AI-Driven Decision Making: Pros, Cons & Examples
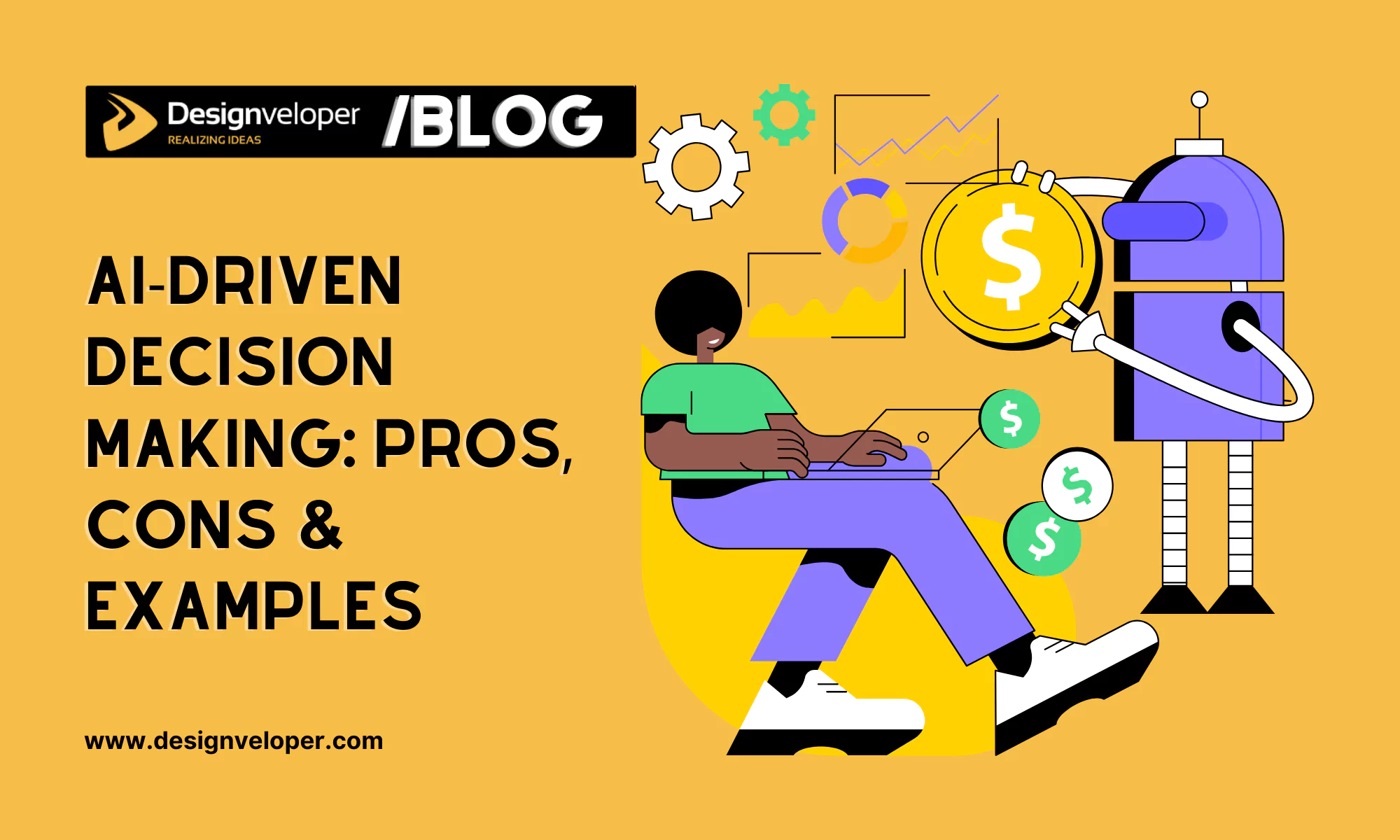
Artificial Intelligence (AI) has become popular in recent years with its transformative impacts. It can translate texts, write code, edit images, and noticeably, assist us in making business decisions. AI is being widely used for decision-making processes not just because of a higher demand for automation. But it helps us remove our inborn limitations from these processes, like cognitive bias or lack of ability to process huge datasets. To better understand AI-driven decision making, let’s take a deep look at how it works, which benefits and challenges it can present, and what applications it has.
Understand AI-Driven Decision Making
Firstly, we’ll learn about what AI-driven decision making looks like in reality.
What Does It Look Like?
How can AI support decision-making processes? There are two common models to illustrate how this advanced technology works as follows:
Without Human Intervention
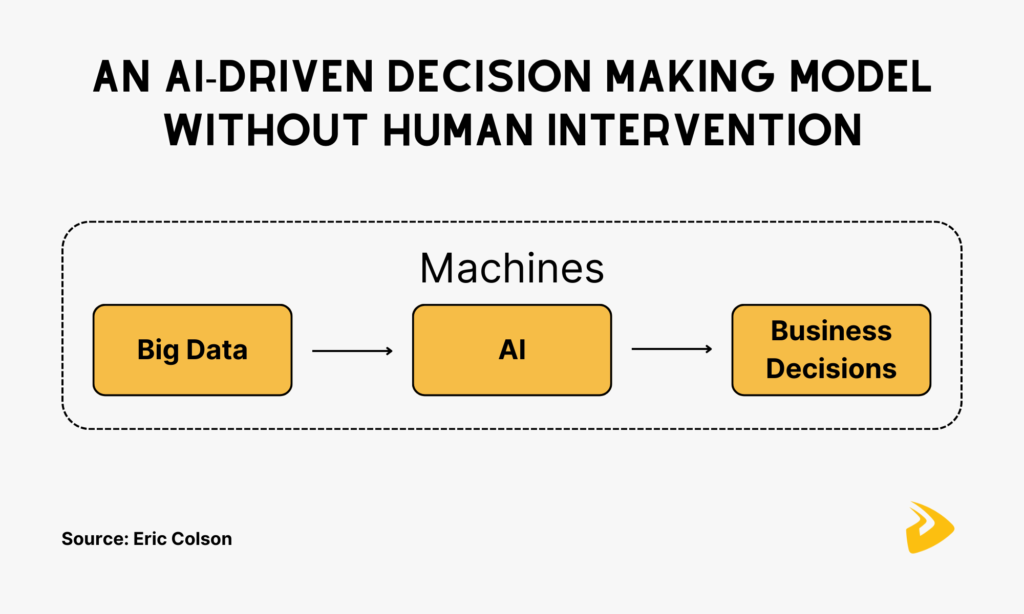
Eric Colson, Advisor at Activation Fund, developed an AI-driven model to handle regular decisions that primarily depend on clear and structured data. This model removes the human factor from direct decision-making as AI can process vast volumes of structured data (e.g., financial data or CSV files) better than humans.
Particularly, AI can recognize subtle patterns that human analysts might miss. It can even discover the non-linear relationships of data variables (e.g., exponential or binomial distributions) without being affected by cognitive biases. This helps it make better use of datasets and deliver more accurate decisions.
One typical application of this model is in fraud detection. Normally, AI systems process structured data such as account details, transaction logs, and historical patterns to spot suspicious spending activities and warn of possible fraudulent transactions. They also automatically block transactions that meet specific thresholds (like a sudden spike in purchases from an unusual location).
With Humans in the Loop
In reality, many business decisions rely on not only structured data but also other factors including:
- Unstructured data: Technological advancements like natural language processing (NLP), computer vision, and deep learning allow AI to process unstructured data (e.g., social media posts or phone calls). AI sometimes struggles to process unstructured datasets that have complex contexts or cultural nuances.
- Intangible factors: Important things like company values or strategy often lie outside the scope of AI’s analysis because of their subjective nature, rapid change, and reliance on human judgment.
- Ethical considerations: If your business has to make decisions related to long-term visions, social responsibility, and ethical dilemmas, human engagement is a must.
As AI can’t access and process well, humans need to get involved to validate AI-generated solutions and deliver relevant, accurate decisions.
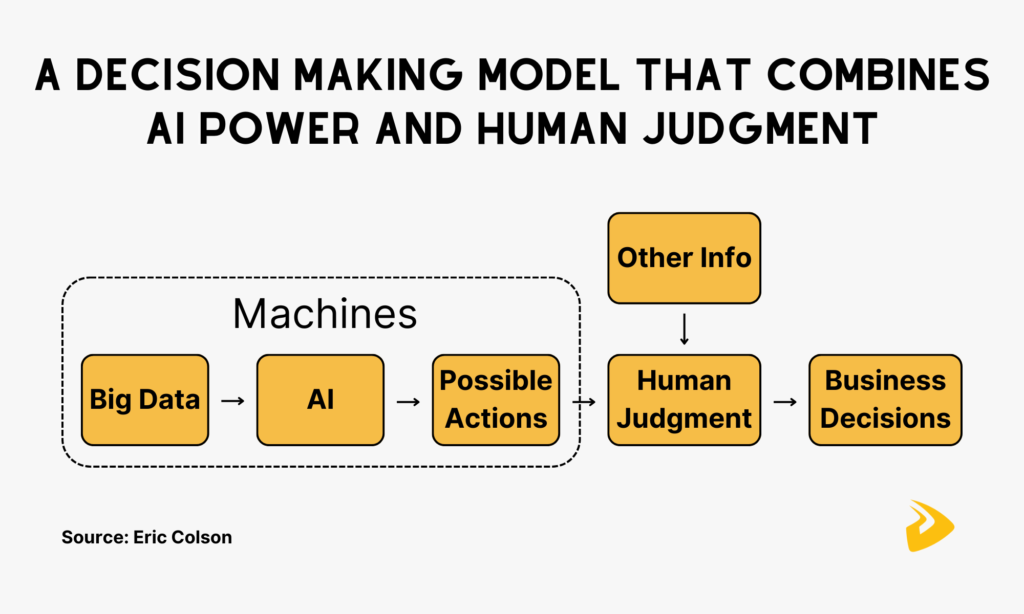
How? Let’s take a look at our guidance and example below:
- Big Data Collection: Gather vast volumes of structured data.
- AI Processing: Analyze big data to discover patterns, trends, and relationships that can be invisible to human analysts.
- Possible Actions: Recommend possible solutions or actions to specific challenges.
- Human Judgement with Other Information: Human analysts review AI-generated suggestions. Combined with other essential factors like contextual and ethical issues behind, they can assess whether these solutions are relevant and suitable.
- Business Decisions: Humans make the final decisions based on their judgment and AI-generated insights.
For example, AI can recommend spending more on marketing to reach the highest return on investment (ROI). But your company may consider maintaining quality standards, hence deciding to slow growth. Similarly, AI can suggest the optimal inventory level to maximize your profits. However, due to the current market’s competitive nature, you still choose to keep extra inventory for a better customer experience, even when this translates to lower profits.
Why Should You Bring AI to Decision-Making Processes?
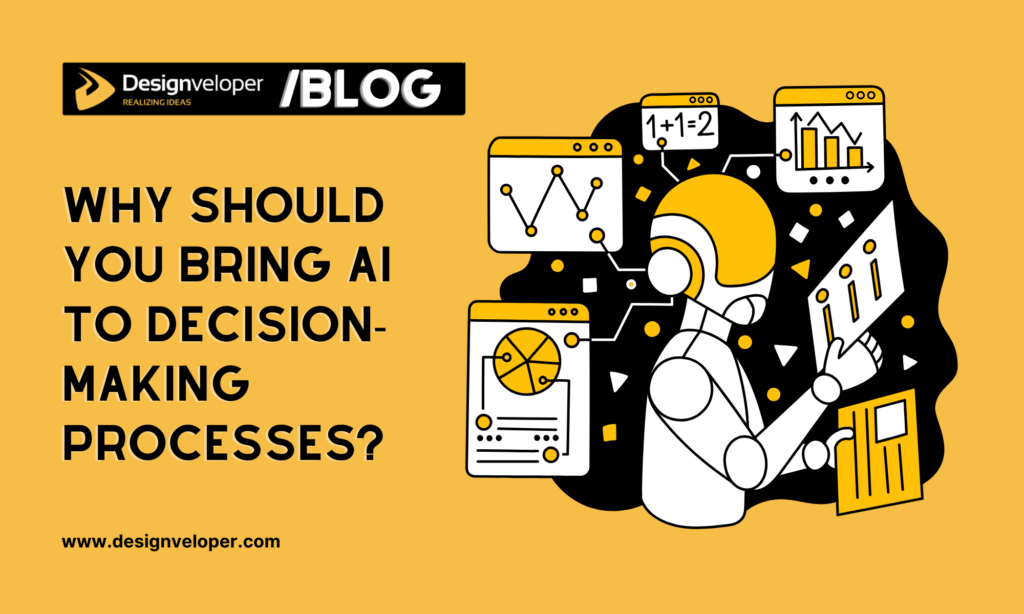
AI is being increasingly used for decision-making across industries. One research has shown that the global value of decision intelligence will reach $16.34 billion in 2025 and continue to increase to $18.62 billion in the next decade. This is not a surprising finding.
Many companies realize AI’s huge potential in helping them stay agile and adaptable to swift market changes. As already said, AI – especially its latest version, generative AI – can analyze a massive volume of structured and even unstructured data faster than humans. They can identify fine patterns that uncover unexpected groupings. These groups might be identified by a complex combination of factors human analysts never think of.
For example, AI can discover seemingly unrelated factors that define patients with an extremely high risk of developing severe Diabetic Retinopathy. These factors can be:
- Lifestyle patterns (those having specific dietary habits or exercise routines);
- Geographic location (patients living in areas with significant air pollution levels);
- Socioeconomic factors (those with low socioeconomic status undergoing higher stress levels, thus influencing blood sugar control).
With this capability, AI helps your company better understand datasets and make timely decisions while improving operational efficiency. Beyond that, AI-driven decision making comes with other impactful benefits:
- Reduce human errors and costs. By automating repetitive tasks (e.g., data entry or report generation), AI can minimize human errors and costs related to waste or rework.
- Boost employee productivity. AI’s automation capability frees up humans to focus on more creative and strategic initiatives. Plus, it can analyze data to find the best way to allocate resources (e.g., budgets, time, or staffing), consequently avoiding unnecessary expenses and optimizing employee workflows.
- Make predictions. AI can analyze historical data and discover patterns that predict future outcomes or trends (e.g., forecasting equipment breakdowns).
10 Real-World Examples of AI in Decision Making
With its huge potential, how do organizations worldwide leverage AI for decision making processes? Let’s look at a detailed list of ten real-world examples in this section:
1. Walmart – Retail Demand Prediction
Walmart employs AI-driven predictive analytics to forecast customer demand. By analyzing historical sales data, local events, and even weather patterns, they can swiftly anticipate which products will sell more in particular locations. This allows them to optimize inventory levels and avoid shortages or overstock.
2. Designveloper – Project Management
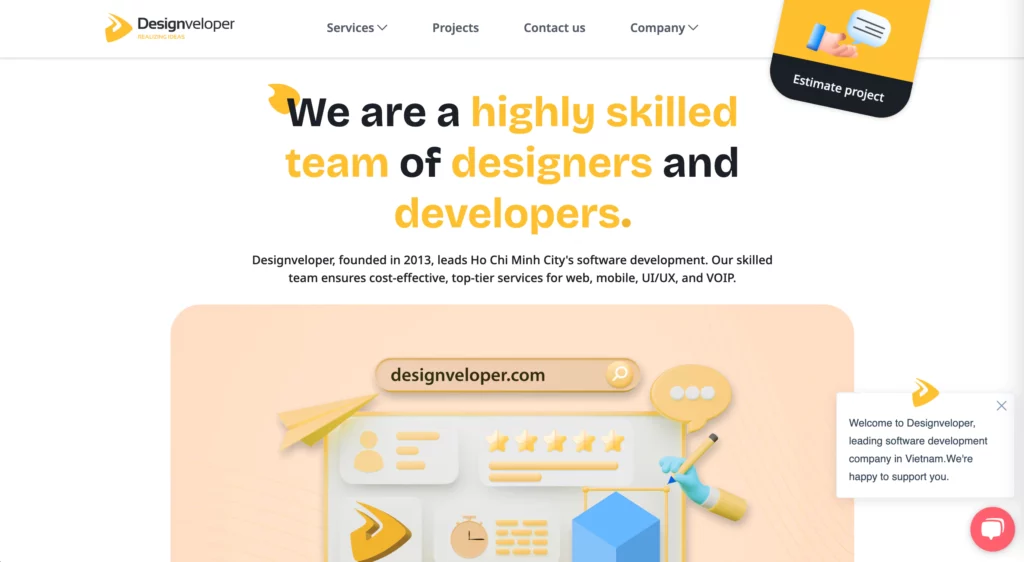
At Designveloper, AI serves as a versatile assistant who helps with effective project management.
AI-powered software like Autogen or Devin AI helps us forecast work progress, identify which tasks should be prioritized, and manage a project to address a specific client requirement. Such AI tools have already drawn a framework and estimated tasks and provided a working Proof of Concept (PoC) to help us envision the big picture of our projects better.
However, we realize that these tools are still at an early age, so containing many flaws that lead to poor or unsuitable outcomes for the client’s initial requirements. That’s why we just consider AI-generated results as a reference and then hold discussions to improve our project management process.
3. Netflix – Recommendation Engine
Netflix leverages AI to suggest movies and shows to viewers. By analyzing viewer interactions (e.g., viewing patterns, watch history, and ratings), Netflix’s recommendation system can personalize content displayed on each individual user’s page. For instance, if you frequently watch rom-com series produced in Korea, Netflix’s AI system will prioritize suggesting similar genres or trending content that fits your preferences.
4. JPMorgan Chase – Trade Optimization
JPMorgan Chase developed LOXM, an AI-powered platform, to assist users with executing equity trades automatically. This AI system uses machine learning and reinforcement learning to analyze large volumes of data and forecast price changes. As trained on simulated and historical trading scenarios, LOXM can conduct trades that precisely align with real-time market conditions. This helps traders manipulate timely execution and ensure the best prices while reducing market impact.
5. PayPal – Fraud Prevention
PayPal offers intelligent systems – Fraud Protection Advanced and Chargeback Protection – to protect businesses from fraud. The former system uses advanced machine learning and analytics to automatically assign a risk score to every transaction based on your preset filters. This allows your business to manage risk proactively and effectively. Similarly, the latter helps evaluate whether real-time transactions on both credit and debit cards commit fraud.
6. Uber – Ordering Experience
Uber developed Michelangelo, a proprietary machine learning (ML) platform, to make business-critical decisions like ETA (estimated time of arrival), Eats home feed ranking, rider-driver matching, and fraud detection. Below is how real-time ML powers user flow in Uber’s Rider and Eats apps.
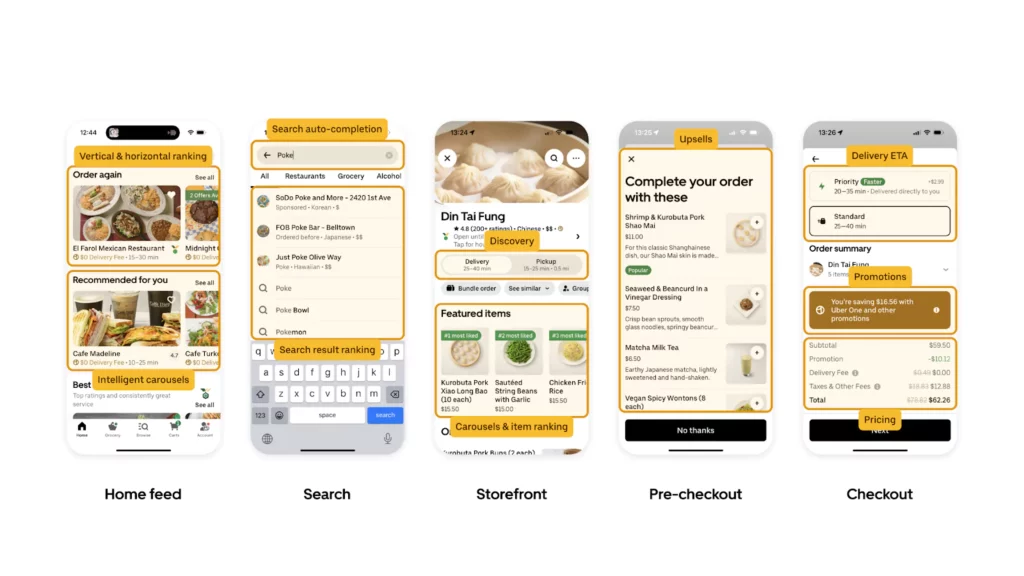
For example, in the Eats app above, Michelangelo analyzes user preferences, interactions, and order history to prioritize frequent orders (in the “Order again” category) and tailor recommendations (in “Recommended for you”). It also uses NLP and machine learning to forecast what users are about to type and rank search results based on their habits, relevance, or popularity.
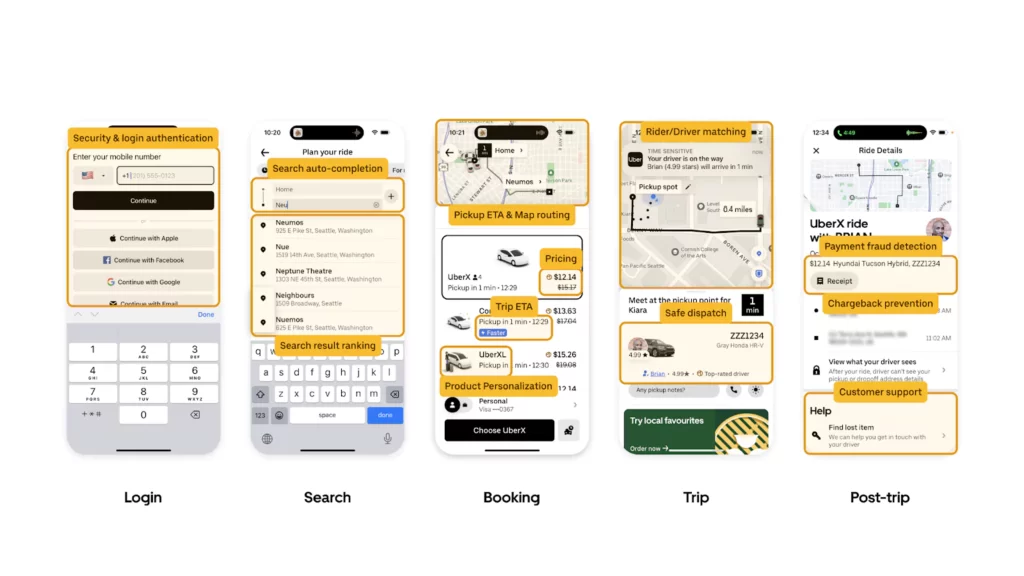
On the other hand, Uber’s Rider app uses ML to spot fraud signals when users try to log in and make payments. ML also helps recommend destination auto-completion, calculate ETA, compute possible trip prices, and pair riders with drivers in a way that prioritizes safety yet ensures effective service.
7. Cleveland Clinic – Patient Care
Cleveland Clinic developed a wide range of AI tools to support its operations. One of them is the Clinician Insights Platform. By analyzing physician notes and patient data in real-time, it helps identify possible diagnoses and treatment plans, as well as tailor recommendations for each patient based on their unique medical history.
Cleveland also uses another ML-powered tool called the Clinical Pathways Program to create personalized treatment plans for cancer patients. Additionally, Cleveland implemented AI predictive analytics software to determine patients at risk for readmission.
8. Amazon – Supply Chain Optimization
Amazon uses AI in different aspects of operations, and one outstanding application is optimizing its supply chain. By analyzing many factors like road closures, traffic jams, or weather conditions, AI can plan the most optimal routes for delivery drivers. Moreover, AI can predict daily demand and forecast where these orders may come from. This enables quicker delivery, especially during the holiday season.
Amazon also developed the Packaging Decision Engine (PDE) system to optimize and accelerate packaging tasks. The PDE leverages NLP and historical data to analyze new text-based information from each product (e.g., name, price, description, or package dimensions) and identify the suitable packaging type.
The retailer also launched Project P.I. to detect defects. This AI model uses generative AI and computer vision to determine whether product specifications (e.g., size or color) are correct before they reach the end users.
9. General Electric (GE) – Energy Management
General Electric developed AI-powered tools to support its clients in making decisions associated with energy management. One noticeable invention is Proficy® for Sustainability Insights. This software solution helps industrial companies manage equipment performance, energy consumption, and climate metrics required for compliance. By analyzing operational and sustainability data, it can identify which areas need to improve for energy efficiency, reduce waste, and minimize emissions.
10. Greyparrot – Waste Management
Greyparrot developed an AI-powered system to help recycling facilities make more efficient decisions in waste management. The system analyzes data transmitted from cameras that track waste materials on conveyor belts. With customizable dashboards that present real-time insights, it helps operators spot issues and manage equipment effectively.
Challenges With Artificial Intelligence
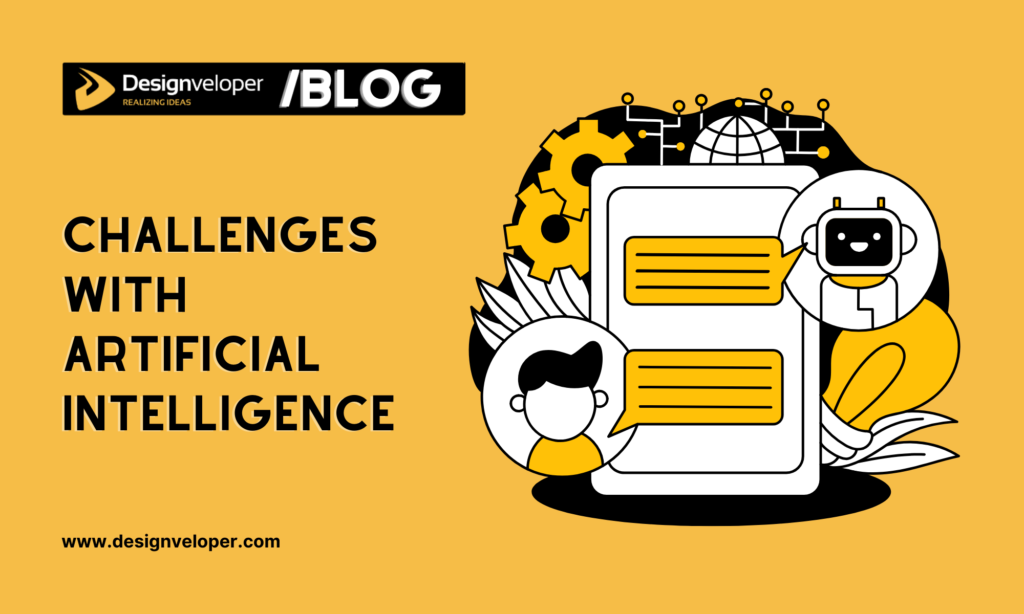
AI still presents some potential challenges for decision making. Below are some problems you should be cautious about:
Data Bias
Unlike humans, AI isn’t impacted by cognitive biases. But there’s still a real risk of AI producing unfair or misleading outcomes. We all know AI works primarily on data. If the training data contains biases, no wonder that the model can reflect those biases. There are many reasons behind this.
- The way data is collected or used can introduce biases. For example, if your survey unfairly excludes specific demographic groups, the resulting data may not represent the entire population. As a result, AI models trained on this data can make unfair decisions associated with those groups.
- Data itself is biased due to scarcity or historical inequities. Suppose there’s a scarcity of data related to individuals with darker skin tones in the training datasets. So, an AI-powered makeup app has difficulty suggesting a suitable makeup look for this group.
Ethical Concerns
AI models depend on vast amounts of data for decision-making processes. But this raises concerns about privacy violations and data security. For instance, the collection of personal data for facial recognition in surveillance can violate individual privacy rights. Besides, many individuals may not fully be aware of how AI collects, shares, and uses their data. This can erode their trust in organizations using AI to gather their information.
Lack of Transparency and Explainability
Many think that AI-driven decision making lacks transparency because they don’t know how AI systems come to a specific decision. This is often considered a “black box” problem where the inner workings of AI algorithms, especially complex ones like Convolutional Neural Networks (CNNs), are hard to explain.
In high-stakes fields like healthcare, finance, or legal systems, trust and accountability are crucial. So, understanding how AI systems produce certain outcomes is necessary. For instance, if an AI model suggests a particular medical treatment, physicians need to know why in order to maintain fairness and adherence to regulations.
Conclusion
Now, your journey of discovering AI in decision making comes to an end. Through this article, we expect you to have a better understanding of how AI-driven decision making looks, coupled with its immense benefits and outstanding applications. However, AI itself comes with several challenges including data bias, lack of explainability, and ethical concerns. This requires human involvement to ensure AI-generated outcomes are accurate and trustworthy. So what about you? Are you using AI in daily decision-making processes? Share with us on Facebook, X, and LinkedIn!
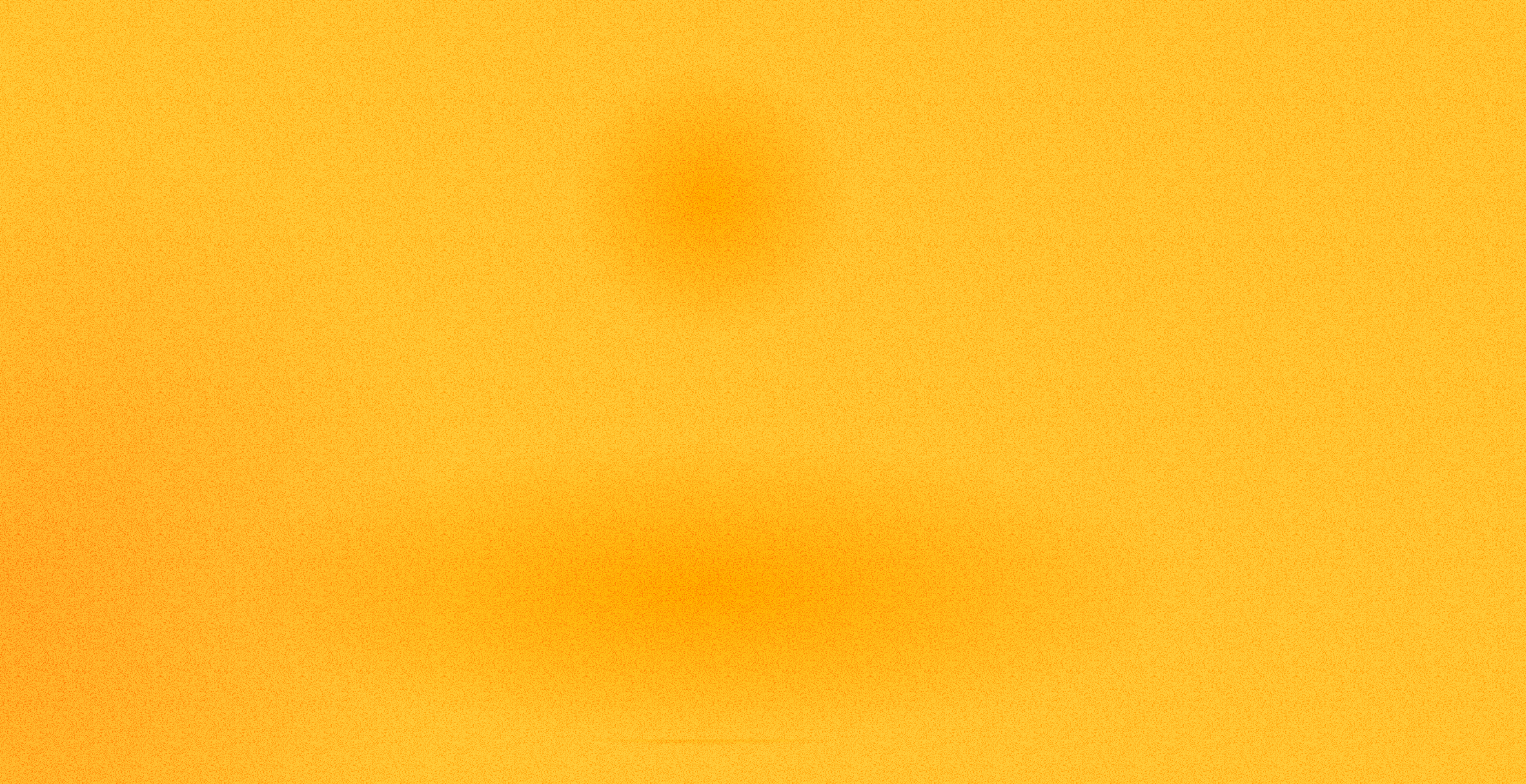
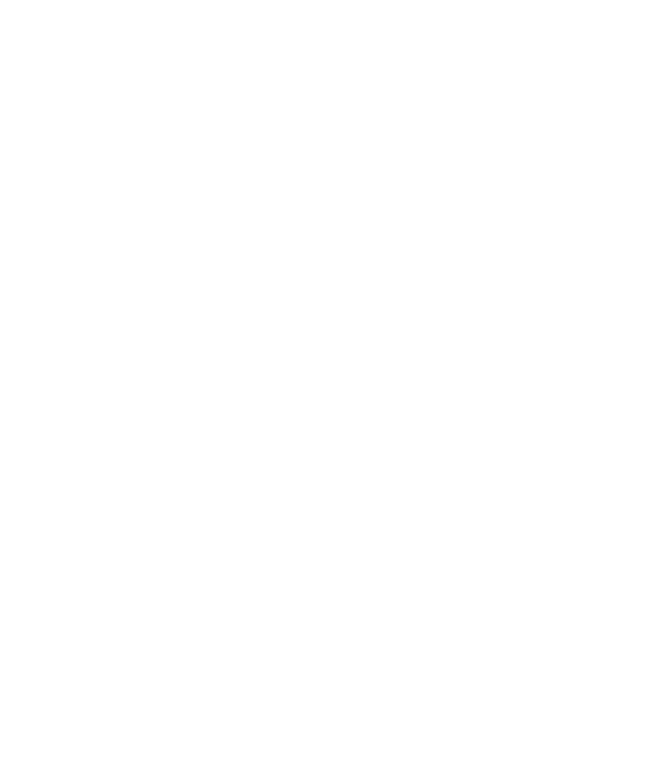
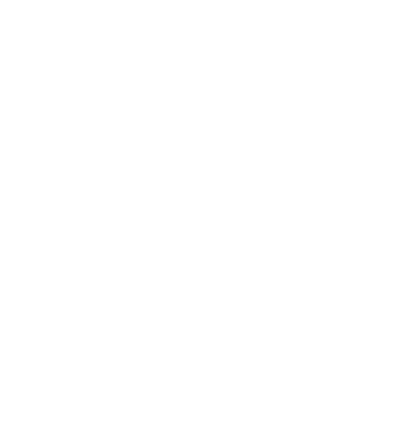
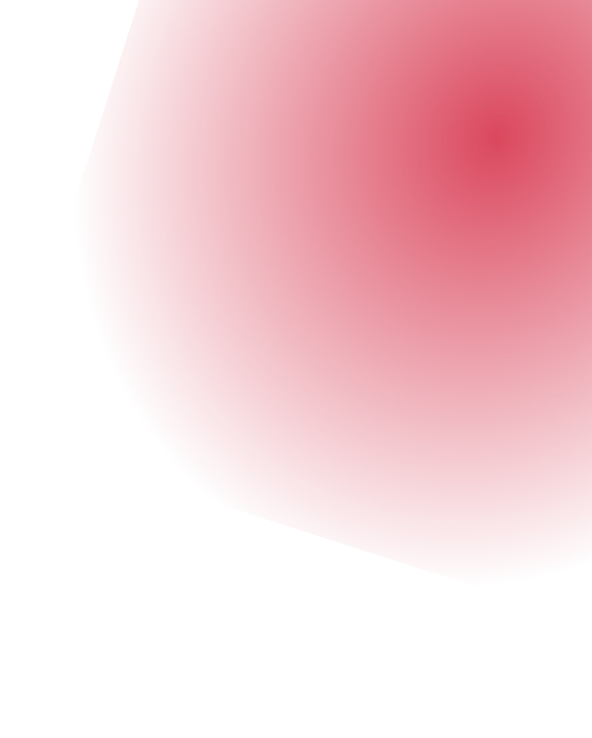
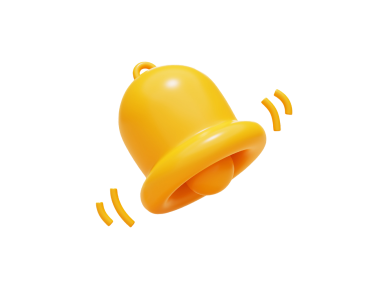
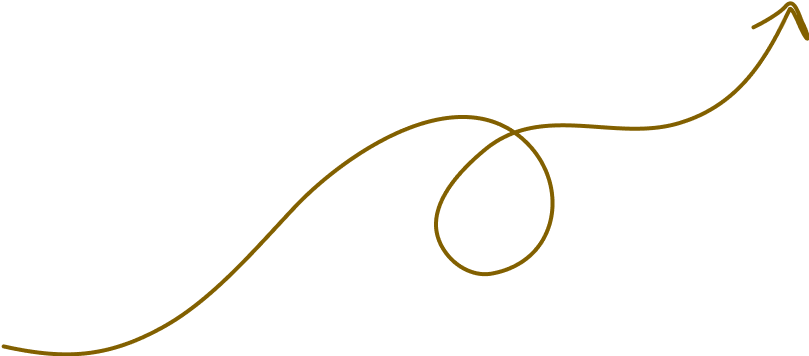