AI Predictive Analytics in Healthcare: 5 Key Use Cases
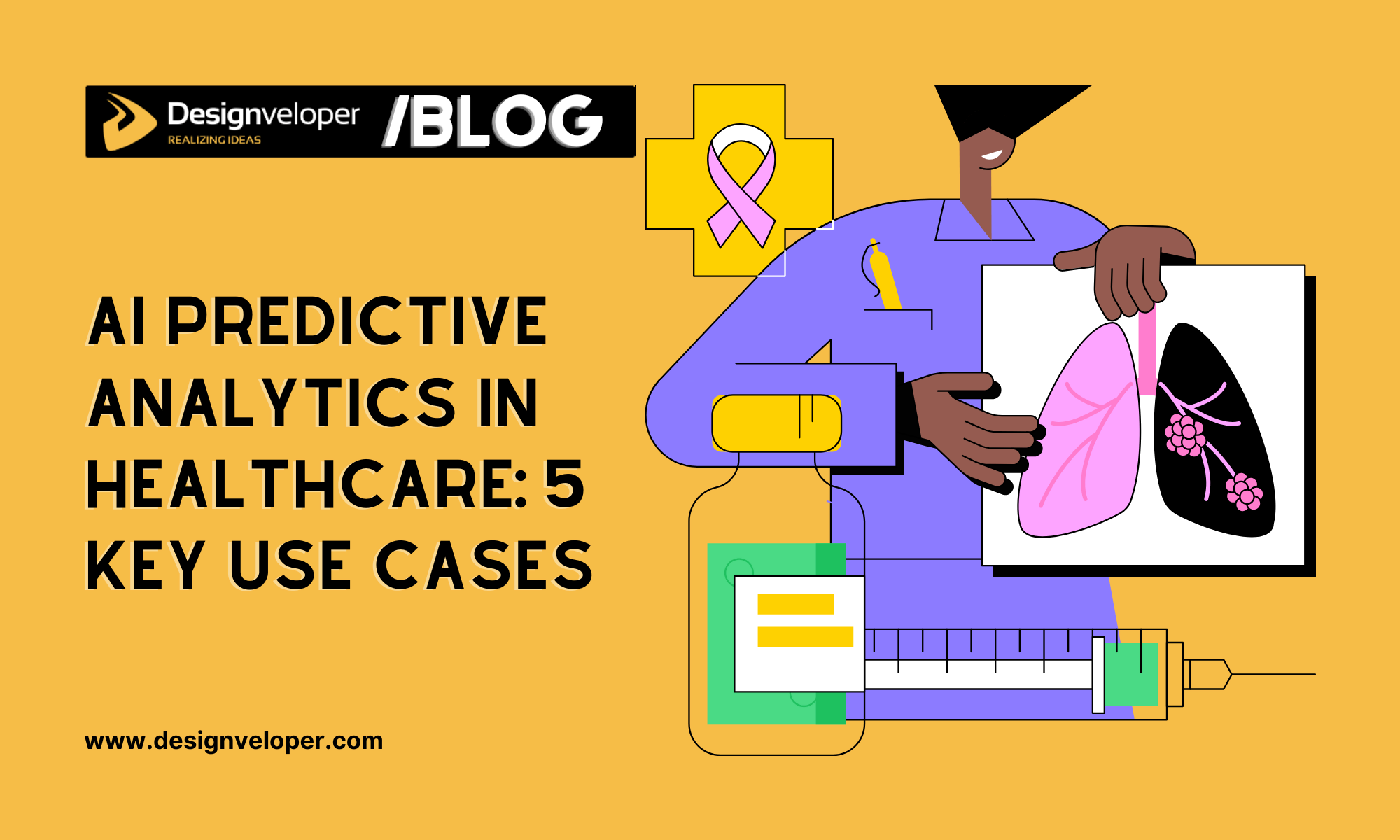
Artificial intelligence, or AI, is transforming different aspects of our lives, especially in healthcare. One of the most promising use cases of AI in this realm is predictive analytics. This application plays a key role in detecting diseases, tailoring patient care, and making better operational decisions. But how?
This article will be the right place if you want to seek everything about how AI is being used for predictive analytics in healthcare. From its five real-world applications to potential challenges, this article will equip you with the necessary knowledge about this interesting topic. So, ready? Let’s dive in!
What is AI Predictive Analytics in Healthcare?
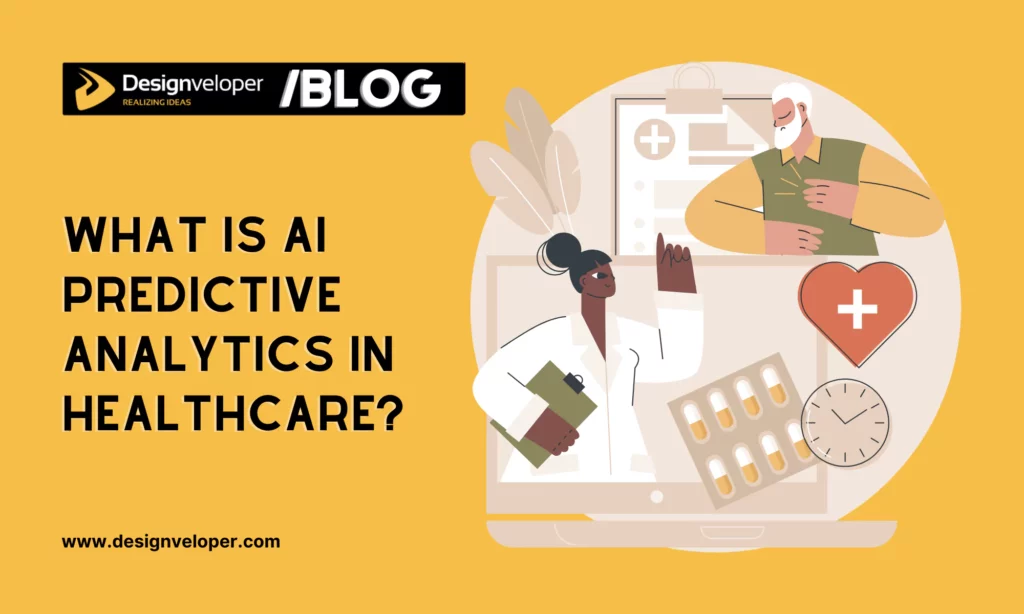
Predictive analytics is a process of analyzing historical data to predict future events. In healthcare, these events may involve disease onset, patient readmissions, pandemic outbreaks, or resource demands.
To generate accurate predictions, predictive analytics must combine statistical models and machine learning algorithms (e.g., K-means clustering or decision trees) to analyze large datasets from different sources as follows:
- Electronic health records (EHRs) that cover a patient’s medical history, diagnoses, medications, and even lab test results.
- Medical imaging like X-rays, CT scans, and MRIs.
- Genetic information.
- Health surveys or interviews.
- Disease or patient registries.
- Social and lifestyle data such as diet, exercise, and environmental exposure.
- Wearable devices such as smartwatches.
- Claims-based datasets (or administrative information) that cover data about patient-provider interactions (e.g., prescription details or appointments).
With AI predictive analytics, doctors can spot early risks of specific health problems (like heart attacks). This enables them to intervene timely and take proactive action to prevent serious conditions. For example, Mount Sinai, a US-based medical center, developed machine learning models to anticipate severe medical conditions and mortality likelihood in COVID-19 patients. These models could support clinical practitioners in managing and caring for these patients better.
Further, the medical industry is witnessing a big shift toward personalized care while facing growing challenges like rising costs. By automating repetitive tasks and aiding informed decision-making, AI predictive analytics can help clinics enhance the quality of care and operational efficiency.
These factors have fueled the growth of healthcare predictive analytics, from $16.75 billion in 2024 to $184.58 billion in 2032.
When Should You Use Predictive Analytics?
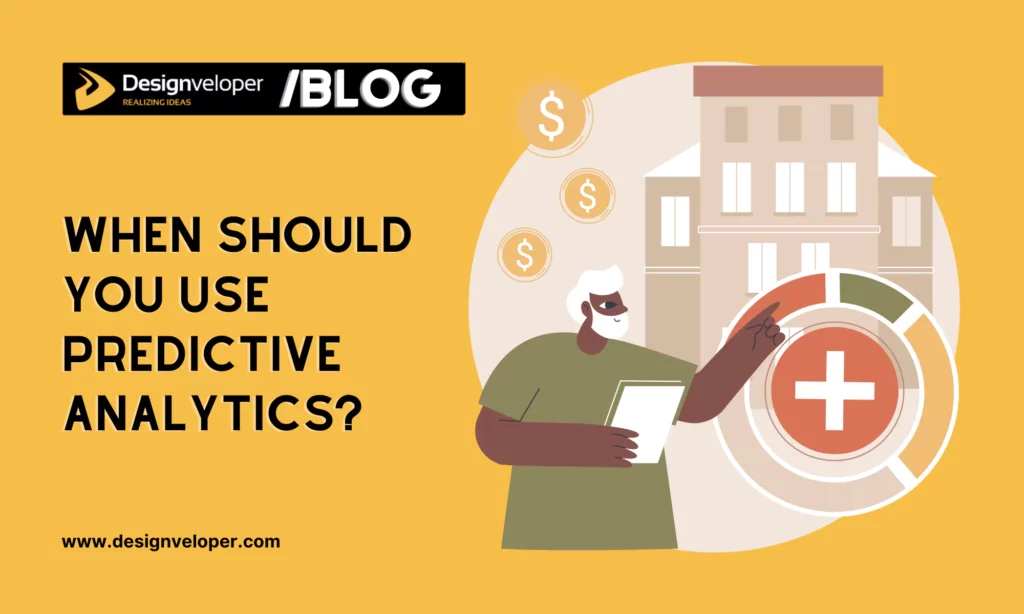
So far, healthcare providers have used traditional analytics like linear regression or statistical modeling to explain past events. By using simple tools like spreadsheets, these techniques can quickly answer questions such as “What happened?” or “Why did it happen?” to help healthcare providers understand patterns and past trends.
As they focus on providing descriptive insights, they’re sufficient to handle simple, well-defined problems. For instance, traditional analytics can point out that hospital admissions rose by 15% last month. With this capability, traditional analytics is ideal when problems are simple and well-defined.
Predictive analytics, on the other hand, proves more useful when you want to handle complex tasks that require predicting future trends. It analyzes historical data using advanced algorithms to answer questions like “What is likely to occur?” or “What should we do to prevent it?”.
This analytics approach can harness a vast amount of data (even including unstructured data such as clinical notes) to identify hidden patterns. For example, predictive analytics can forecast that hospital admissions will increase by 20% next month and even suggest appropriate steps to react to the demand.
5 Real-World Use Cases of AI Predictive Analytics in Healthcare
Now, AI-driven predictive analytics is increasingly popular in healthcare due to its transformative benefits. Let’s see how this analytics technique is applied in the medical industry through the following real-world applications:
1. Early Disease Detection and Diagnosis
One of the most common applications of AI predictive analytics in healthcare is detecting and diagnosing diseases at an early stage. This analytics technique uses machine learning and deep learning algorithms to analyze a wide range of data, especially medical imaging (e.g., X-rays, CT scans, or MRIs). It then helps doctors spot subtle abnormalities and identify individuals with a high risk of developing specific diseases.
Looking at the figure below, you may better understand how an AI model is trained and operated for early disease detection and diagnostics:
As can be seen, the trained AI model can analyze a chest X-ray and generate a prediction (e.g., “Cardiomegaly” with a confidence level of 78%). However, without the explainability feature, the trained AI model may not clarify how it made the prediction and which parts of the X-ray contributed to the prediction. This means that doctors hardly measure whether the prediction is correct or incorrect.
That’s why some trained AI models today integrate deep learning algorithms to offer more interpretable insights into their predictions. Accordingly, the trained AI model in the above figure can explain the reasoning behind its outcome (“The lungs exhibit cardiomegaly with a large silhouette of the heart.”).
To do so, it compares the current image with similar ones in its training dataset to see whether the heart silhouette size increased. Further, it uses heatmaps to visualize which regions of the image impacted the prediction. This helps doctors make more accurate predictions.
2. Treatment Personalization
AI-driven predictive analytics plays a big role in tailoring treatment plans and improving precision medicine initiatives.
How? AI systems can constantly track a patient’s health status and disease progression over time by analyzing data from wearable devices, medical records, or lifestyle factors. For example, wearable devices can record heart rates, glucose levels, and activity patterns in real-time. This allows doctors to customize patient care instantly based on the patient’s condition.
Further, AI advancements and the growing popularity of rare diseases have increased the use of predictive analytics in personalized medications. Particularly, AI can analyze a patient’s genetic information and predict how the patient metabolizes specific medicine. This helps doctors identify the right dosage and medications that fit the patient, allowing personalized care plans.
With this capability, AI predictive analytics has a wide application in cancer treatment. In 2024, researchers from the National Cancer Institute (NCI) developed an AI tool called PERCEPTION to predict drug responses. Particularly, they used high-resolution gene expression data from individual tumor cells to understand why some cancer patients develop resistance or don’t respond to drugs, matching them with medications that will be effective for their certain tumor DNA. This not only optimizes therapeutic outcomes but also reduces negative effects.
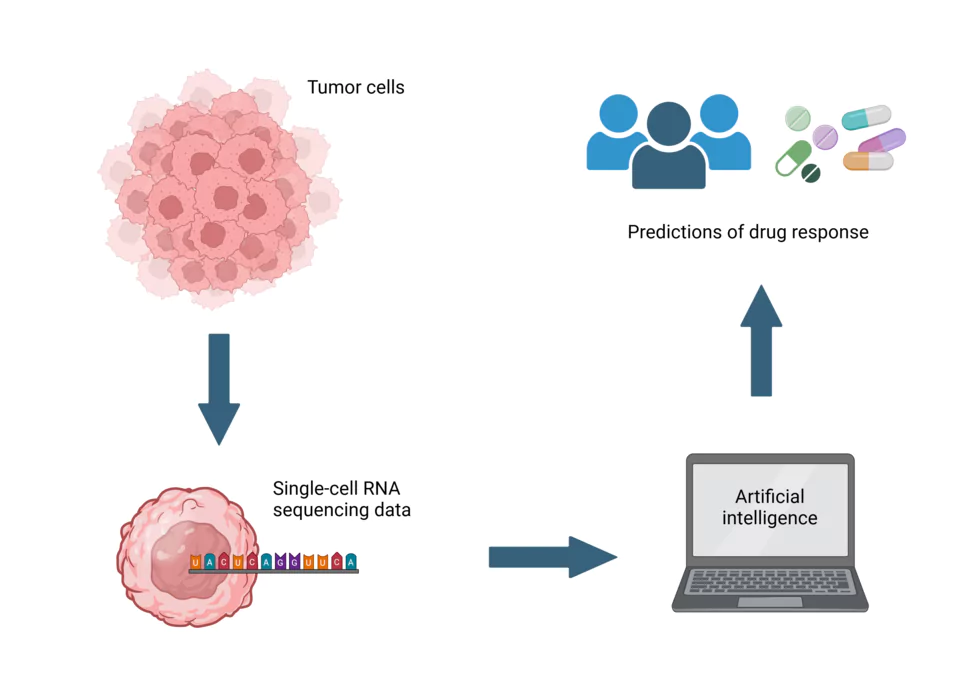
3. New Drug Discovery
Normally, drug development takes 10-15 years for completion, from conceptualization to delivery, let alone its extremely high risks like drug attrition. This is where AI predictive analytics comes into play. This advanced technology is introduced to speed up new drug discovery in healthcare, with a roughly 30% annual growth rate from 2024 to 2029. Here’s how:
First, AI models can screen candidates for drug testing. These candidates are molecules that can be developed into therapeutic drugs for certain diseases. By analyzing their molecular structure and mimicking protein-protein interactions, AI can forecast the effectiveness and safety of these candidates. This will reduce the list of candidates for further testing.
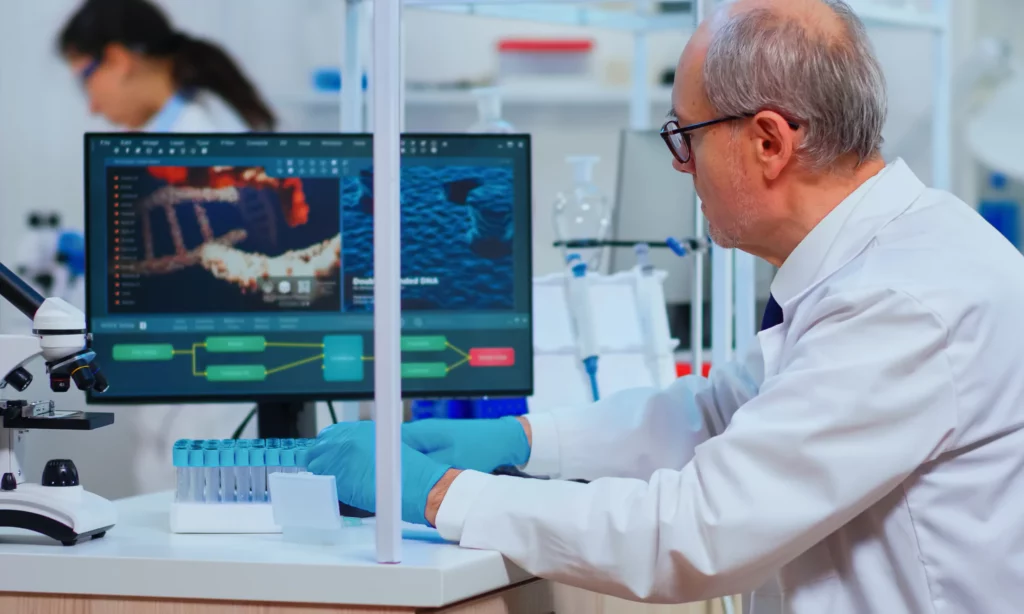
Second, AI’s predictive algorithms aid researchers in developing new molecules with desired properties. They also help modify existing compounds to enhance their performance or minimize side effects.
For example, Insilico Medicine – a biotechnology company – uses generative AI to design ISM001-055, a new drug candidate that treats idiopathic pulmonary fibrosis (IPF). Despite just being in the Phase IIa trial, the drug is considered safe and well-tolerated for patients. It also helps enhance forced vital capacity (FVC) – a crucial measure of lung function – in 12 weeks.
Third, AI plays a key role in drug repurposing. By analyzing patterns in medical and clinical information, AI systems can discover new uses for existing medications. For this reason, a drug designed for one disease can be repurposed to cure another based on predictive insights.
One typical example of this application is the repurposing of Baricitinib, which was originally designed to combat rheumatoid arthritis, to prevent COVID-19. Using AI algorithms to analyze molecular interactions and disease pathways, researchers can determine Baricitinib as a promising treatment.
4. Operational Efficiency
AI predictive analytics improve operational efficiency in healthcare by streamlining workflows and optimizing resource allocation.
Particularly, AI models can predict admission rates by analyzing historical patient data, local events, and seasonal trends. These predictions allow healthcare facilities to plan staffing, allocate resources (e.g., beds or equipment), and monitor supply chains efficiently. As a result, they can avoid underpreparation or overstaffing, minimizing operational costs.
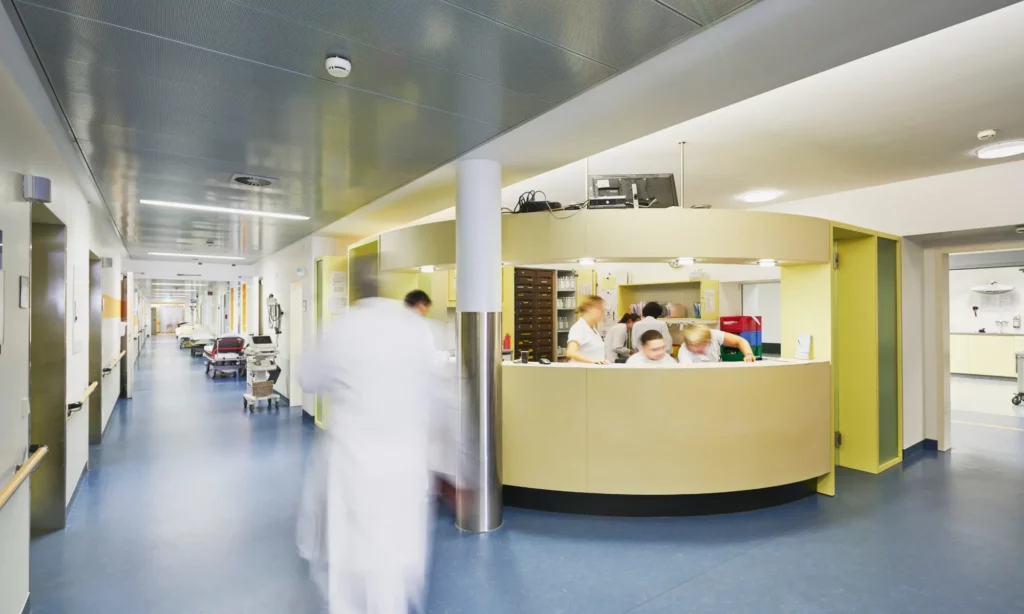
Hospitals and clinical centers also use AI tools to anticipate readmission rates. The Cleveland Clinic, for example, developed a “readmission risk score” instrument to forecast the possibility of a patient being re-admitted to the hospital. This tool analyzes data from electronic medical records (EMRs) and looks at different factors like past hospital visits (especially Emergency Department trips) or type of admission.
The score ranges from 1 to 100. If patients score above 40, they’ll stay in the top 5% of those most likely to be readmitted. This tool proves effective, even during COVID-19, although it works less accurately for conditions like rare diseases or infections.
5. Population Health Management
On New Year’s Eve, BlueDot – a Canadian AI-powered health monitoring tool – identified the early signs of the COVID-19 pandemic in Wuhan, China. These signs were alerted by BlueDot almost a week before the World Health Organization and the US Centres for Disease Control and Prevention issued their first statement about COVID-19.
BlueDot’s AI algorithms analyzed large data sources, including health records, news reports, and even airline ticketing data to spot unusual patterns that signal the likelihood of the outbreak. It also marked a cluster of pneumonia cases and forecasted the potential global spread based on international travel trends.
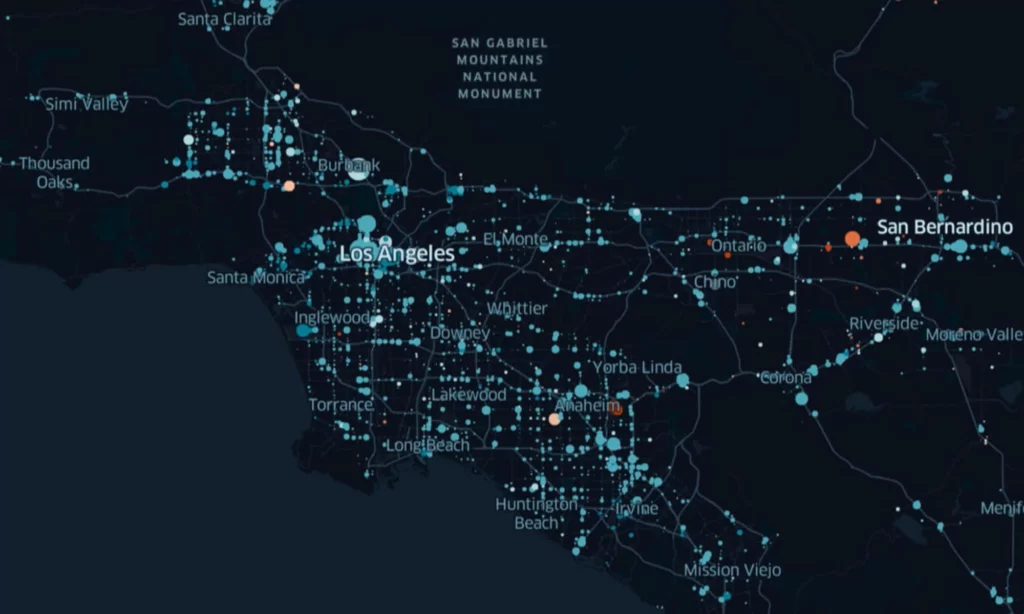
The case study of BlueDot is a typical example of how AI-driven predictive analytics is used to monitor population health. With this capability, AI models help healthcare facilities develop intervention programs that target high-risk populations.
Kaiser Permanente, for instance, built an AI predictive model (known as Advance Alert Monitor) to prevent emergencies before they occur. The tool automatically analyzes the electronic health data of patients every hour to spot those with a high risk of serious conditions. It then alerts a specialized virtual quality nursing team that will review the data to identify how they should intervene. This model helps save roughly 500 lives a year.
Benefits of AI Predictive Analytics in Healthcare
Along with all these applications, AI-powered predictive analytics brings transformative benefits to healthcare providers. They include:
- Enhanced Patient Outcomes: By spotting likely health risks or disease signs early (e.g., sepsis or heart failure), AI allows for timely interventions. Besides, AI can analyze patient data to tailor treatment plans. These capabilities help doctors improve patient outcomes.
- Informed Decision-Making: AI predictive models, especially XAI (Explainable Artificial Intelligence), can offer data-driven insights with clear reasoning. These insights help healthcare professionals make precise diagnoses and treatment plans. Further, administrators can use these models to discover trends, assess potential risks, and make informed decisions.
- Streamlined Operations & Cost Reduction: Predictive analytics can automate parts of hospital workflows. They may involve predicting admissions and readmissions, managing resources (e.g., medical equipment or staff), and supporting drug testing. This helps healthcare facilities save significant costs on unnecessary tests and resource management while ensuring effective operations.
Challenges of Using AI Predictive Analytics
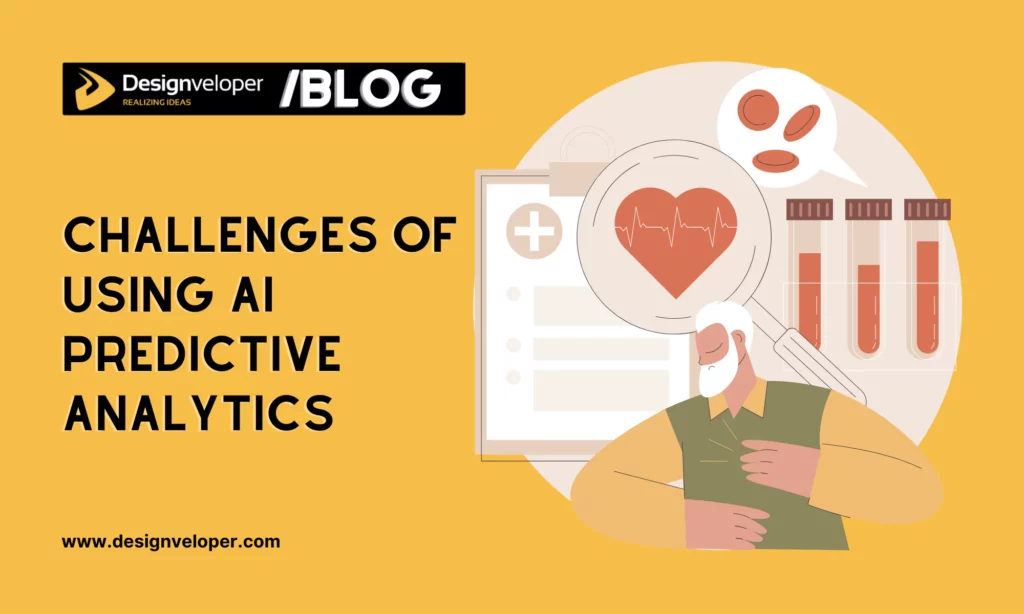
While AI predictive analytics proves helpful in different aspects of healthcare, it still comes with several problems.
Data Issues
This is the biggest challenge that hinders the wide adoption of AI predictive analytics in the medical industry. These issues are about data privacy, security, bias, and scarcity.
As we all know, AI models often require a vast volume of patient and clinical data to generate predictions. However, such data is considered highly sensitive, hence often coming with risks like possible data breaches or misuse. Therefore, it’s no wonder why many patients are hesitant to share their health records with healthcare providers. Without enough or correct data, AI systems may have difficulty providing accurate outcomes.
Moreover, datasets may contain biases, which might result in inaccurate or unfair predictions. For example, if training data underrepresents specific demographics, an AI model can perform poorly on those groups.
Another data issue is scarcity. Some rare diseases or health conditions can have limited data, or the available dataset is of poor quality. This will influence the accuracy of predictions significantly.
To minimize these adverse impacts, healthcare providers must leverage security practices and ensure adherence to regulations like HIPAA to protect patient data. Additionally, AI models should be trained on diverse datasets and their outcomes need to go through rigorous audits by humans to reduce bias.
Integration with Existing Systems
Many healthcare providers are using legacy systems that might not be compatible with AI predictive models. Upgrading infrastructure to integrate these models seamlessly can be time-consuming and expensive. Therefore, it’s crucial to develop modular and interoperable AI solutions to ensure smooth integration with existing systems.
High Investment Cost
We can’t deny that AI predictive analytics would bring long-term benefits in terms of operations, healthcare, and finance.
However, initial investments and even ongoing maintenance costs are so high that many healthcare facilities, especially small and medium-sized ones, can’t afford them. Not to mention that these modern healthcare solutions require skilled staff to develop, install, and update.
Therefore, healthcare providers need to consider their budget and long-term goals before deciding to invest in AI predictive models.
How to Get Started with AI Predictive Analytics in Healthcare: Designveloper’s Expert Advice
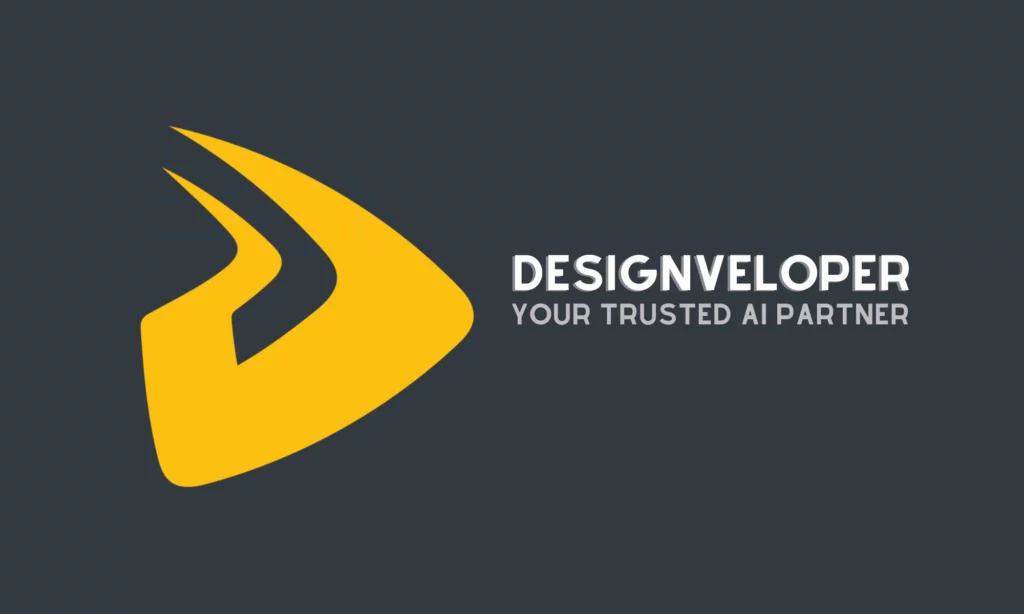
If you want to invest in AI predictive analytics in your healthcare facility but don’t know where to start, let’s take our expert advice.
1. Identify the Right Use Cases for Implementation
AI predictive analytics has a wide range of applications in healthcare, as we listed above. So, which areas of healthcare do you want AI to help with? Are you looking for AI solutions for disease management, personalized patient care, and operational efficiency? Understanding your facility’s specific needs is the first step to building the right AI model. For example, if you prioritize reducing hospital readmission rates, focus on AI models that can forecast high-risk patients.
2. Develop a Strong Data Infrastructure and Governance Framework
Data is a key component of AI predictive analytics. Therefore, ensuring smooth access to high-quality, diverse data is a must to help AI models produce precise outcomes. Further, to handle data privacy and security concerns, you should build a governance framework to monitor and use data securely and ethically. This framework will ensure strict compliance with industry standards to safeguard patient information while helping AI models work effectively.
3. Collaborate with AI and Healthcare Experts
If you want AI predictive models to work efficiently, it’s crucial to bring together AI and healthcare professionals. The former can develop and implement AI models that match your technical needs while the latter ensures your AI solution can solve real-world clinical challenges.
4. Train and Upskill Healthcare Staff
AI predictive models are only effective if people know how to use them correctly and ethically. So, it’s essential to train your healthcare staff to understand and work with these systems effectively. In addition, you should upskill clinicians in grasping predictive insights and adopting them to decision-making. This training would build trust in AI tools and allow for better AI usage.
5. Partner with AI Solution Providers
If you don’t have a strong in-house team to craft a customized AI solution, outsourcing this development is a good option. However, choosing the wrong partner can ruin your AI journey. So, look for reliable providers with a proven track record in healthcare.
As one of the leading companies in Vietnam, Designveloper has extensive expertise and experience in AI and software development. We’ve successfully developed ODC, a France-based telehealth platform, to connect doctors and patients, especially during COVID-19. The platform facilitates healthcare services like e-prescription or online appointments while abiding by stringent regulations like GDPR or HIPAA.
With our understanding of healthcare and AI, we’re committed to building tailored AI solutions that can scale with your demands. We also offer full life-cycle support for AI development, from conceptualization to ongoing maintenance.
Conclusion
This article provided you with a basic understanding of AI predictive analytics in healthcare, from its common use cases to challenges. If you want to start this AI journey, you need a thoughtful approach that focuses on clear requirements, reliable data, and strong collaboration. Invest in your healthcare staff and work with trusted technology providers. These steps will help your healthcare facility achieve the best results with AI tools. Wanna share your AI idea? Contact us now and discuss this further!
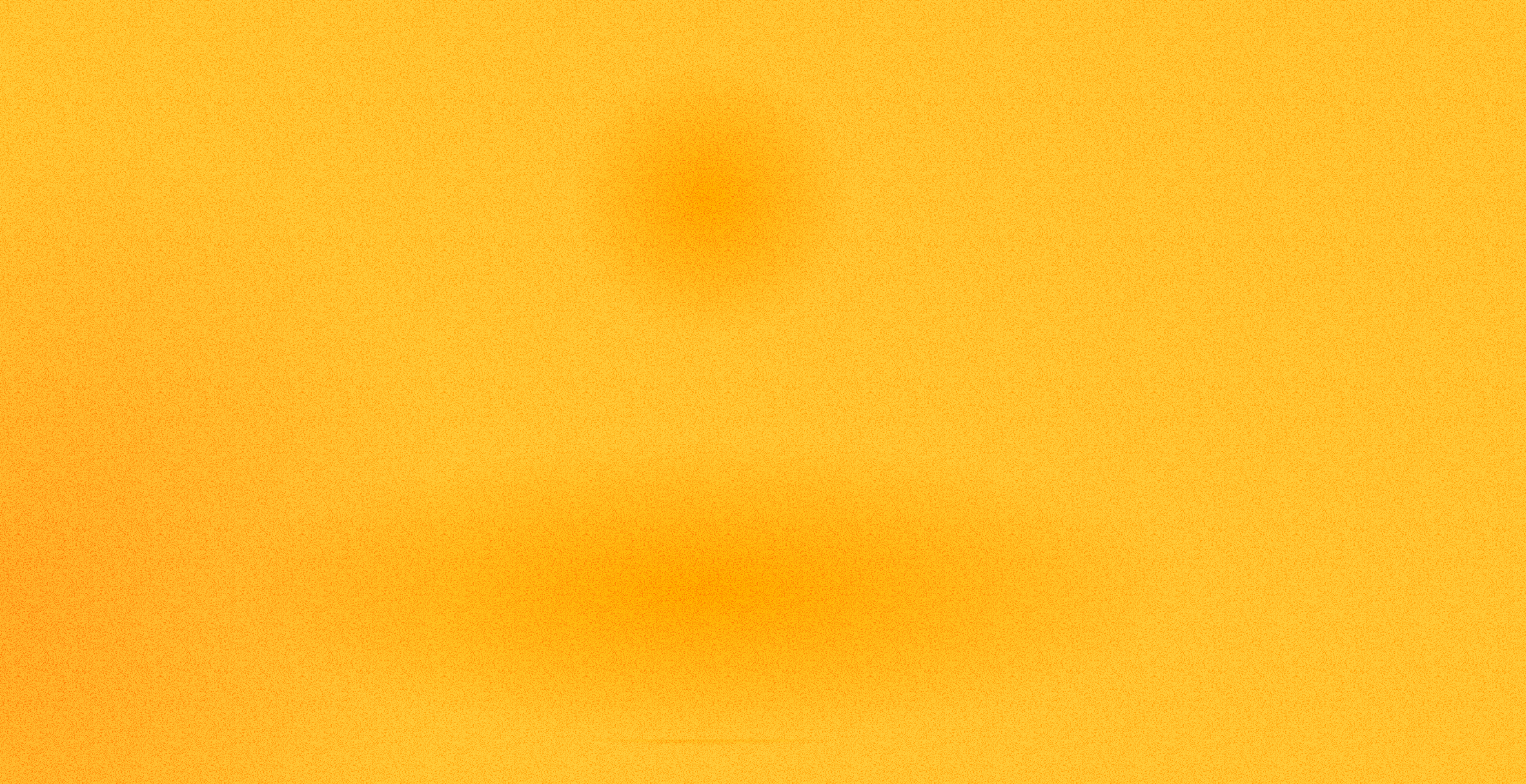
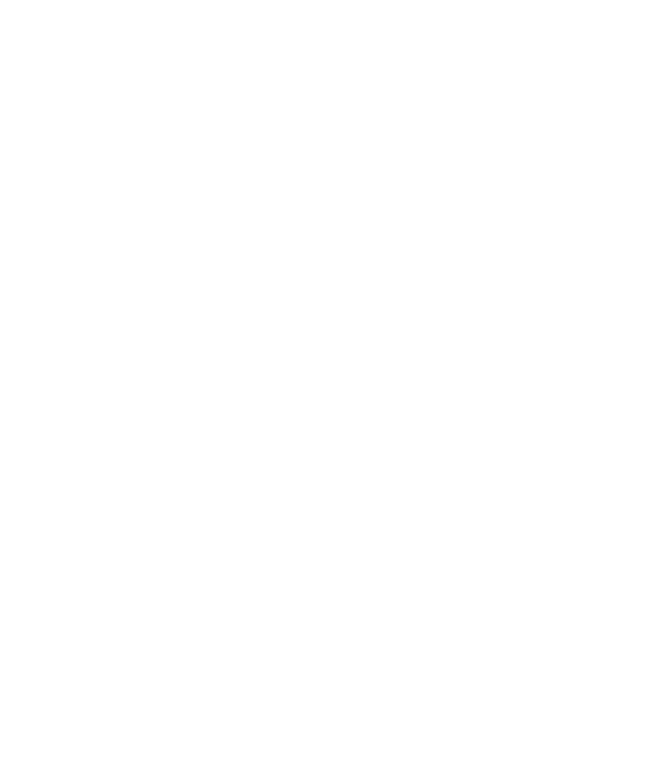
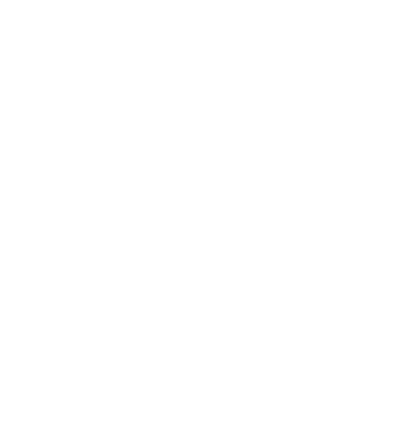
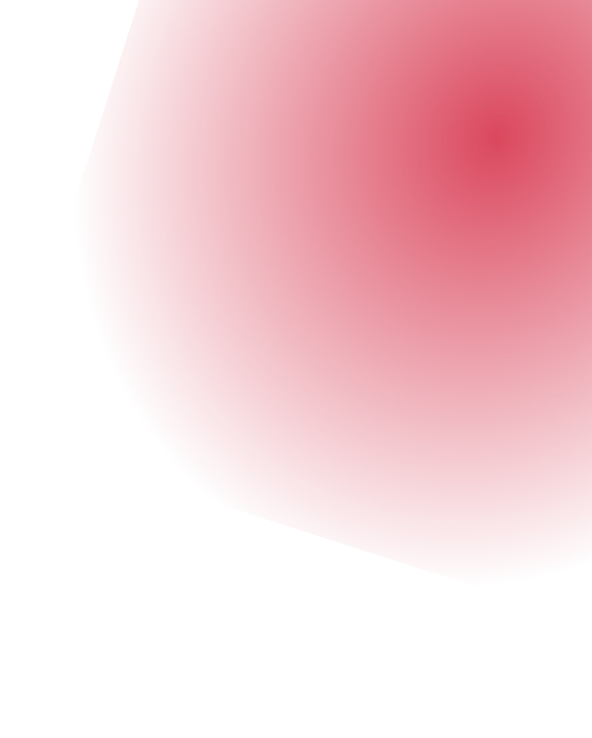
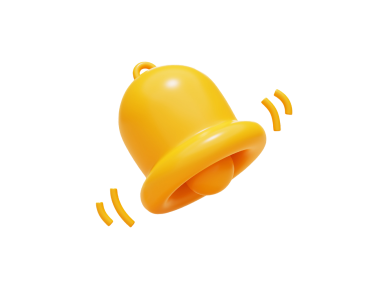
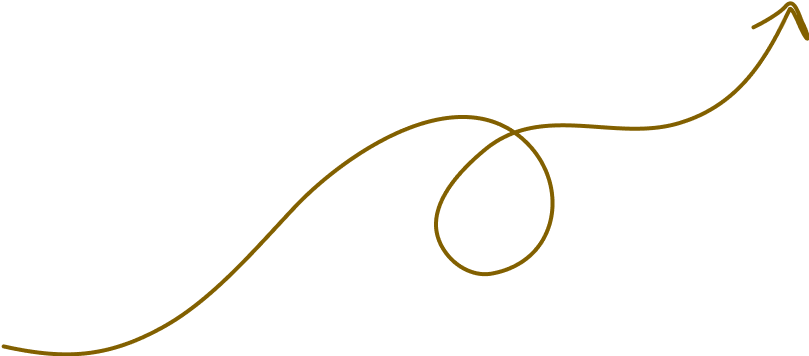