Machine Learning in Risk Management: 5 Use Cases
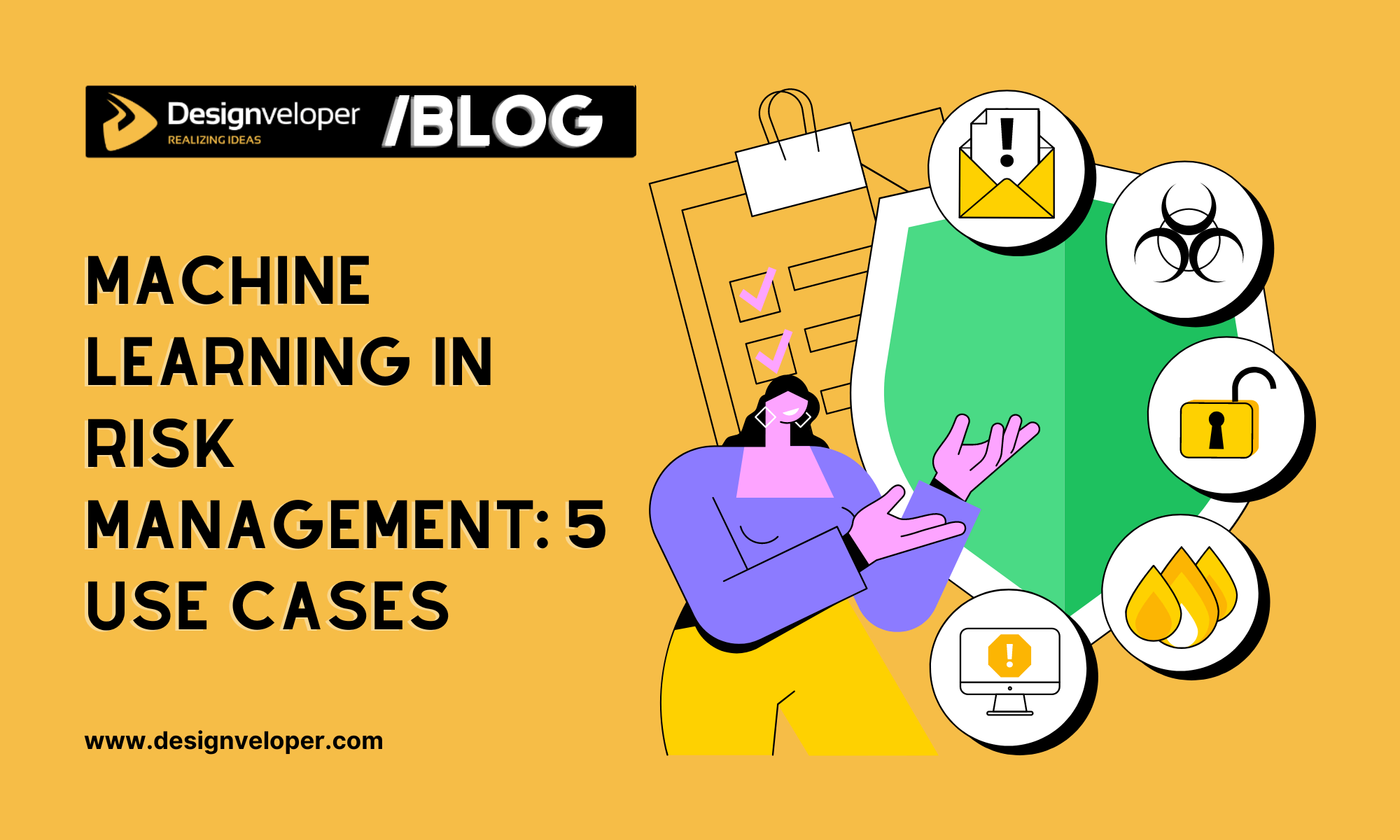
Is your business confronting many risks, from finance and cybersecurity to compliance? You’re not alone. Many businesses now have the same bottleneck that significantly impacts their operational activities. Traditional approaches, however, prove ineffective in risk management, especially when risks are becoming more complex and sophisticated. This is where machine learning appears to change the way we handle risks.
Machine learning in risk management means using advanced algorithms to analyze vast volumes of data and detect risk patterns. This helps your business identify and prevent various threats like fraud or financial losses. If you want to learn more about how it works, keep reading our article! Here, we’ll elaborate on ML’s use cases, benefits, and challenges in risk assessment. But first, let’s dive into which common risks your business may encounter.
What are Common Risks Your Business May Encounter?
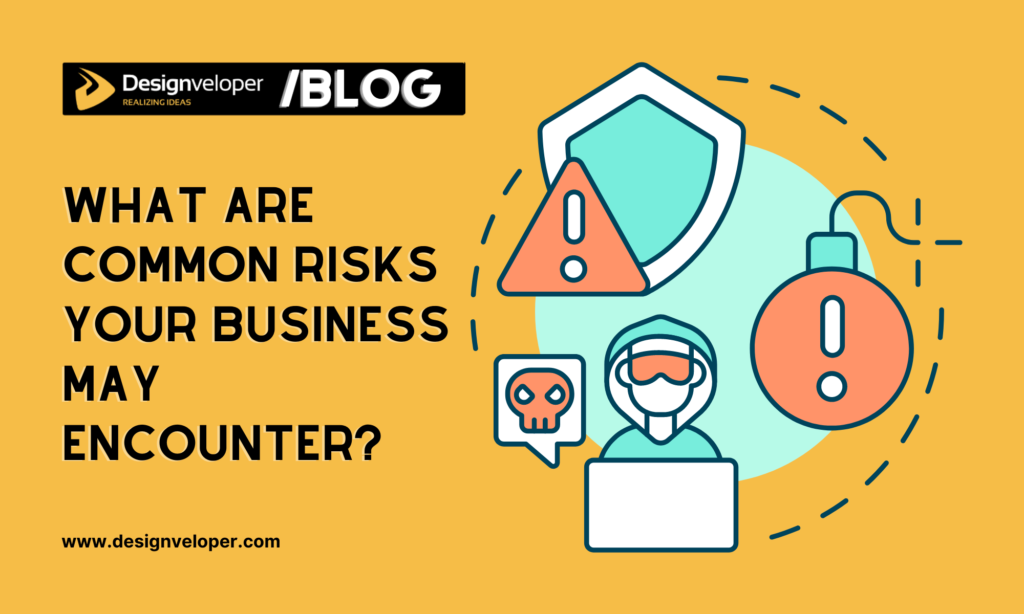
When it comes to business risks, we often think of financial damage or cybersecurity threats. But in fact, a business may encounter more risks than what we mentioned. They include operational risks, compliance risks, market competition, supply chain disruptions, and more. Here, we’ll focus on common risks your business can come across:
1. Financial Risks
Finance is the backbone of businesses as it provides the necessary resources for them to operate, grow, and stay competitive. This means your business may face potential losses when financial risks arise. These risks often involve cash flow problems, high debt, or unexpected expenses.
There are various culprits behind financial risks, such as poor financial management or external factors. Global leaders in the financial industry forecast that businesses would have to face more serious risks in 2024, mostly due to interest rates and inflations. Further, market fluctuations and liquidity issues can greatly impact cash flow and profitability.
2. Operational Risks
Have you ever undergone these scenarios: delays in the manufacturing supply chain or errors in data entry slow down your company’s operations? These are two common types of operational risks. They refer to the potential for losses because of internal failures or external fraud. Accordingly, operational risks come into different categories:
- Process risk: This involves the inefficiency of internal procedures, like supply chain breakdowns, errors in processing transactions, or ineffective processes for addressing customer issues.
- People risk: This covers human-related risks, like a shortage in human resources, unauthorized transactions by employees, or a supplier breach of contract.
- Strategic risk: This refers to any business risk incurred by strategic decisions, like rebranding, new product launches, or mergers.
- Technology risk: This includes all risks of using technology and systems, like system failures, insufficient IT infrastructure, or cyberattacks.
Even the financial issues we mentioned above are also a type of operational risk. Further, external events like natural disasters or political instability also expose out-of-control risks to your company’s operations.
3. Cybersecurity Risks
In 2024, cyberattacks are the top risk for businesses of all sizes worldwide. Several sectors like manufacturing and finance are reported to be more vulnerable to these incidents than others.
Cyber threats occur when hackers attempt to breach your systems, possibly stealing confidential data and disrupting operations. Accordingly, network intrusion, ransomware, and phishing are recorded as the most common attacks targeting US-based companies. They resulted in not only a significant financial loss of more than $452 billion in 2024 but also reputational damage.
4. Compliance Risks
This risk happens when businesses fail to comply with specific industry standards. These standards can include primary legislation or rules issued by legislators, codes of practice by industry associations, market conventions, and internal codes of conduct. Violating these standards can lead to financial penalties and legal issues.
Reasons behind compliance risks vary. For example, employees may not receive enough training to understand compliance requirements. Besides, frequent updates or complexity levels can make it challenging to ensure adherence.
5. Fraud & Theft
This type of risk can take place internally (employee fraud) and externally (customer fraud). Particularly, employees can participate in fraudulent practices like embezzlement or intellectual property violations, while external fraud can stem from customers engaging in payment fraud.
One case study of this risk is the 2016 scandal of Wells Fargo. At that time, the financial institution was embroiled in a massive fraud scandal when its employees were discovered to create millions of unauthorized credit card and bank accounts using customers’ names without their knowledge. The fraud resulted in significant financial losses, and worse, declined the bank’s public perception score to -23.1.
Overview of Risk Management
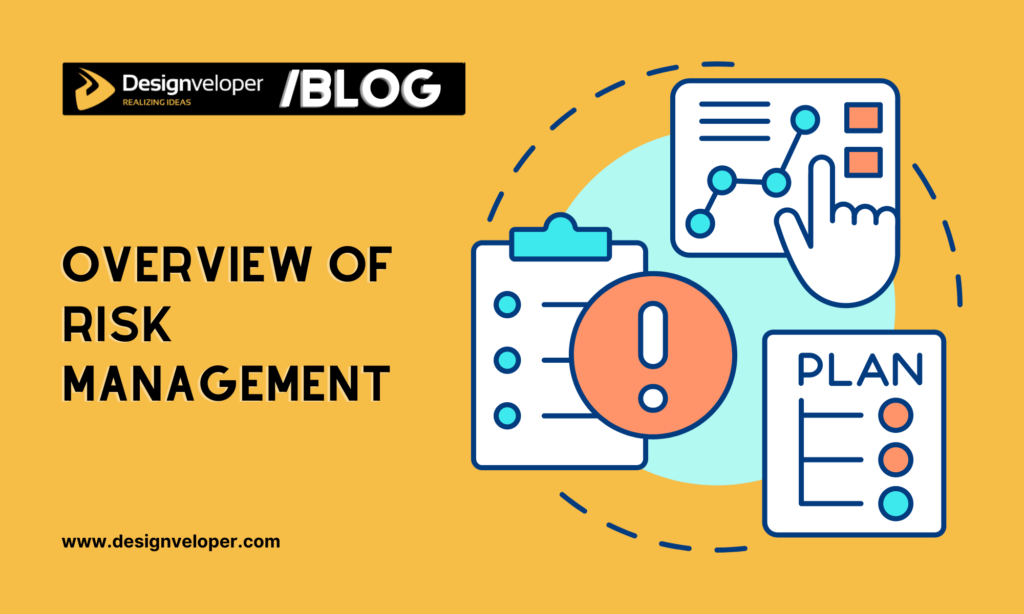
To assess and manage these risks, businesses have adopted such approaches as statistical models and expert judgment.
Statistical techniques are often used to analyze historical data and predict possible risks. In particular, this traditional method focuses on probability distributions, correlations, and past trends to forecast how risk incidents are likely to happen. For instance, many banks leverage VaR (value-at-risk) models to evaluate possible financial losses over a specific time. These models, albeit useful, may not forecast rare or complex risks (e.g., fraud) effectively.
Many companies also rely on expert knowledge and experience to assess possible risks. Accordingly, experts analyze market trends, operational problems, and even the business context to generate insights that are hardly quantified by data. However, expert judgment can be subjective, thus containing human biases.
As business risks become increasingly sophisticated, these traditional approaches prove limited. They accordingly struggle with handling new, complex risk events in real-time. This is where machine learning comes into play, upgrading risk management to a new level.
By using advanced algorithms, ML can analyze huge datasets in a second, detect anomalies, and forecast future risks with a high level of accuracy. ML can even constantly learn and adapt to new databases to offer real-time predictions.
With its huge potential, machine learning can bring lots of immense benefits to your company:
Larger Data Segmentation
Traditional approaches primarily work with structured data (e.g., numerical values or categorized data) as it’s easier to analyze, quantify, and integrate into existing risk management frameworks.
However, they struggle with processing unstructured data (e.g., text, images, and other non-standardized formats) as it lacks a clear format. For this reason, traditional approaches may overlook meaningful insights that are extracted from this unstructured data, consequently leading to inaccurate predictions.
Machine learning, however, is capable of handling all available data, whether structured or unstructured. This allows ML to identify hidden patterns and trends that facilitate risk assessments.
Enhanced Risk Management
Unlike static models, machine learning algorithms can constantly learn from new data and adapt to new behaviors to improve risk predictions over time. This provides your business with impactful insights based on real-time data, hence managing risks more effectively.
Personalized Risk Profiles
A risk profile is a detailed description of a business’s risk exposure. It helps evaluate the possibility of particular risks happening and their possible impact.
Machine learning can customize your company’s risk profile by analyzing its specific data points (e.g., financial transactions or internal processes) to identify a risk score (e.g., financial stability). This personalization allows your company to make more accurate decisions and improve risk management.
Streamlined Workflows
Machine learning can automate repetitive tasks, hence minimizing human errors and burdens in risk management processes.
By analyzing vast datasets and detecting patterns that humans can miss, ML can spot unusual network activity, identify ineffective business operations, and predict possible risks (e.g., vendor disruptions or customer defaults). It can even automatically discover potential regulatory violations and provide early warnings.
For this reason, your business can channel more time and effort into strategic initiatives while keeping risk assessment processes effective.
Top 5 Use Cases of Machine Learning for Risk Management
With these potential benefits, machine learning has been increasingly adopted for risk management. Here are its five popular use cases that businesses globally are focusing on:
1. Credit Risk Modeling
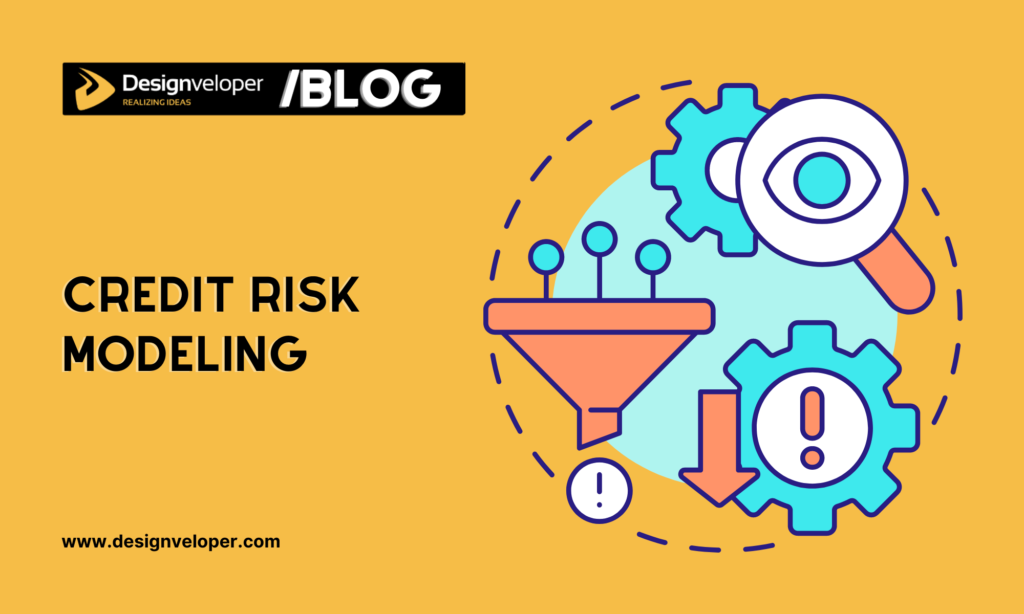
Modeling credit risks means using techniques and tools to forecast the possibility of a borrower defaulting on a loan or failing to fulfill their financial obligations. Businesses, especially financial institutions, will analyze lots of data (e.g., a borrower’s income, debt levels, and credit history) to make smarter lending decisions. This helps them minimize potential losses and monitor overall risk exposure.
With advanced analytical techniques and predictive capabilities, machine learning has transformed credit risk modeling in many ways. First, its algorithms can analyze a wide range of data to detect high-risk borrowers and mark possible default incidents before they arise. Second, they can automatically assess a customer or an entity’s creditworthiness to improve credit scoring and speed up the credit approval process.
Finally, machine learning can divide customers into different groups based on their risk profiles and credit behaviors. It even can dynamically modify interest rates or credit limits based on real-time data about a borrower’s credit risk and market conditions. This allows financial institutions to personalize offerings, loan terms, and communication strategies to meet customer needs and make them satisfied.
2. Detection of Credit Card Fraud & Money Laundering
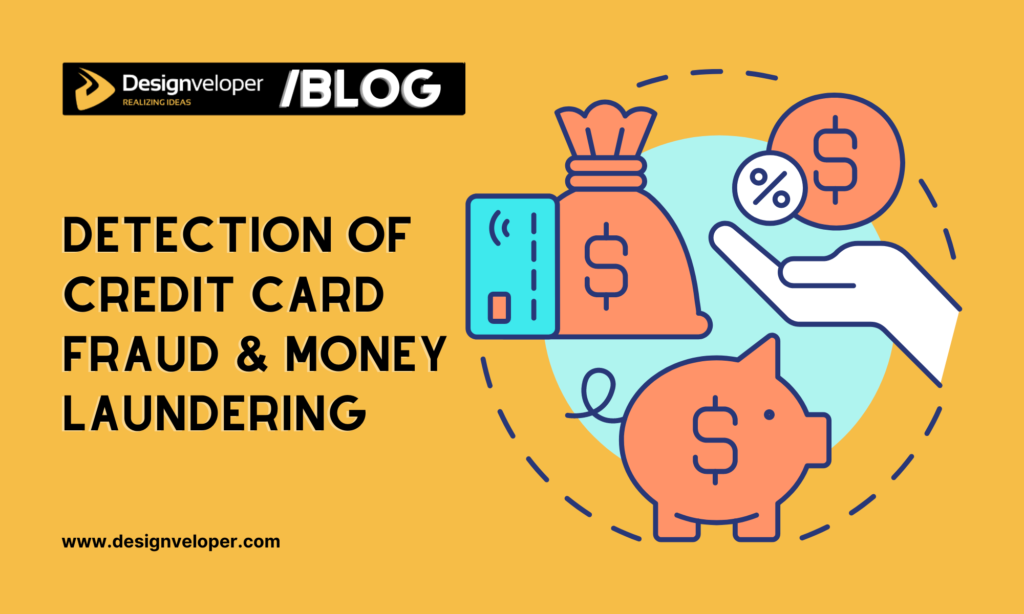
In 2024, $1.1 trillion of money laundering was reported across the Americas, with $102.6 billion attributed to payment fraud and $13.6 billion contributed to credit card fraud. When fraud has become increasingly complex, identifying it in real-time is crucial.
Today, various financial institutions have equipped their credit card payment infrastructure with ML-based monitoring systems (also called “workflow engines”). By scanning vast volumes of historical payment data, these systems can identify and block possibly unusual transactions in real-time.
Machine learning also helps impede money laundering activity significantly. It has the ability to spot complex patterns in the data and combine transaction information from different sources rapidly. This gives organizations a comprehensive picture of a customer’s activity, detects the likelihood of committing fraud, and greatly reduces false positives.
However, money laundering is hard to identify. As there’s no globally accepted definition of money laundering, financial institutions don’t often get feedback from law enforcers on which cases are actually considered money laundering. This means they hardly train machine learning models on these cases to spot money laundering activity.
Therefore, many institutions will optimize their ML risk management models by depending on lower-level suspicious activity reports. These reports split alerts as false alarms (not indicating actual money laundering) or legitimate cases (appearing as potential fraud).
Further, institutions leverage unsupervised learning to spot potential risks without pre-existing labels. They also rely on clustering to group similar data points and detect outliers, hence looking for anomalies in the data.
3. Surveillance of Conduct Behaviors
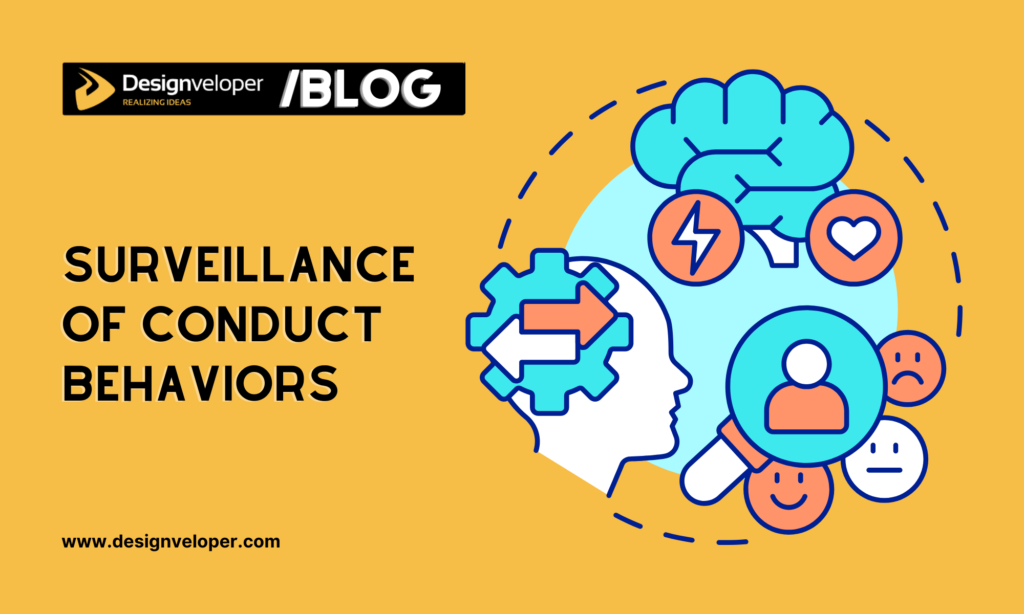
The 2016 scandal of Wells Fargo has raised a big concern about internal fraud. As employee behavior is crucial to keeping a business ethical and compliant, companies now use machine learning to track and understand even complex patterns in employee actions.
For example, in financial institutions, machine learning will analyze a trader’s individual trades and entire trading portfolio. If ML detects any sign of misconduct like opening unauthorized cards or breaking company rules, it will alert management or compliance teams for further investigation. This helps prevent the problem from becoming worse.
So, how can machine learning help with surveilling employee behaviors? By learning from past activities that are considered “normal” behavior, machine learning can distinguish which actions deviate from these normal patterns and flag them as suspicious.
ML models can continuously learn from new information based on their past observations or actions and use that information to modify their future behavior over time. This process is often known as a “feedback loop”. For this reason, ML models can adapt to any evolving employee behavior and detect future actions more accurately.
4. Cybersecurity
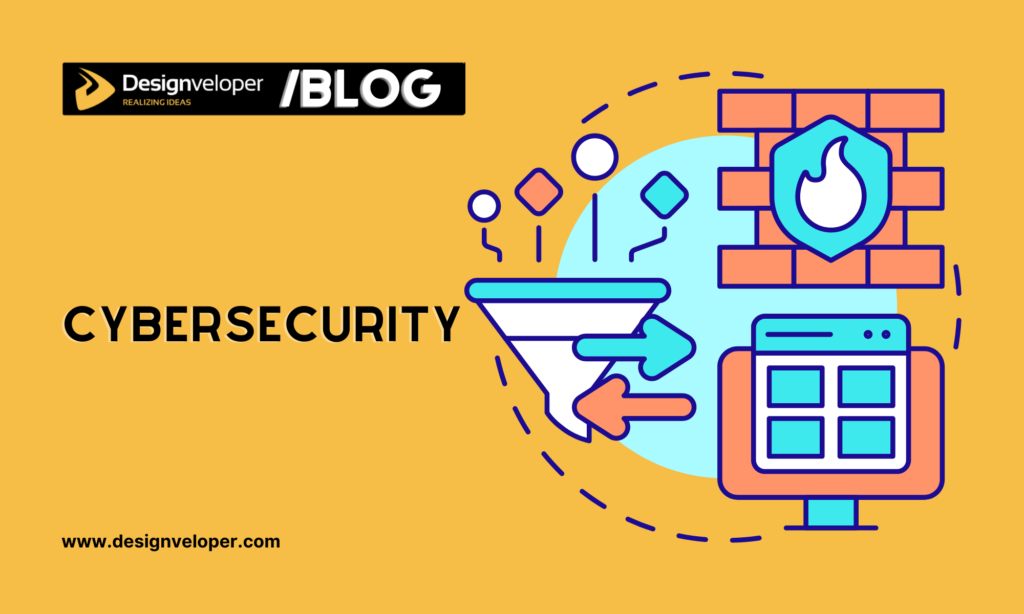
Machine learning plays a key role in analyzing and managing cybercrime, especially in the era when threats keep evolving. Its algorithms are trained to detect “normal” network activity. So when they see any suspicious signs – like an unexpected login from a new location or unusual links within an email, they’ll alert cybersecurity teams.
ML can analyze threat data from different sources (e.g., phishing campaigns or malware databases) to identify already known attack patterns and predict similar threats in the future. Some ML models can automatically respond to threats by separating affected systems, limiting access, or blocking suspicious IP addresses. ML also wins over traditional methods in learning from historical events and constantly adapts to new, evolving threats.
Drax – a leading power infrastructure provider of around 7% of the UK’s power – uses machine learning to reinforce its defense systems. The company previously confronted a wide range of challenges, like a rapidly evolving threat landscape, large amounts of data insufficiently analyzed, false positives, and insider threats. Therefore, Drax chose an ML-based cybersecurity platform called Enterprise Immune System to identify even unseen threats. This platform has self-learning capabilities to detect anomalies in real-time without the need for prior knowledge.
Upon successfully implementing the Enterprise Immune System on its corporate network, Drax rolled it out to its crucial industrial control system (ICS). The platform quickly alerts the company about potential intrusions that may bypass other security tools. This helps Drax continuously track and analyze the overall health of its system.
5. Operations
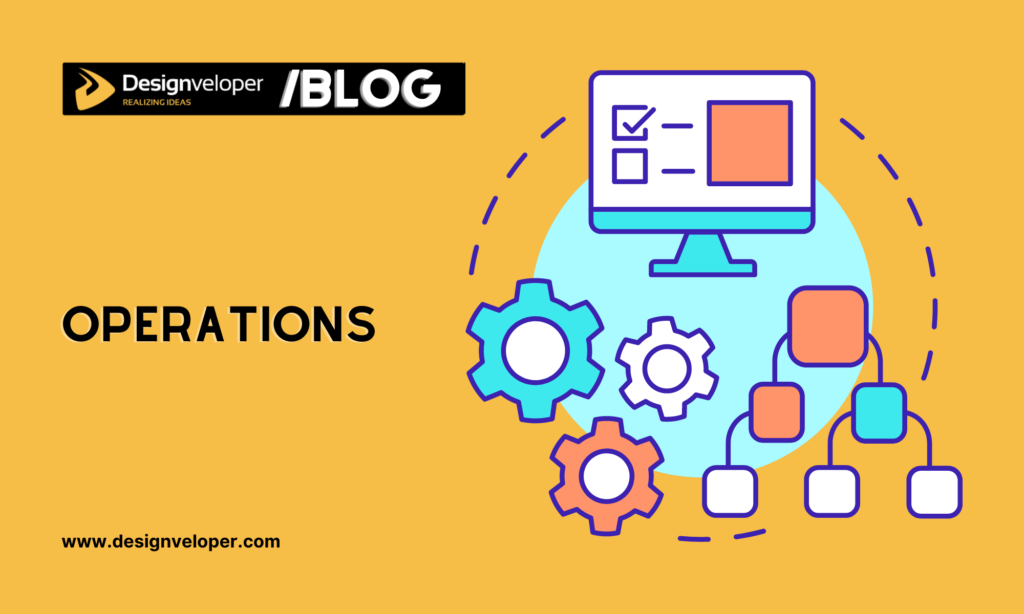
Machine learning helps with operational risk management by automatically identifying, assessing, and reducing risks related to business processes and human activities. Particularly, ML algorithms can analyze sensor data (e.g., vibration or temperature) from machinery to predict failures they they happen. This mitigates downtime and maintenance costs accordingly.
ML also helps prevent supply chain risks by analyzing data from suppliers, market trends, and geopolitical events to forecast possible disruptions. This allows companies to proactively modify their operations, avoiding production delays or stock shortages. ML models can recognize deviations from “normal” business processes to discover issues early and deter operational inefficiencies.
UPS (United Parcel Service) is a successful example of adopting machine learning to optimize logistics and reduce operational risks. In particular, the company developed a proprietary platform called ORION (On-Road Integrated Optimization and Navigation) to optimize delivery routes by constantly learning from traffic data, driver behavior, and weather conditions. Through ORION, UPS has saved about 100 million miles and 10 million gallons of fuel every year. It also helps minimize delays, reduce fuel consumption, and even prevent unanticipated accidents.
Machine Learning: A Long Road Ahead
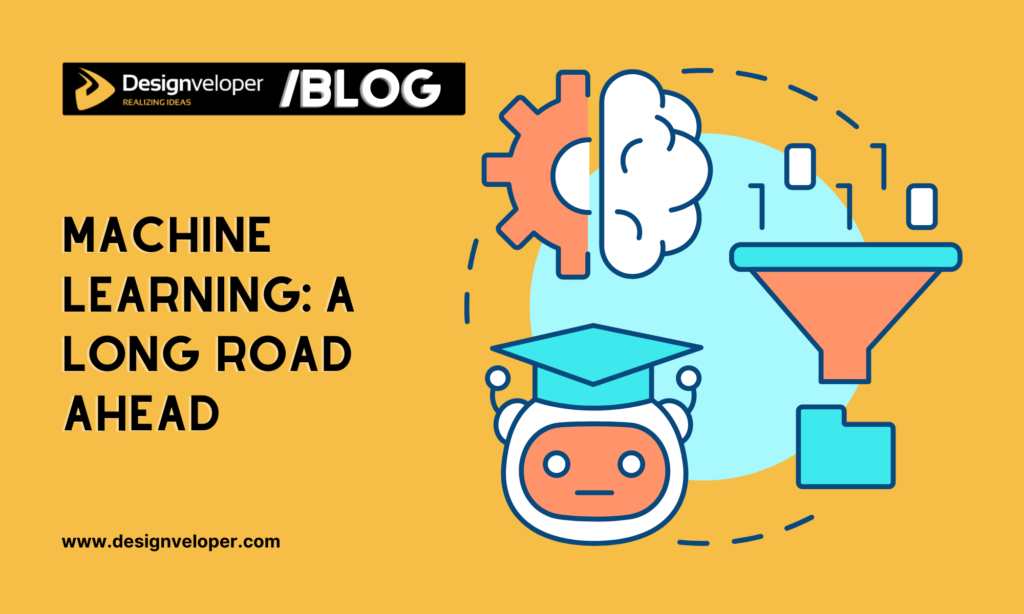
We can’t deny the huge potential and benefits of machine learning in risk management. However, it’s a long way to go if machine learning wants to achieve the intelligence level of human brains. It’s because machine learning itself still presents several challenges that prevent some business activities from performing well:
Lack of Explanatory Power
ML models, especially complex ones like neural networks, are often considered “black boxes.” This means they can make accurate predictions, but hardly interpret why they make a particular prediction or how they arrived at certain decisions. Therefore, they’re criticized for focusing on historical data to find patterns or correlations without needing to understand the root causes.
If you don’t understand the underlying reasons behind a correlation, the relationship can stop being true in the future due to condition changes. As a result, you’ll have no way of knowing when or why that might happen.
Imagine a company finding the sales of ice cream and sunglasses are highly associated. Whenever ice cream sales go up, so do sunglasses sales. If they don’t understand that both are correlated due to sunny weather, they can mistakenly think ice cream sales directly boost sunglasses sales and create marketing campaigns (e.g., buy an ice cream, give a 10% discount on sunglasses). These campaigns may be no longer effective when that correlation stops – perhaps because of unusual events like a pandemic changing customer behavior.
Therefore, using machine learning for risk management needs the involvement of human experts to explain further the correlation of variables.
Data Issues
Companies face problems with data quality, privacy, and availability. If the dataset has incomplete or missing information, ML models may not have a detailed view of the factors affecting risk. This can lead to poor predictions or biased outputs. This also proves true when the data contains errors, noise (irrelevant information), or outdated information.
In some risk management scenarios (like loan defaults or fraud detection), the dataset may face imbalance issues. For example, if the number of fraud cases is much smaller than that of legitimate transactions, ML models have difficulty detecting rate but crucial risk incidents. As a result, they can be in favor of forecasting more common, less risky events. Not to mention that ML can struggle with data from various sources (e.g., internal systems or external reports) if these sources are not standardized or consistent.
To deal with these data issues, companies may apply techniques like data standardization, oversampling (like SMOTE), or feature engineering. They may adopt an MLOps approach to continuously monitor, retrain, and fine-tune ML models to ensure they always perform as expected.
Final Thoughts
In this era, using machine learning for risk management is not something new. This cutting-edge technology has a wide range of applications in assessing your business risks, from credit fraud and money laundering to cybersecurity and operations. Despite some existing challenges, machine learning still brings immense benefits to your business if it’s properly used. Further, combining human expertise and other techniques will buffer the negative impacts of ML models. For more interesting news about this topic, subscribe to our blog!
In case you want to build a customized, scalable ML model, yet struggle with expertise related to this advanced tech, contact Designveloper and discuss your idea!
Our team has extensive expertise and experience in crafting cutting-edge AI solutions tailored to your specific business needs.
Leveraging the latest AI advancements and advanced algorithms, we’re committed to creating dynamic and innovative models that seamlessly integrate your existing infrastructure and offer robust security practices. These solutions boost your operational efficiency, enhance customer experience, and propel your business growth.
So why wait? Let’s talk!
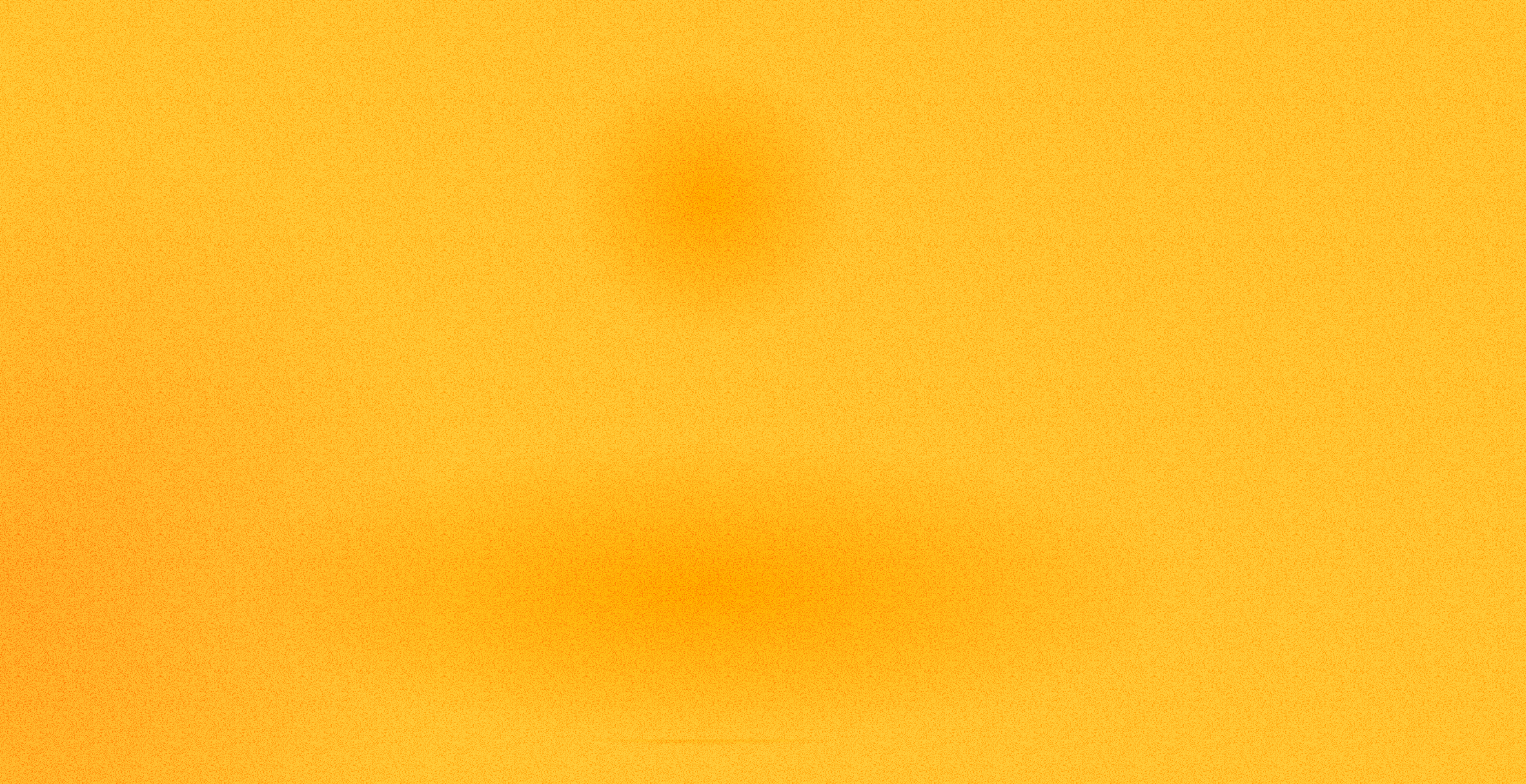
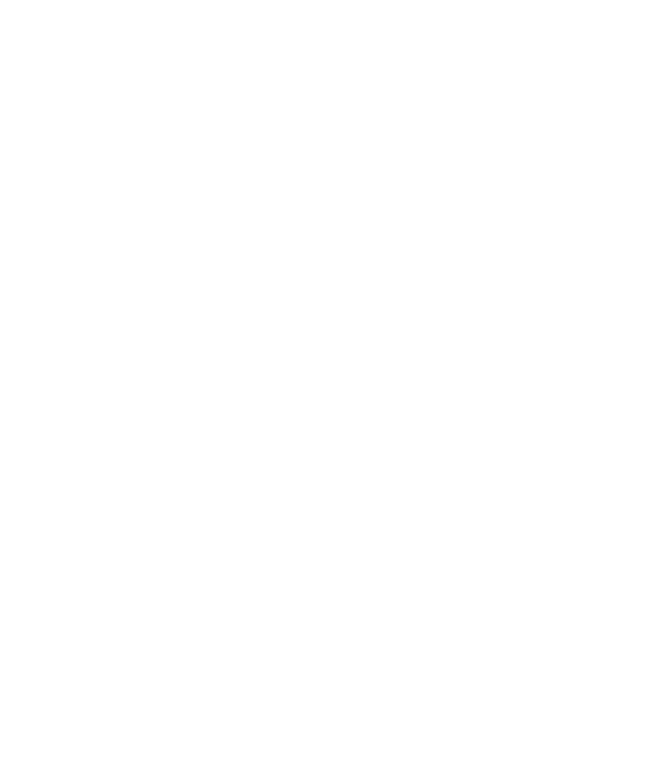
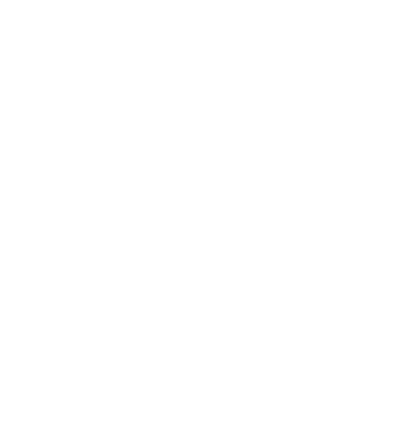
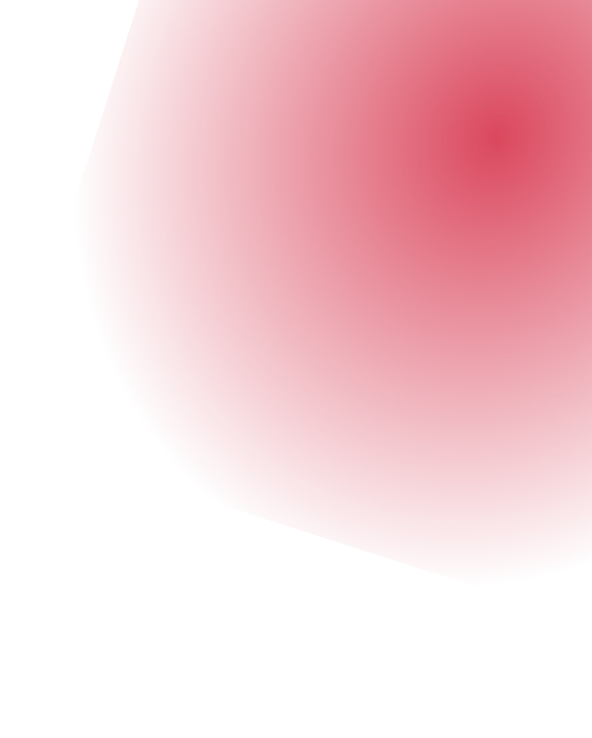
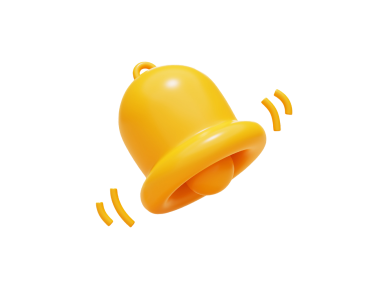
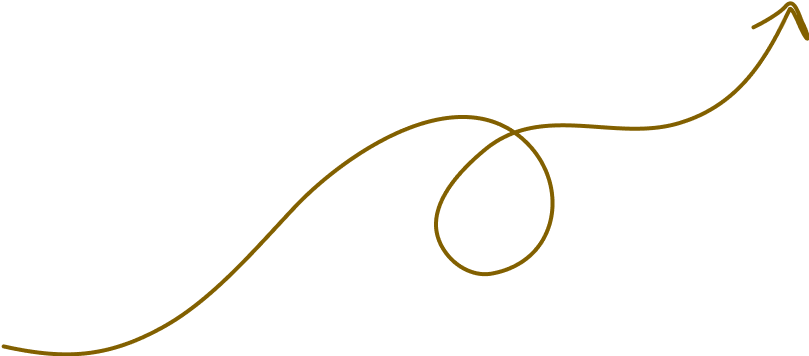