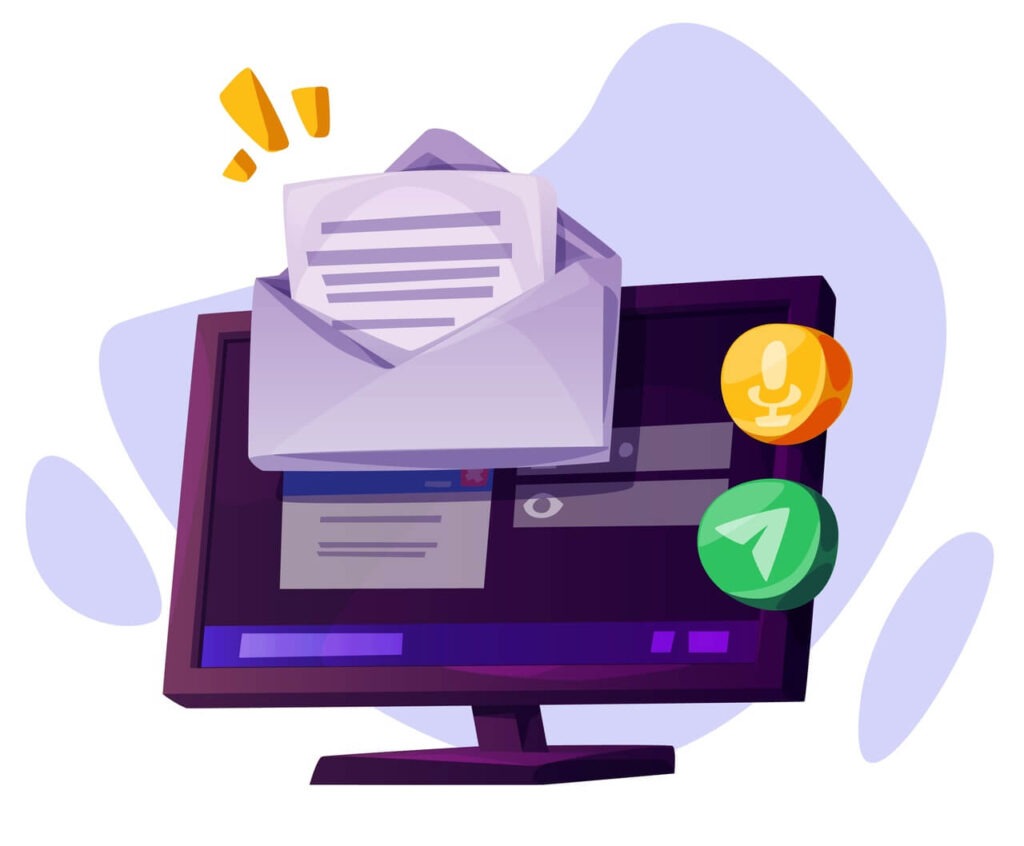
AI in data integration is also helpful for email management. AI is transforming email communication, making it more efficient and polished.
- Typo Correction: AI can catch and fix typos before you hit send, ensuring your emails are error-free.
- Tone Adjustment: AI can suggest rephrasing to make your emails sound friendlier, more formal, or more persuasive, depending on the context. This enhances communication and boosts your professional image.
5. Customer and Prospect Analysis
AI is improving the process of discovering and comprehending new clients.
- Lookalike Analysis: By evaluating current customer data, AI may find “lookalikes” – people who have similar traits to your greatest consumers. This enables marketers to target their efforts more efficiently.
- Behavioral Insights: AI can track client interactions across many channels (website, email, and social media) to create a complete profile. This enables individualized offers and recommendations, which increases client engagement.
Obstacles to Effective AI Data Integration
Integrating data from relational databases, streaming services, and other real-time sources has proven increasingly difficult for enterprises. To find important insights, well-designed data integration procedures must guarantee data is regulated, governed, and trusted. However, several challenges can hinder effective data integration, including:
- Manage data from multiple sources.
- Deal with various data integration tools.
- Navigate a business environment where multiple handlers can access, edit, copy, and duplicate data.
- The slow movement of data from data lakes and the Cloud.
To address these challenges, firms must begin to perceive data as a corporate asset, rather than a byproduct. Beyond design considerations, employing sophisticated technologies such as AI has proven helpful in addressing many of the major issues connected with large-scale data integration.
Choosing the Right AI Data Integration Solution
AI-powered data integration may raise some challenges. So it’s crucial to select the right data management tool to handle today’s complex data landscape.
Beyond AI capabilities, a top-notch data integration solution should offer:
- A user-friendly interface for designing and managing data pipelines.
- Powerful processing skills for dealing with large volumes of data in multiple forms.
- To secure sensitive information, use robust security measures such as encryption, access limits, and data masking.
- Broad interoperability with a diverse set of data sources, including cloud platforms, databases, and APIs.
Seeking a data integration solution that can significantly streamline your workflows and accelerate your data initiatives? Contact us now via sales@dgroup.co or Estimate Project.
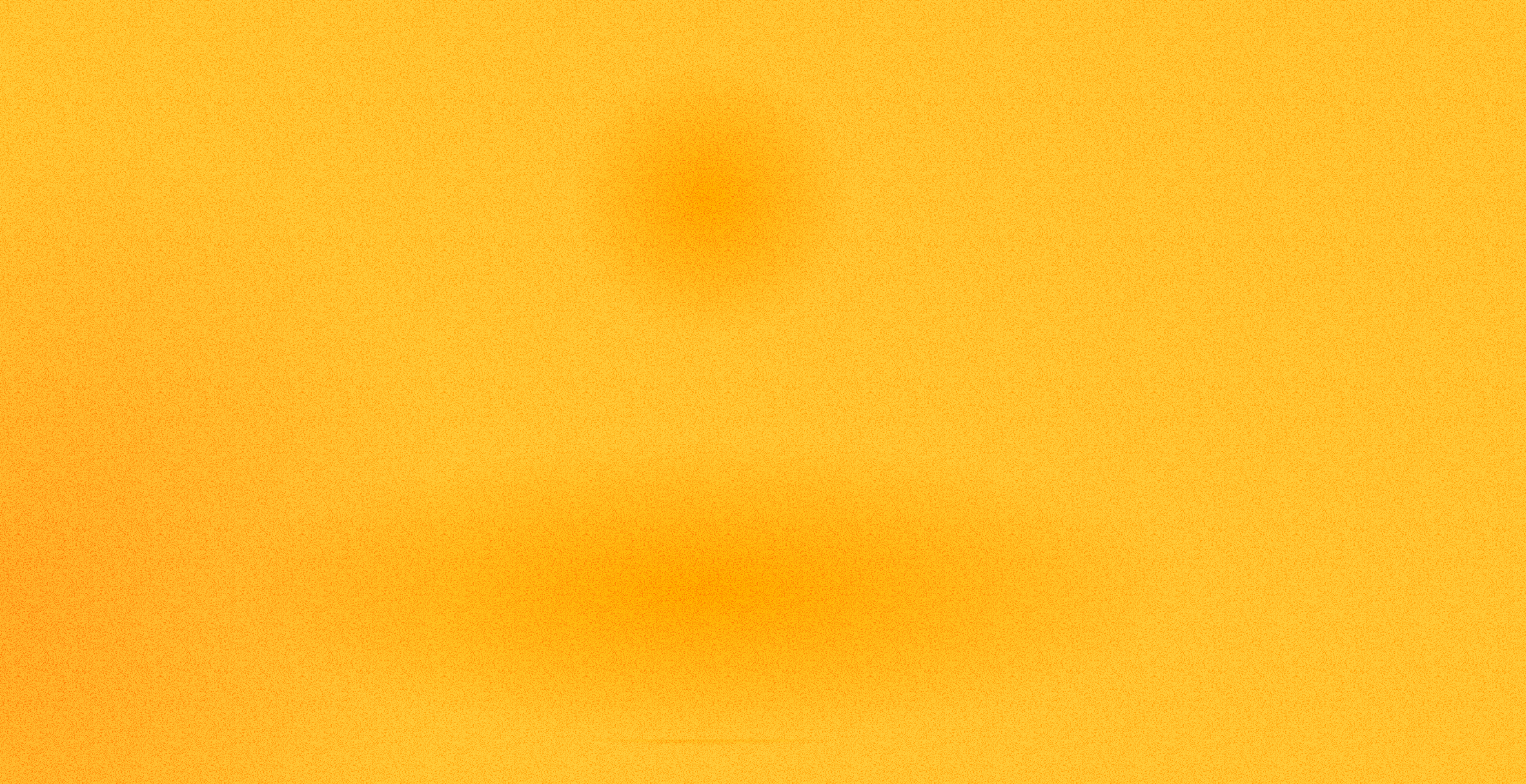
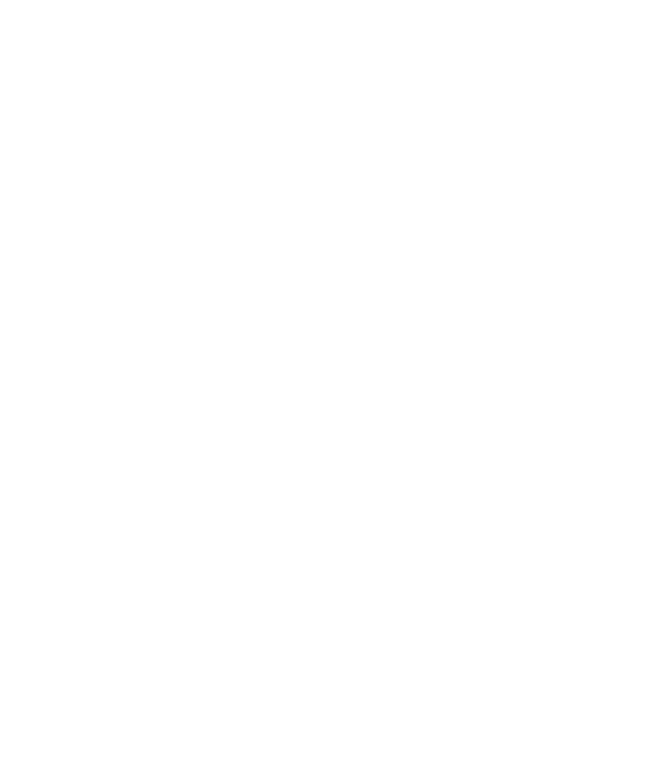
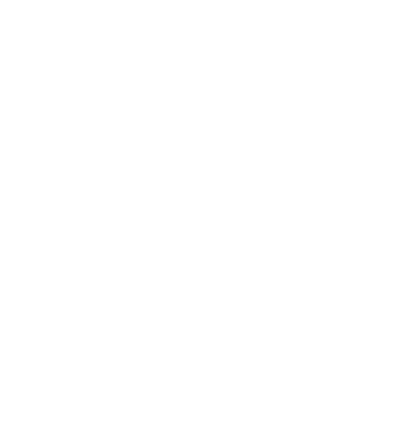
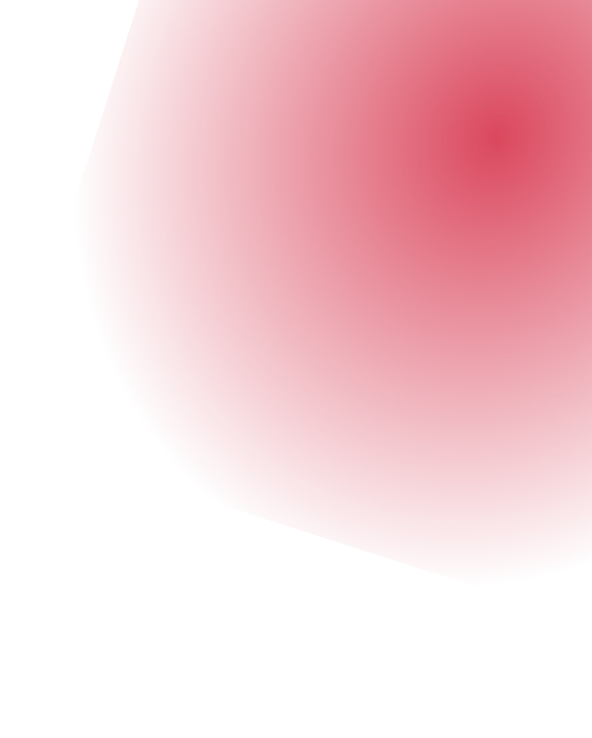
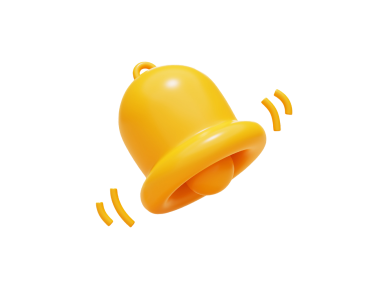
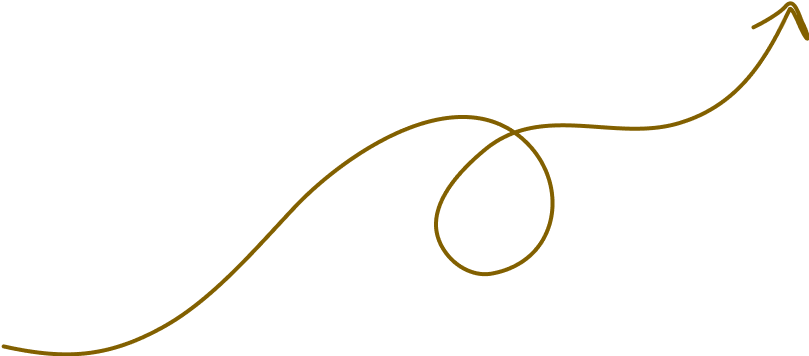