AI in Finance: A Guide to Applications, Benefits, and Future
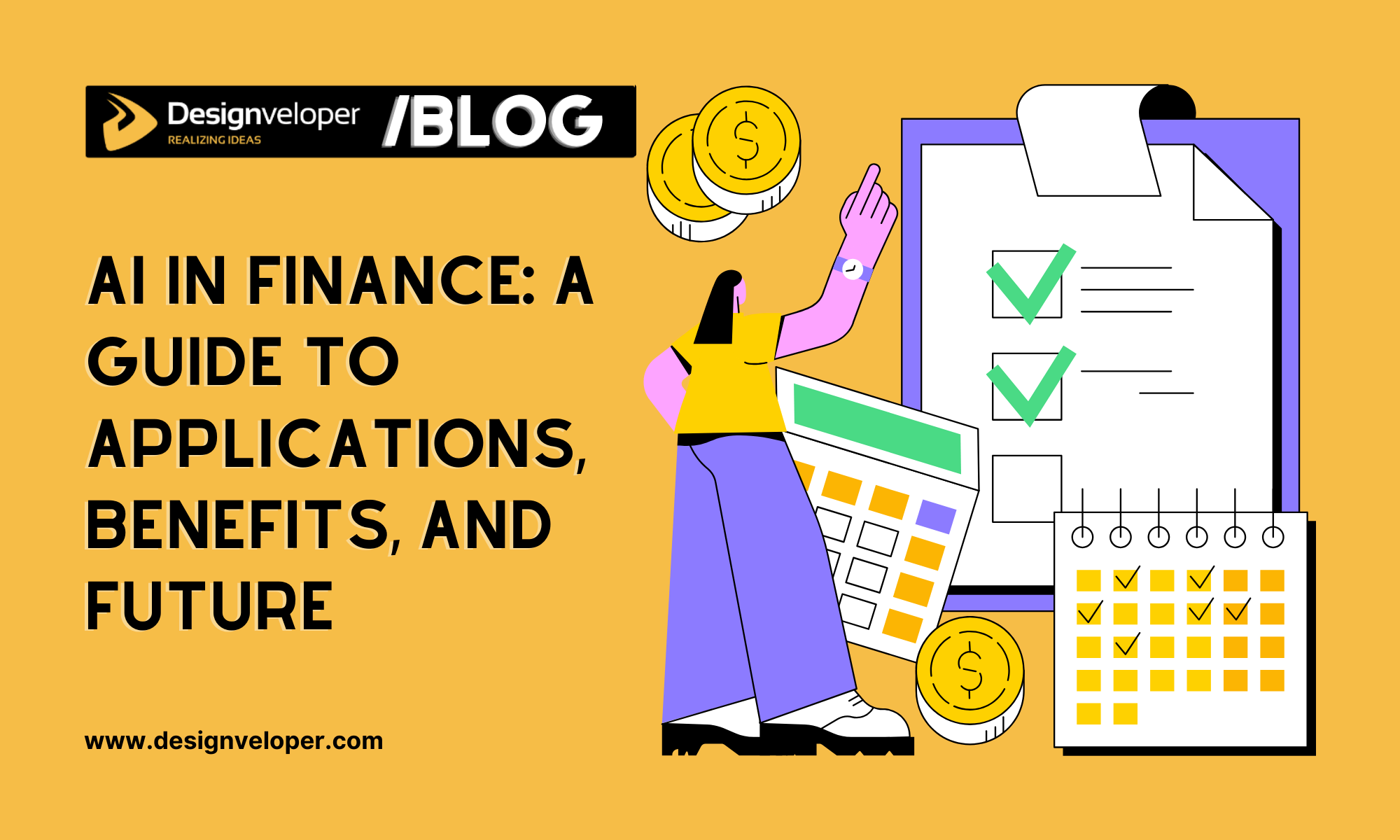
Are you tired of manually retrieving and analyzing customer data from different sources for financial advisory or risk assessment? Don’t worry because you now have AI as a powerful assistant to handle these tasks quickly and effectively. So, how exactly is AI being used in finance? What benefits can your company reap from AI? Are there any challenges you may encounter when adopting this advanced tech? Understanding all these things is crucial if you want to stay ahead of the curve in this AI-driven era.
What is AI in Finance?
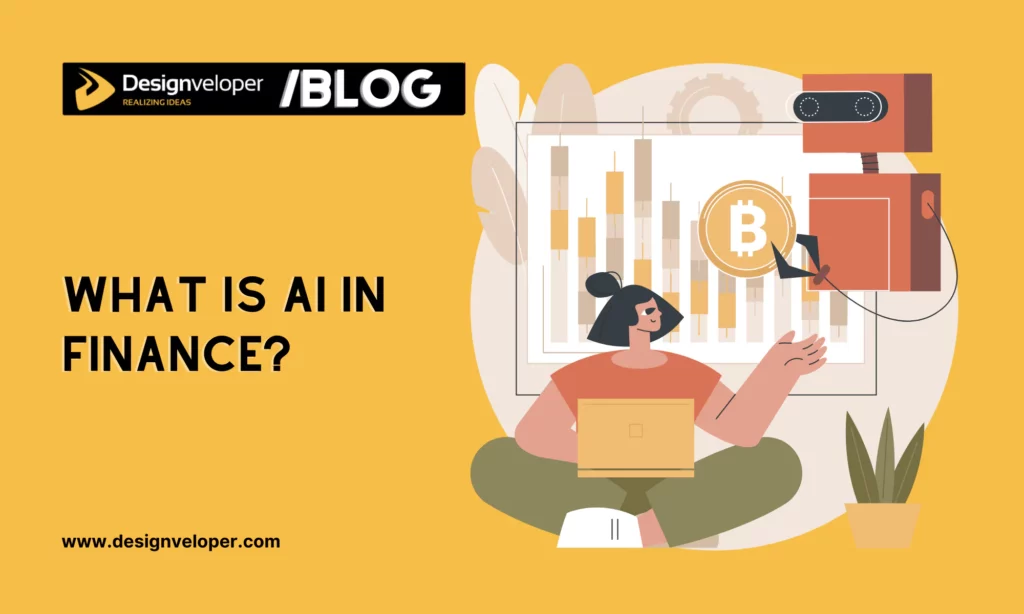
AI in finance refers to the use of artificial intelligence to process vast amounts of data. Such data might include transaction information (e.g., records of purchases or payments), customer information (e.g., credit scores or spending habits), market data (e.g., interest rates or stock prices), risk data, (e.g., loan performance or potential financial risks), and operational data (e.g., audits or regulatory reports).
With AI handling this task, you can streamline financial workflows like automating data analytics, managing risks, and making informed decisions. Further, you can leverage AI-powered chatbots to give customers faster answers 24/7 or generate financial reports in a second.
For this reason, AI can help your company work smarter and more effectively while providing better financial services.
Machine Learning in Finance
As a subsegment of AI, machine learning (ML) is widely used in financial systems to advance decision-making and predictions. Particularly, it uses algorithms that enable financial software to learn from data and automate some specific tasks. These tasks involve spotting fraudulent activities through pattern recognition, forecasting market trends, automating credit scoring, and customizing financial products.
10 Key AI-Driven Applications in Finance
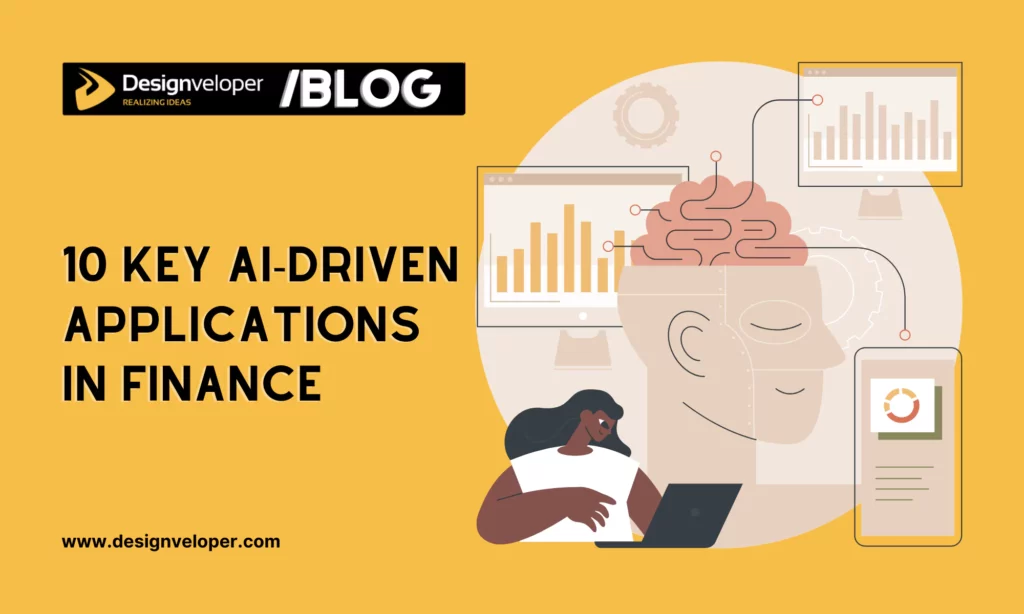
We’ve briefly mentioned what AI/ML is in finance. So, how exactly is artificial intelligence being used in financial systems? Let’s dive into its main applications in this realm:
1. Customer Support Through Chatbots
AI-powered chatbots can support human agents in offering quick customer questions. One typical example is Erica, a virtual assistant developed by Bank of America. Erica, accordingly, helps customers manage their finances through a mobile app. The chatbot uses natural language processing (NLP) to understand and answer customer questions 24/7. Also, it can provide budgeting advice, remind customers of upcoming bills, and report past transactions.
2. Fraud Detection & Prevention
AI can identify and prevent fraud in financial systems in real-time. Particularly, it may learn from past data about previous fraudulent activities, and then analyze transaction data or track user behavior to spot unusual patterns. American Express, for example, uses an advanced deep-learning model through the NVIDIA GPU computing platform to detect anomalies in transactions via Amex cards. This real-time fraud detection system helps Amex boost accuracy and protect its card users better.
3. Risk Management & Portfolio Optimization
By analyzing historical data, economic indicators, and market trends, AI can help you evaluate potential risks in investment portfolios. It can simulate different market scenarios to identify how these portfolios can perform under unexpected conditions (i.e., economic downturns). Plus, it may automatically allocate assets to balance risk and return based on investor goals and market conditions. Then, investors may make smarter decisions to manage risks better.
For instance, BlackRock developed Aladdin which integrates data analytics and risk management tools to streamline its portfolio management. This AI-driven platform can analyze vast data predict market shifts and assess the possible risk/return rate of various investment strategies. For this reason, it can help investors automatically optimize portfolio allocations and achieve better financial results.
4. Personal Recommendations
AI plays a crucial role in tailoring financial advice and investment products. Accordingly, AI algorithms can analyze vast datasets (like investment preferences, transaction history, or risk tolerance) to automatically recommend financial services or products that align with individual needs. Several AI models may even adjust recommendations in real time according to market shifts, changing client requirements, or new financial offerings.
Betterman, the world’s leading robo-advisor, uses AI to create personalized investment plans for clients, whether they’re for retirement or wealth building. It also supports automated trading, rebalancing, and dividend reinvestment. Additionally, it leverages AI to allocate assets to investments that will generate minimum taxes.
5. Predictive Modeling
AI algorithms handle large amounts of customer information, trading data, and other data insights to make highly accurate predictions. These predictions may be helpful if you want to evaluate possible risks (e.g., market volatility or credit risks), future customer behaviors (e.g., spending habits or loan defaults), and fraudulent practices. Then, predictive modeling can support informed decision-making and portfolio optimization.
For example, Global Credit – a financial services company – utilizes DataRobot’s predictive AI platform to forecast loan defaults and spot unbanked individuals with a lower possibility of default. This helps the company boost its loan acceptance rate while keeping risk at the same level. Moreover, the company’s predictive model helps mitigate the resources needed to acquire former customers by predicting who is most likely to return.
6. Sentiment Analysis
Sentiment analysis in finance means using AI tech (like natural language processing) to analyze emotions and opinions from news, social media, financial reports, and even earning calls. This approach helps your company assess risks, anticipate market shifts, and spot possible trading opportunities. By understanding sentiment, you can improve decision-making.
Like at Goldman Sachs Asset Management (GSAM), AI powers sentiment analysis can detect small, insignificant signs from management during earnings calls. These signs may indicate how confident or worried management is about the company’s true prospects. This allows smarter investment decisions.
7. Document Processing
In finance, AI can process documents quickly and effectively by analyzing and extracting important data from documents (i.e., contracts or compliance reports). This helps minimize human errors and accelerate document processing while still ensuring a high level of precision.
For example, JPMorgan Chase develops COIN (Contract Intelligence) to retrieve and analyze data from legal documents like commercial loan agreements. Previously, this task required roughly 360K hours a year for completion. However, the introduction of COIN significantly reduces the time and operational costs needed for the document review process. Further, this AI-driven platform handles an increasing volume of documents accurately.
8. Algorithmic Trading
As the name states, this refers to the use of computer algorithms to execute financial trades automatically based on preset factors like stock volume or price.
Particularly, AI will analyze large amounts of data in real-time, spot trading opportunities, and initiate trades. This can minimize manual errors and enable high-frequency trading by capitalizing on short-term market inefficiencies. With this benefit, the value of algorithmic trading is expected to increase by 7% from 2024 to 2032.
Renaissance Technologies – a US-based hedge fund – uses its proprietary trading algorithms in its flagship fund, the Medallion Fund, to consistently get high annual returns (40%+ before fees).
9. Financial Operations
AI can streamline your financial operations by automating some tasks like transaction processing or financial reporting. This boosts operational efficiency and accuracy while enabling you to focus on more strategic activities.
For instance, DBS Bank has developed two internal AI-powered platforms to enhance its operations and decision-making. These solutions, ADA and ALAN, help DBS send out millions of monthly personalized suggestions that guide customers toward better financial planning or investment decisions. AI also speeds up the financing process and spots potential credit risks early on. Meanwhile, DBS Bank still ensures data governance, quality, and security.
10. Compliance Monitoring
The finance industry is very sensitive to data usage because of strict privacy regulations and the need to maintain customer trust. With AI, your financial institution can improve compliance monitoring and avoid unexpected compliance costs by spotting possible violations of these requirements. Treasury Prime, a San Francisco-based fintech company, uses Kobalt Labs’s AI-enabled platform to swiftly check vendor documentation against regulatory standards.
Pros and Cons of AI in Finance
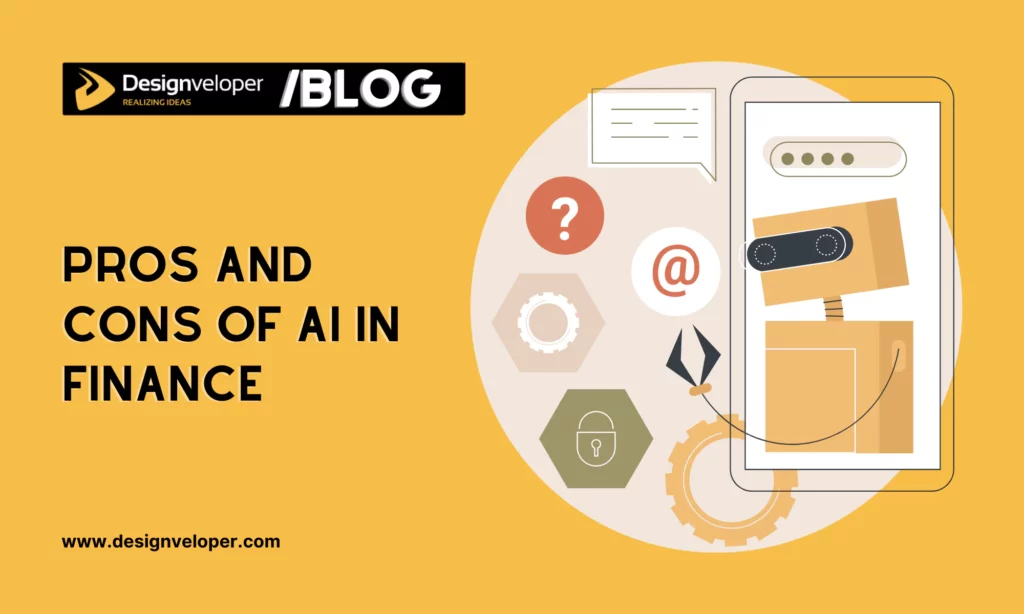
With all these key applications of AI in finance, it’s no wonder that this advanced tech can bring various immense benefits to your company:
Benefits of AI in Finance
AI has been reshaping the way financial institutions work and make decisions.
First, it boosts operational efficiency and accuracy (43%). Particularly, your financial institution has to process massive datasets daily. With the support of AI, this data can be handled at significant speeds while reducing manual errors.
Such data, accordingly, provides in-depth insights into customer behavior, financial transactions, market movements, and even patterns that may point out anomalies. This enables you to make prompt decisions, personalize financial offerings, and protect clients against fraud.
Also, AI can automate reporting, power customer support chatbots, and create tailored marketing strategies. All these things enhance customer experience (27%) and give your company a competitive edge over other peers (42%).
Second, AI reduces operational costs significantly (14%) by automating repetitive tasks like data entry or invoice processing. This allows employees to focus on more strategic activities, hence boosting resource allocation and productivity. Even when your company grows up, AI models can accordingly scale up to handle your growing demands. This removes the need for additional staff or complicated infrastructure, therefore maintaining low operational costs.
Finally, your company can improve decision-making through AI-powered data analytics. By digging into massive databases to retrieve impactful insights, AI can help your company evaluate risks, predict market movements, and optimize investment strategies. This enables informed decision-making and even opens new business opportunities (23%).
Challenges of AI in Finance
Besides optimistic signals, AI also brings about several challenges to financial institutions.
The biggest issue of AI in finance is related to data (38%). Such practical challenges as privacy concerns, data sovereignty, and data scattered across different sources make it hard to monitor, use, and analyze financial data effectively. This can deter AI models from performing well, hence leading to unreliable insights.
Further, AI systems use vast amounts of financial data which is considered highly sensitive. This might raise ethical concerns about how AI should use the data. Not to mention that 27% of financial institutions consider a lack of high-quality, diverse databases as another challenge to effective model training and accuracy. This enables your company to face biased or unfair outcomes if these results are generated from ineffective AI models. As a result, you may make wrong decisions, especially in areas like loan approvals or credit scoring.
How to Overcome Challenges
To address these hurdles of AI in finance, various financial institutions are planning or executing a framework to monitor the way AI is developed, trained, and utilized to comply with industry regulations (84%). They also leverage tools to verify and manage these frameworks for reliable and explainable AI (84%).
Plus, many start using federated learning to train AI models on a decentralized platform without delivering data. This allows AI models to be developed more securely. Additionally, they use confidential computing tech to create a secure environment for handling sensitive data. This allows them to meet compliance requirements like GDPR or CCPA.
Finally, no matter how intelligent AI is, it still needs human oversight in some cases. The bottom line is seeking the right balance where AI models improve accuracy and efficiency while human agents offer sound instructions to ethics and business priorities.
The Future of AI in Finance
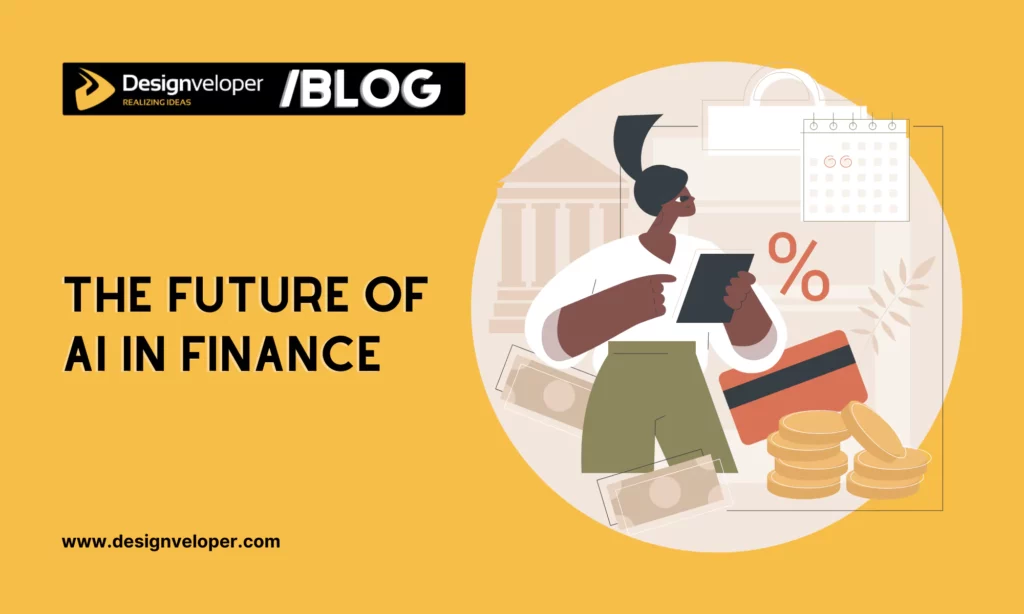
AI plays an increasingly crucial role in finance, particularly in the banking sector. In 2023, this sector recorded the highest AI investment (USD 20.6 billion) compared to the whole finance industry (USD 35 billion) and other industries. Besides, the value of AI in fintech will increase by 15.9% annually from 2024 to 2034. This underscores the potential for the financial industry to take advantage of AI to automate investing and banking services.
Accordingly, the future of AI in finance becomes more exciting with emerging trends:
- Generative AI: The launch of ChatGPT ignited interest in GenAI. This new tech can refine your FinOps by supercharging chatbots, simulating financial data & scenarios, estimating costs, generating financial reports & analysis, etc.
- Integration With Blockchain: AI can combine with blockchain tech to ensure more secure and transparent transactions. Blockchain maintains a decentralized record of all transactions, each of which is stored in a block and linked to a previous one to create a chain. This shared chain (“ledger”) allows everyone in the network to access and verify the transaction history without the need for a central authority.
- Quantum AI: Quantum computing refers to the use of quantum bits (qubits) to handle specific problems faster than conventional computing. AI models, combined with quantum computing, can implement data analytics faster while significantly enhancing cybersecurity. Accordingly, quantum AI is widely used to detect fraud, mitigate risks, and optimize portfolio performance.
- Test With Regulatory Sandboxes: Regulatory sandboxes are controlled environments where your financial organization can test new AI models under the supervision of authorities. It gives a secure place for experiments while ensuring regulatory compliance.
How Designveloper Can Help with AI in Finance
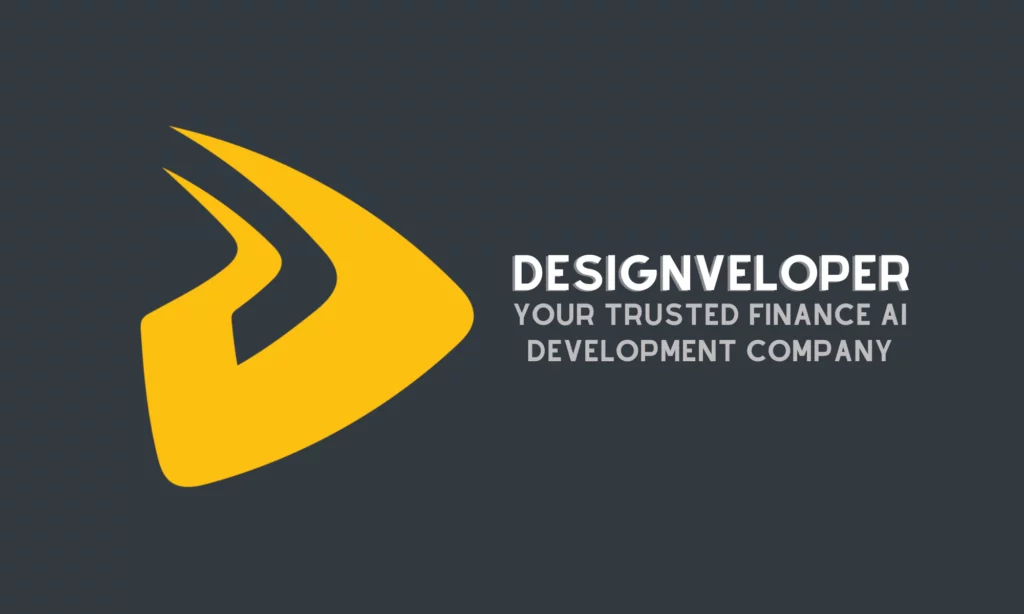
We can’t deny the huge potential of AI in today’s financial landscape. Besides the popular AI use cases in finance we mentioned, financial institutions are looking for opportunities to adopt this modern technology in additional financial aspects. However, along with its immense benefits, AI also poses several challenges. This requires your company to be well-prepared to overcome these problems and capitalize on AI to the fullest.
So, if you’re looking for a reliable, professional partner for AI fintech projects, Designveloper is here to help! With over a decade of experience in software development, our teams have implemented 100+ projects with 50 technologies applied. One of our notable projects is Song Nhi – a virtual financial assistant that allows users to manage personal cash inflow and outflow easily.
Here, we offer a wide range of AI development services:
- AI Software Development: We create cutting-edge solutions customized to meet your specific business needs. By using AI’s latest advancements, we deliver robust and scalable software that drives efficiency and innovation.
- Generative AI: We harness the power of advanced algorithms to develop innovative and dynamic GenAI solutions that inspire and engage your end-users.
- AI Chatbot Integration: We provide top-notch AI chatbot integration services to revolutionize your customer engagement. Accordingly, our advanced solutions smoothly integrate AI tech into your existing platforms. No more mundane tasks! Instead, your company can improve efficiency, streamline communication, and boost user experience with our tailored chatbots.
So why wait? If you have any AI fintech ideas, contact us and discuss more!
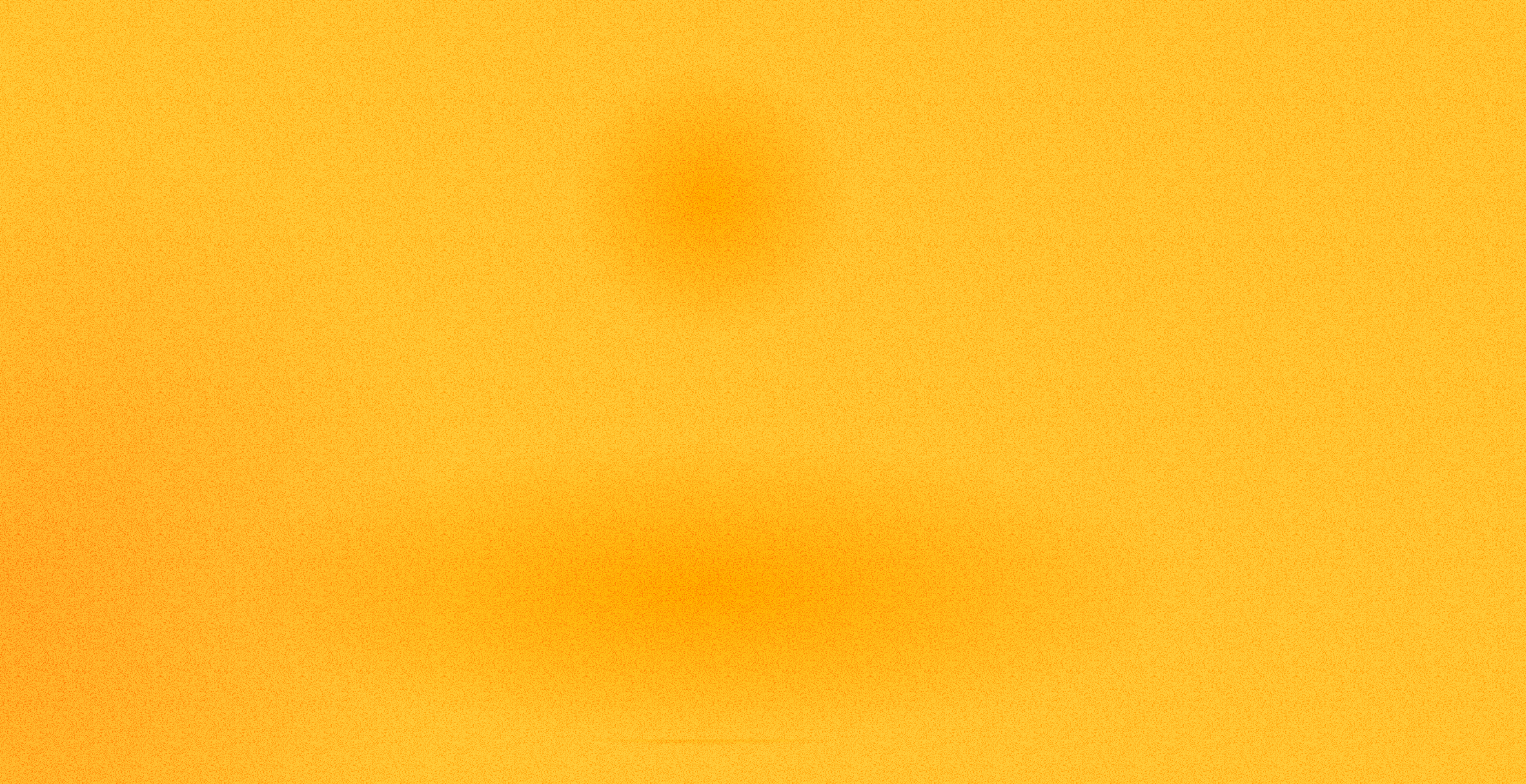
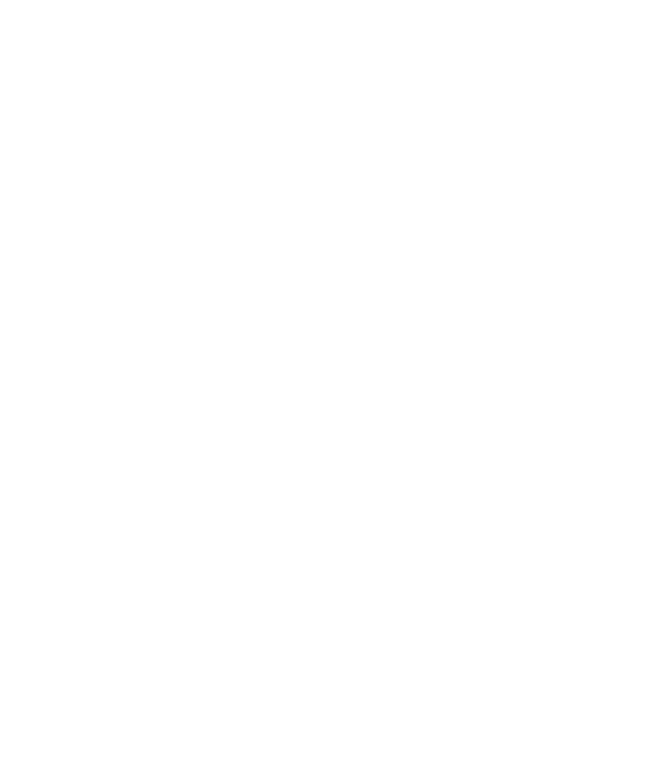
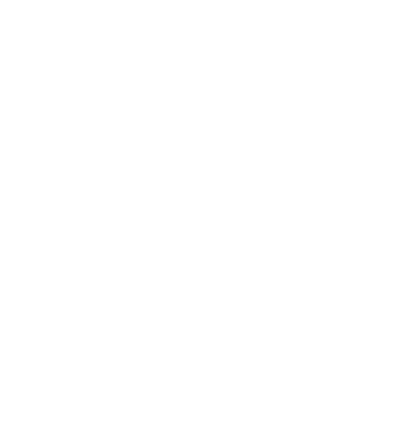
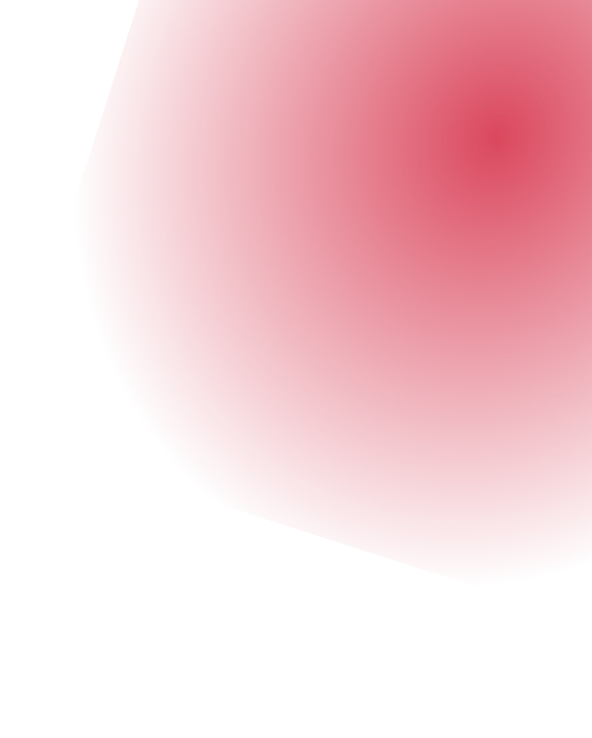
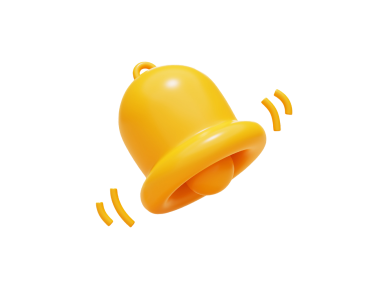
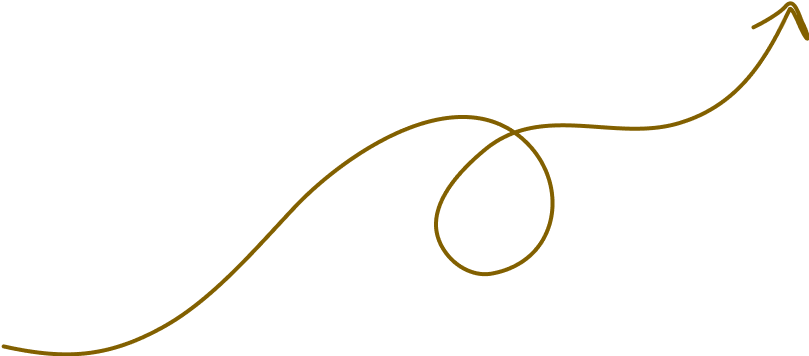