Machine Learning in Supply Chain: Top 5 Real-World Applications
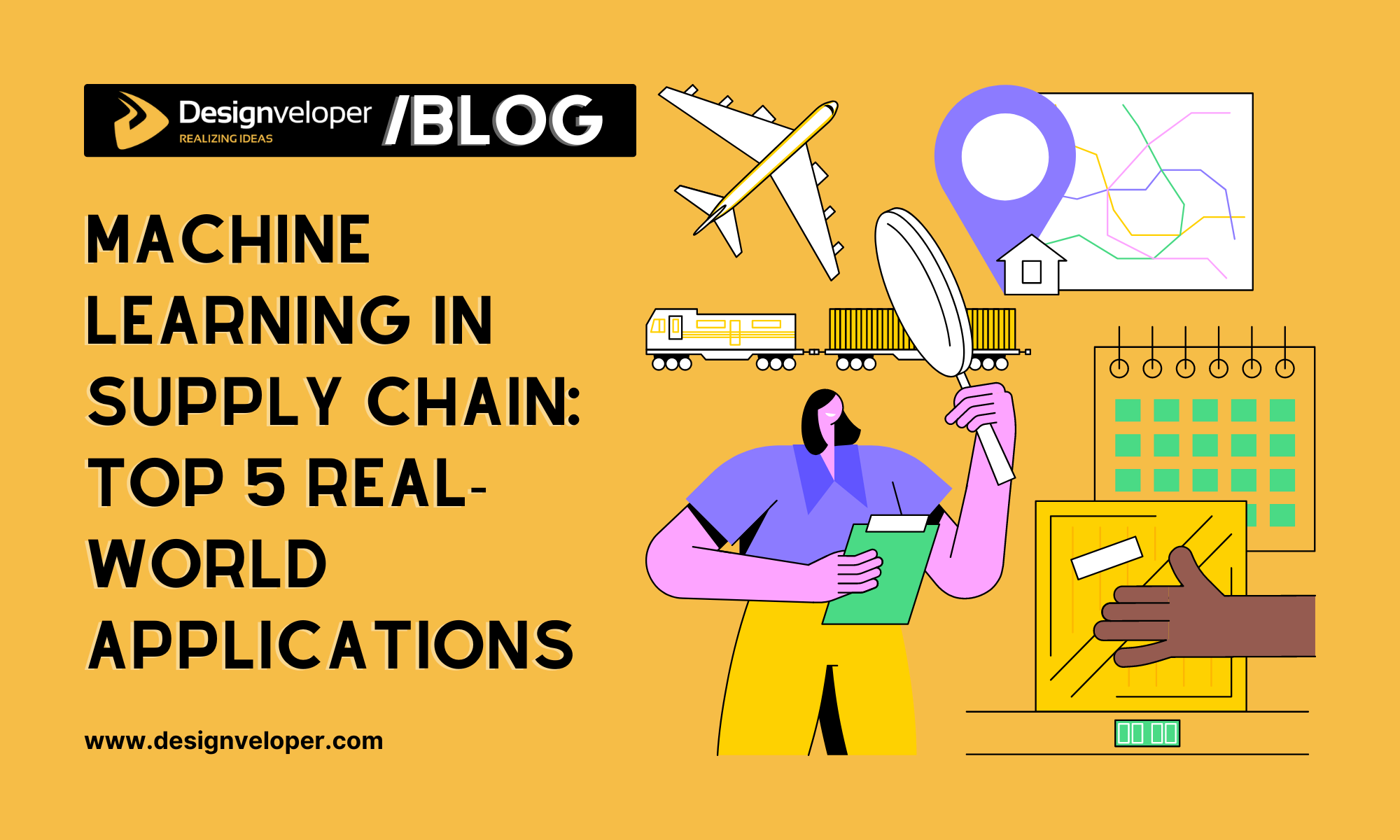
Machine learning in supply chain management is revolutionizing the industry. New stats reveal that 50% of supply chain leaders are investing in AI technologies to improve efficiency and reduce costs. This trend is only going to grow in the coming years.
For instance, machine learning algorithms are better at predicting demand than traditional methods. These predictions are used by companies like Amazon to optimize their inventory, so that products are always available to customers without excess stock. Not only does this reduce costs, but it also increases customer satisfaction.
In general, machine learning is not just a buzzword, but a game changer in supply chain management. This technology can help businesses become more efficient, save costs, and improve customer satisfaction. Curious about diving deeper? Read this article from Designveloper.
The Importance of Machine Learning in Supply Chain
Machine learning in supply chain management is transforming how businesses operate. This benefits by increasing the efficiency and also reducing the cost. A recent McKinsey report found that early adopters of AI enabled supply chain management have reduced logistics costs by 15 percent, inventory levels by 35 percent, and service levels by 65 percent.
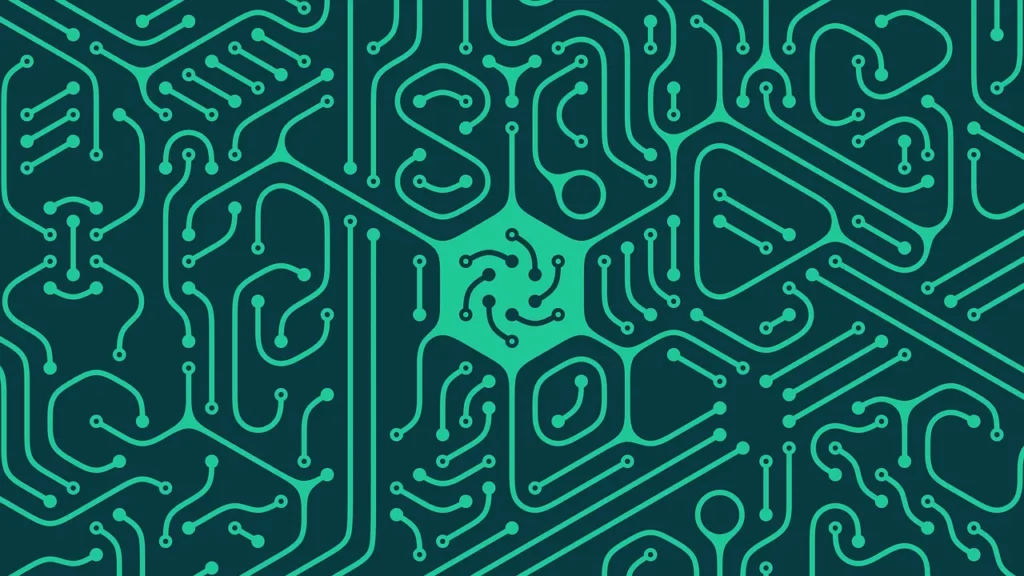
One key advantage is to have better demand forecasting. By using historical data, machine learning algorithms try to predict future demand better. This stops stock outs and overstocking yet smoothens operations. For instance, Gartner found that top performing supply chain organizations are using AI and machine learning to optimize their processes at more than twice the rate of low performing peers.
Inventory management is another important application. By analyzing many of the same factors such as sales trends and seasonal fluctuations, machine learning helps balance inventory levels. It helps ensure that companies have the right amount of stock at the right time, cutting holding costs and improving cash flow.
Supply chain agility is also improved by machine learning. It enables the companies to respond to market change or supply chain move immediately. An example of this is companies using machine learning were able to pick up the changes in demand and supply much faster during the COVID-19 pandemic.
In summary, machine learning in supply chain management is crucial for modern businesses. It helps in demand forecasting, inventory management and generally supply chain agility. Using machine learning, companies can be more efficient, less costly and more resilient to disruptions.
Top 5 Applications of Machine Learning in Supply Chain
What if the supply chain learns and adapts on its own? It sounds like something from the future, but it’s happening now thanks to machine learning in supply chain management.
Application 1: Demand Forecasting
Machine learning in supply chain has revolutionized demand forecasting. Advanced algorithms are used now to make better and better predictions of future demand. It helps in keeping inventories at minimum level and in reducing costs.
Machine learning can increase forecast accuracy by up to 50%, according to recent reports. It is important for businesses to remain competitive. For example, McKinsey’s survey revealed that 90 percent of supply chain executives intend to overhaul their planning IT within five years.
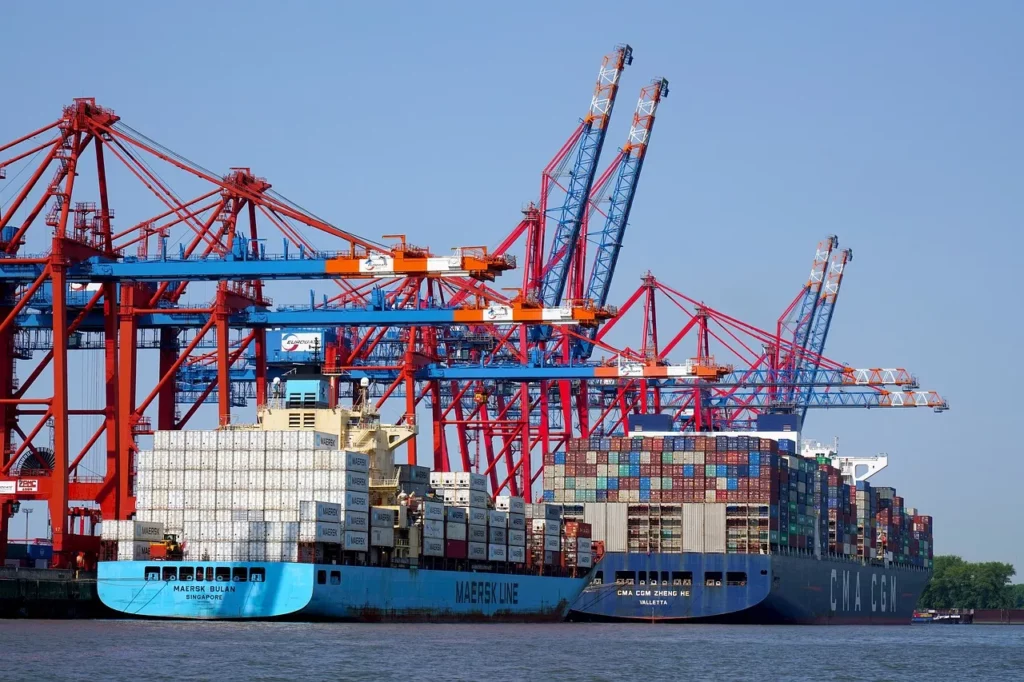
Walmart is one notable example. Machine learning is used by the retail giant to analyze huge amounts of data, including past sales, weather patterns and economic indicators. Now they have the ability to stock the right quantity of the right products at the right time and that keeps waste to the minimum and then the customers are quite satisfied with their products.
In conclusion, machine learning in supply chain is a game-changer for demand forecasting. It gives businesses the ability to make the right decisions and keep up with the competition.
Specific example: Retail sector using ML for inventory management
Retailers continually seek ways to control inventory better. A solution to this is provided by machine learning (ML) which predicts patterns of demand and optimizes stock levels.
ML can increase inventory accuracy by a lot. McKinsey study shows how ML enables retailers to react quickly to market changes and keep inventory levels at the optimal level. With ML, retailers can eliminate stockouts and reduce risk of excess inventory as well, resulting in huge cost savings.
In summary, machine learning in supply chain, especially in the retail sector, transforms inventory management by providing accurate demand forecasts and optimizing stock levels. Not only does this improve customer satisfaction but translates into profitability.
Application 2: Predictive Maintenance
Machine learning in supply chain is transforming predictive maintenance. Algorithms can predict equipment failures in advance, by analyzing data from sensors. This cuts downtime and maintenance costs.
General Electric (GE) monitors its industrial equipment using machine learning, for example. Data on machine performance is collected by sensors and algorithms analyze this data to predict potential issues. This enables GE to do maintenance only when it is needed, and not have unexpected breakdowns.
According to reports, predictive maintenance can cut costs in a lot of ways. It is necessary for businesses trying to work smarter. A study from Boston Consulting Group (BCG) says that companies employing predictive maintenance see a dramatic decrease in unplanned downtime.
In conclusion, machine learning in supply chain helps businesses maintain their equipment more effectively. It helps to keep things running smoothly and save money.
Specific example: Manufacturing industry reducing downtime
Predictive maintenance is indispensable for the manufacturing industry. Companies can predict equipment failures before they happen by using machine learning in supply chain. That cuts downtime down by a massive margin. For example, McKinsey reports that early adopters of AI enabled supply chain management have reduced logistics costs by 15 percent and increased service levels by 65 percent.
For an example, take a car manufacturer where machine learning algorithms are being used to monitor its assembly line machines. In real time, the system analyzes data from sensors and predicts when a machine is likely to fail. So that when things go wrong in production, maintenance teams can get them fixed ahead of time before production stoppages occur. And as a result, the company got a 30% decrease in unplanned downtime.
A machine learning method such as another pharmaceutical company predicting when critical equipment needs to be maintained. This helps to ensure that production line will continue with no interruptions. Not only does this improve efficiency, it helps keep product quality.
These examples highlight how machine learning in supply chain can transform the manufacturing industry by reducing downtime and increasing productivity. To learn more about this topic, you can take a look at the Boston Consulting Group’s report on predictive maintenance in manufacturing here.
Application 3: Inventory Optimization
Machine learning in supply chain is transforming inventory optimization. It means algorithms can look at the data and figure out when it is best to keep stock levels. It helps businesses away with the overstocking and stockouts.
For instance, Amazon manages its massive inventory with machine learning. To make sure you have the right amount of stock at all times the algorithms look at sales data, seasonality, and customer behaviour.
Machine learning can cut inventory costs significantly, according to reports. This is important for businesses that want to become more efficient. A McKinsey study showed how companies that use machine learning for inventory optimization achieve substantial savings in logistics costs and service levels.
In conclusion, machine learning in supply chain helps businesses maintain optimal inventory levels. It helps to keep things running smoothly and save money.
Specific example: E-commerce platforms managing stock to meet customer demand
Machine learning is used in E-commerce platforms to optimize stock management. This helps them to meet customer demand effectively. To give an example, Amazon uses machine learning algorithms to predict demand for products. This allows them to always have their inventory levels locked in just right, thereby avoiding stockouts.
McKinsey report reveals that early adopters of AI enabled supply chain management have seen great results. They report 15% reductions in logistics costs, 35% decreases in inventory levels, and 65% increases in service levels. This illustrates the fundamental role played by machine learning in optimizing inventory.
Another example is Alibaba. Machine learning is used by the company to analyze customer behavior and predict future demand. That way, they can determine how much of every product to carry, as they would always have the right products.
In short, machine learning in supply chain is crucial for e-commerce platforms. In helping them manage stock efficiently, meet customer demand and generally improving operational efficiency.
Application 4: Route Optimization
Machine learning in supply chain is transforming route optimization. Nowadays advanced algorithms analyze huge data to find most efficient routes for the delivery. It does this significantly cutting fuel costs and delivery times.
McKinsey report shows that early adopters of AI enabled supply chain management have reduced logistics costs by 15%. Such optimization for supply chain operations is powerful testimony towards the prowess of Machine Learning.
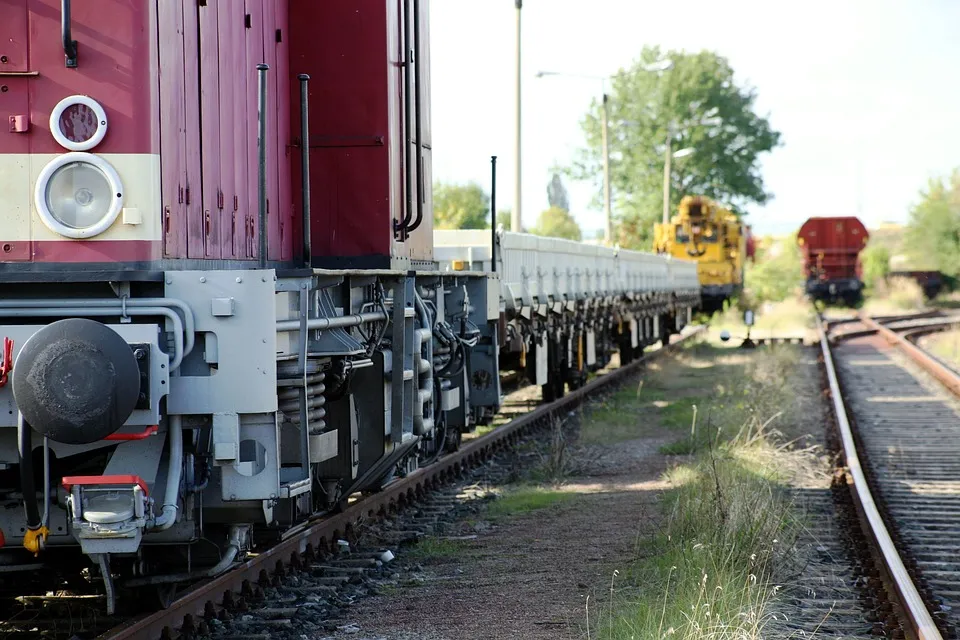
Machine learning is used by UPS to optimize delivery routes, for example. It analyzes traffic patterns, weather conditions and delivery windows to pick the best routes. It not only makes things more efficient but it pleases customers as well.
In conclusion, machine learning in supply chain is a game-changer for route optimization, offering substantial cost savings and efficiency improvements. The advantage with this technology is that companies that embrace it are in a better position to do well in a competitive market.
Specific example: Logistics companies like DHL or FedEx
Machine learning is used by logistics companies such as DHL and FedEx to optimize delivery routes. It helps cut costs and shorten delivery times. For example, DHL’s ‘Greenplan’ algorithm takes its data to find the best transport routes. It also ensures deliveries are efficient to a wide area.
Machine learning is also used by FedEx for route optimization. AI models of theirs analyze the traffic patterns, weather and delivery schedules. It helps drivers to avoid delays and timely deliveries.
Statista report shows that AI in logistics is going to grow significantly. The global cargo drone market, including AI driven logistics, is expected to reach $17.88 billion by 2030. The point here is the increasing role of machine learning in the logistics industry.
In conclusion, machine learning in supply chain is crucial for logistics companies. This allows them to optimize routes, and save costs in delivery efficiency.
Application 5: Supplier Risk Management
Machine learning in supply chain is transforming supplier risk management. Predictive analytics is now used by companies to predict potential disruptions. It is a proactive approach that helps mitigate risks and makes operations smoother.
Machine learning models can predict supplier reliability with high accuracy, as recent reports show. For example, McKinsey found that early adopters of AI enabled supply chain management reduced logistics costs by 15%. This shows how machine learning has a big impact on cost savings and efficiency.
Resilinc’s AI models for supply chain risk management are one notable example. These models look at huge amounts of data to find potential risks and suggest ways to mitigate them. Machine learning can help companies make better decisions about their vendor management and supply chain strategies.
In conclusion, machine learning in supply chain is a game-changer for supplier risk management. It helps companies to predict and mitigate risks effectively, resulting in more resilient and efficient supply chains.
Specific example: Automotive industry ensuring continuous supply of parts
A stable supply of parts is of the essence for the automotive industry. Machine learning in supply chain helps ensure this continuity. Companies cycle through demand, inventory levels, and transportation costs and can predict and address potential disruptions by analyzing data.
For instance, Omdena created AI powered solutions for the automotive industry. These solutions analyze historical data to find ways to improve. It aids companies in keeping a steady stream of parts and avoiding production setbacks.
A PTC report shows the benefits of machine learning in the automotive sector. This demonstrates that these technologies can improve customer satisfaction, employee productivity and safety. This shows how machine learning has changed the industry’s supply chain.
It can be seen that machine learning in supply chain is crucial for the automotive industry. This ensures that the parts flow continuously, which, in turn, means more efficient and socially resilient operations.
Conclusion
In conclusion, machine learning in supply chain offers transformative benefits. The value of this is underscored by its real world applications, supported by robust data and examples. Companies that embrace these technologies are better positioned for improved performance and competitive advantage.
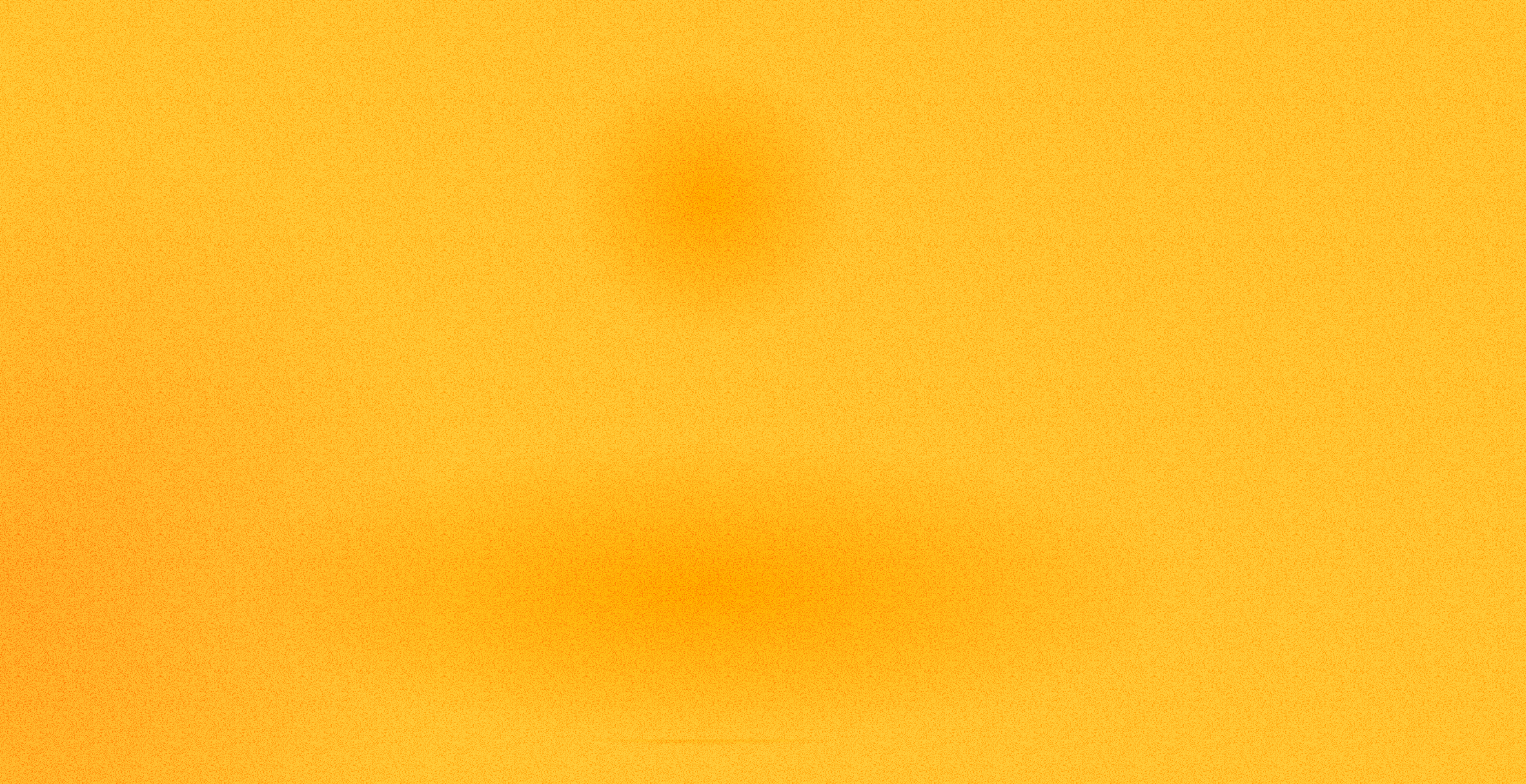
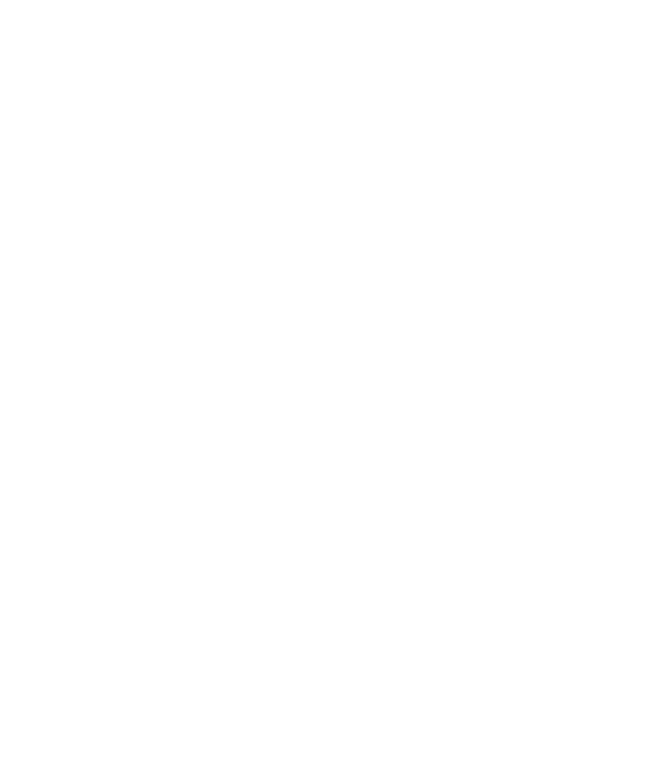
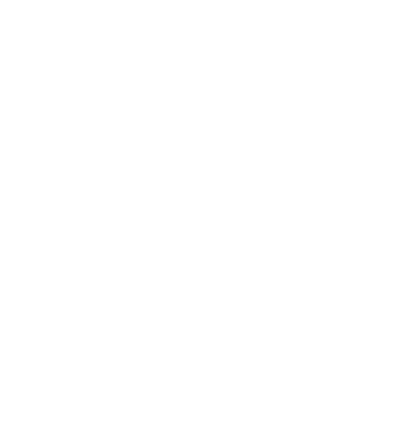
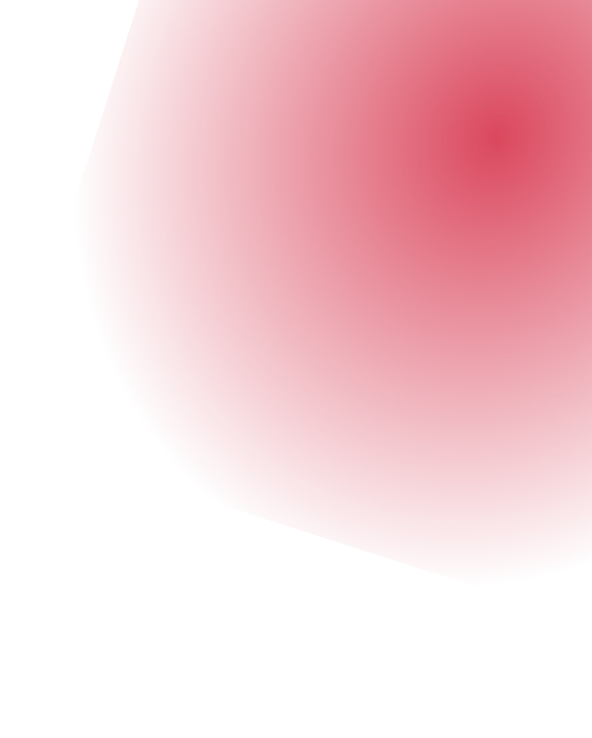
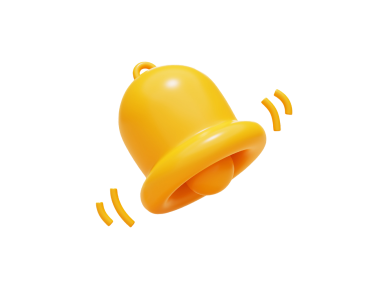
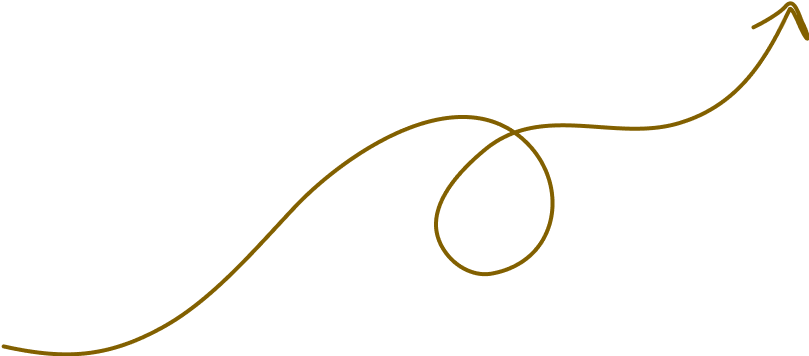